Combining multimodal magnetic resonance brain imaging and machine learning to unravel neurocognitive function in non-neuropsychiatric systemic lupus erythematosus
RHEUMATOLOGY(2024)
摘要
Objective To study whether multimodal brain MRI comprising permeability and perfusion measures coupled with machine learning can predict neurocognitive function in young patients with SLE without neuropsychiatric manifestations. Methods SLE patients and healthy controls (HCs) (<= 40 years of age) underwent multimodal structural brain MRI that comprised voxel-based morphometry (VBM), magnetization transfer ratio (MTR) and dynamic contrast-enhanced (DCE) MRI in this cross-sectional study. Neurocognitive function assessed by Automated Neuropsychological Assessment Metrics was reported as the total throughput score (TTS). Olfactory function was assessed. A machine learning-based model (i.e. glmnet) was constructed to predict TTS. Results Thirty SLE patients and 10 HCs were studied. Both groups had comparable VBM, MTR, olfactory bulb volume (OBV), olfactory function and TTS. While after correction for multiple comparisons the uncorrected increase in the blood-brain barrier (BBB) permeability parameters compared with HCs did not remain evident in SLE patients, DCE-MRI perfusion parameters, notably an increase in right amygdala perfusion, was positively correlated with TTS in SLE patients (r = 0.636, false discovery rate P < 0.05). A machine learning-trained multimodal MRI model comprising alterations of VBM, MTR, OBV and DCE-MRI parameters mainly in the limbic system regions predicted TTS in SLE patients (r = 0.644, P < 0.0005). Conclusion Multimodal brain MRI demonstrated increased right amygdala perfusion that was associated with better neurocognitive performance in young SLE patients without statistically significant BBB leakage and microstructural abnormalities. A machine learning-constructed multimodal model comprising microstructural, perfusion and permeability parameters accurately predicted neurocognitive performance in SLE patients.
更多查看译文
关键词
SLE,lupus,cognitive dysfunction,blood-brain barrier,machine learning,limbic
AI 理解论文
溯源树
样例
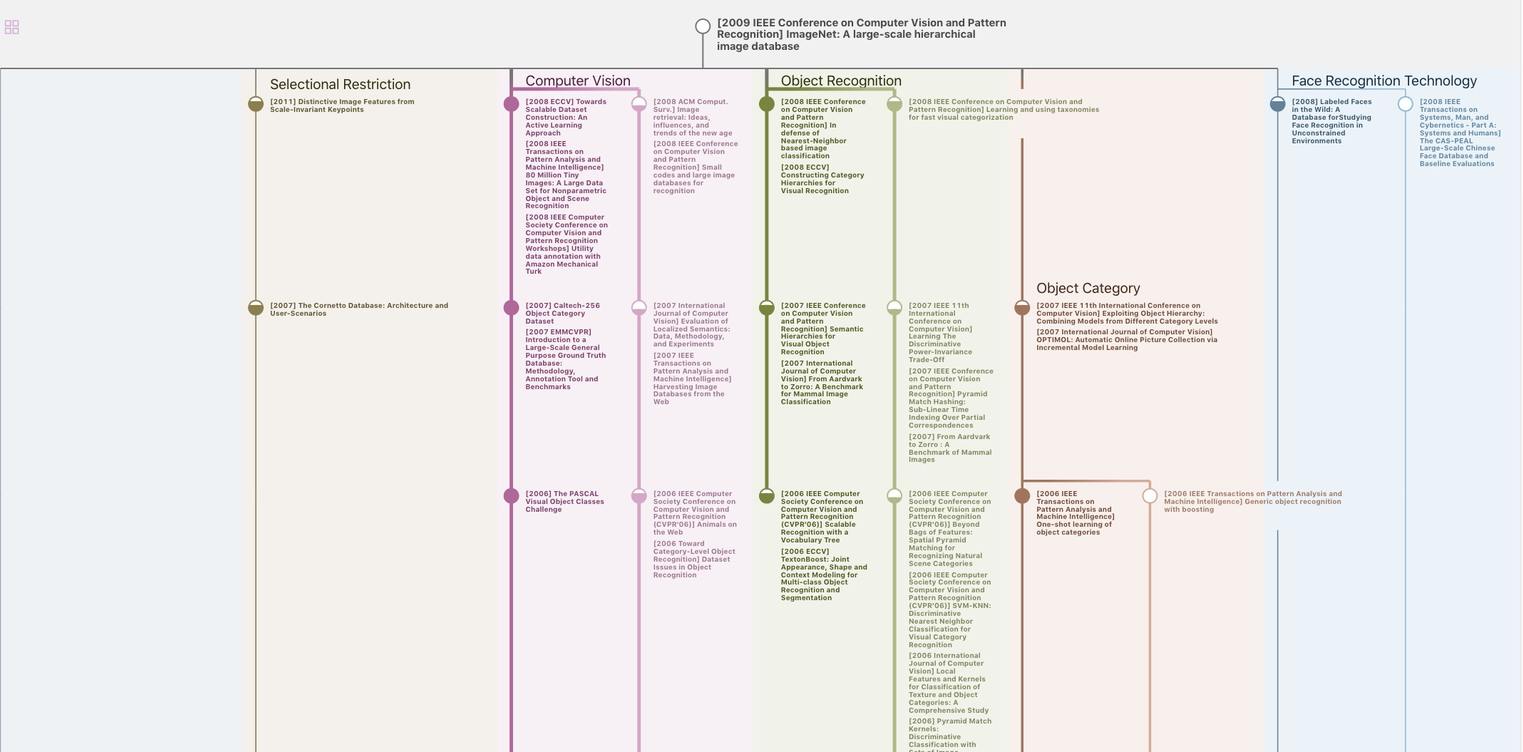
生成溯源树,研究论文发展脉络
Chat Paper
正在生成论文摘要