Statistical Learning for Nonlinear Dynamical Systems with Applications to Aircraft-UAV Collisions
TECHNOMETRICS(2023)
摘要
This article investigates a physics-informed statistical approach capable of (i) learning nonlinear system dynamics by using data generated from a nonlinear system as well as the underlying governing physics, and (ii) predicting system dynamics with reasonable accuracy and a computational speed much faster than numerical methods. The proposed approach obtains the reduced-order model from the full-order governing equations. A function-to-function regression, based on multivariate Functional Principal Component Analysis, establishes the mapping between external forcing and system dynamics, while a multivariate Gaussian Process is used to capture the relationship between parameters and external forcing. In the application, the proposed approach is applied to predict aircraft nose skin deformation after Unmanned Aerial Vehicle (UAV) collisions at different impact attitudes (i.e., pitch, yaw and roll degrees). We show that the proposed physics-informed statistical model can achieve a 12% out-of-sample mean relative error, and is more than 10(3) times faster than Finite Element Analysis (FEA). Computer code and sample data are available on GitHub.
更多查看译文
关键词
Aviation safety, function-to-function regression, functional principal component analysis, Gaussian process, Nonlinear structural dynamics, Physics-informed statistical learning, Reduced-order models
AI 理解论文
溯源树
样例
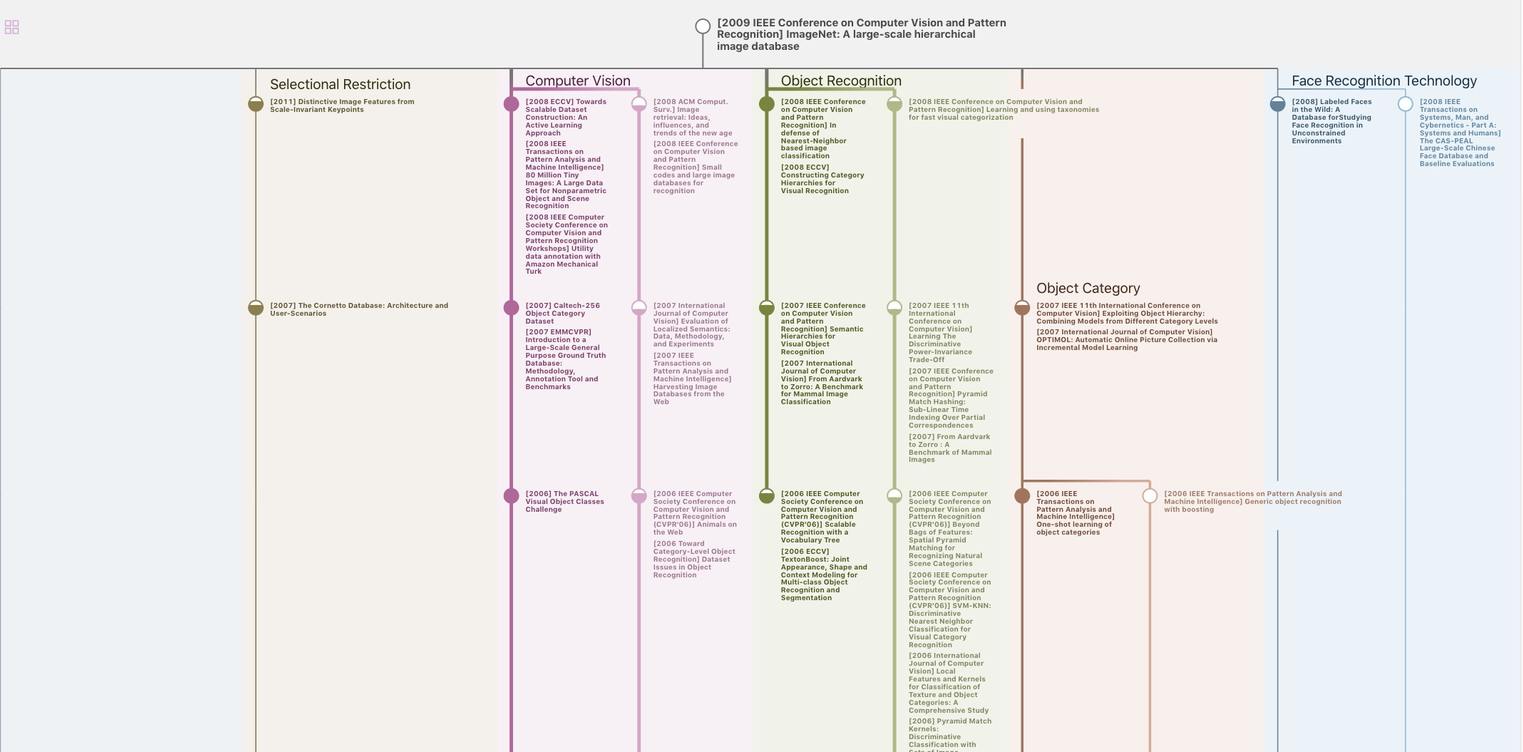
生成溯源树,研究论文发展脉络
Chat Paper
正在生成论文摘要