Feature-Driven Closed-Loop Optimization for Battery Fast Charging Design with Machine Learning
JOURNAL OF THE ELECTROCHEMICAL SOCIETY(2023)
摘要
Electric vehicle batteries must possess fast rechargeability. However, fast charging of lithium-ion batteries remains a great challenge. This paper develops a feature-driven closed-loop optimization (CLO) methodology to efficiently design health-conscious fast-charging strategies for batteries. To avoid building an early outcome predictor, the feature highly related to battery end-of-life is used as the optimization objective instead of using the predicted lifetime. This feature is extracted from the battery's early cycles and the experimental cost is thus reduced. By developing closed-loop multi-channel experiments with Bayesian optimization (BO), the optimal charging protocols with long cycle lives are located quickly and efficiently among 224 four-step, 10 min fast-charging protocols. Experimental results show that BO performs well with different acquisition functions, and a minimum of 12 paralleled channels for each round of experiments are recommended to obtain stable optimization results. Compared with the benchmark, the developed method recommends similar fast-charging protocols with long cycle lives based on much less experimental cost.
更多查看译文
关键词
battery fast charging design,optimization,machine learning,feature-driven,closed-loop
AI 理解论文
溯源树
样例
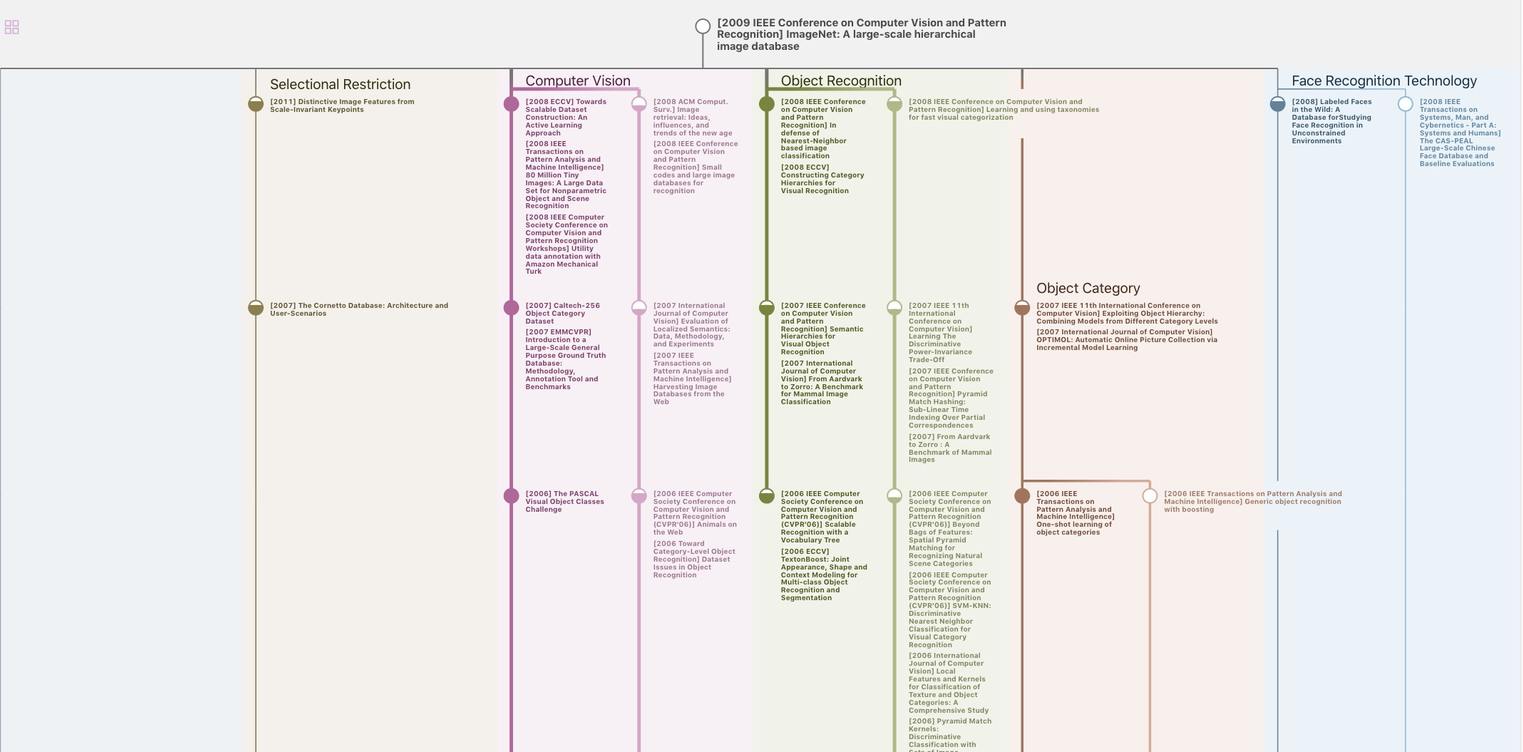
生成溯源树,研究论文发展脉络
Chat Paper
正在生成论文摘要