Comparing estimation approaches for generalized additive mixed models with binary outcomes
JOURNAL OF STATISTICAL COMPUTATION AND SIMULATION(2023)
摘要
Generalized additive mixed models (GAMMs) extend generalized linear mixed models (GLMMs) to allow the covariates to be nonparametrically associated with the response. Estimation of such models for correlated binary data is challenging and estimation techniques often yield contrasting results. Via simulations, we compared the performance of the Bayesian and likelihood-based methods for estimating the components of GAMMs under a wide range of conditions. For the Bayesian method, we also assessed the sensitivity of the results to the choice of prior distributions of the variance components. In addition, we investigated the effect of multicollinearity among covariates on the estimation of the model components. We then applied the methods to the Bangladesh Demographic Health Survey data to identify the factors associated with the malnutrition of children in Bangladesh. While no method uniformly performed best in estimating all components of the model, the Bayesian method using half-Cauchy priors for variance components generally performed better, especially for small cluster size. The overall curve fitting performance was sensitive to the prior selection for the Bayesian methods and also to the extent of multicollinearity.
更多查看译文
关键词
Penalized splines, generalized linear mixed models, penalized quasi-likelihood, Laplace approximation, Markov chain Monte Carlo, variance components
AI 理解论文
溯源树
样例
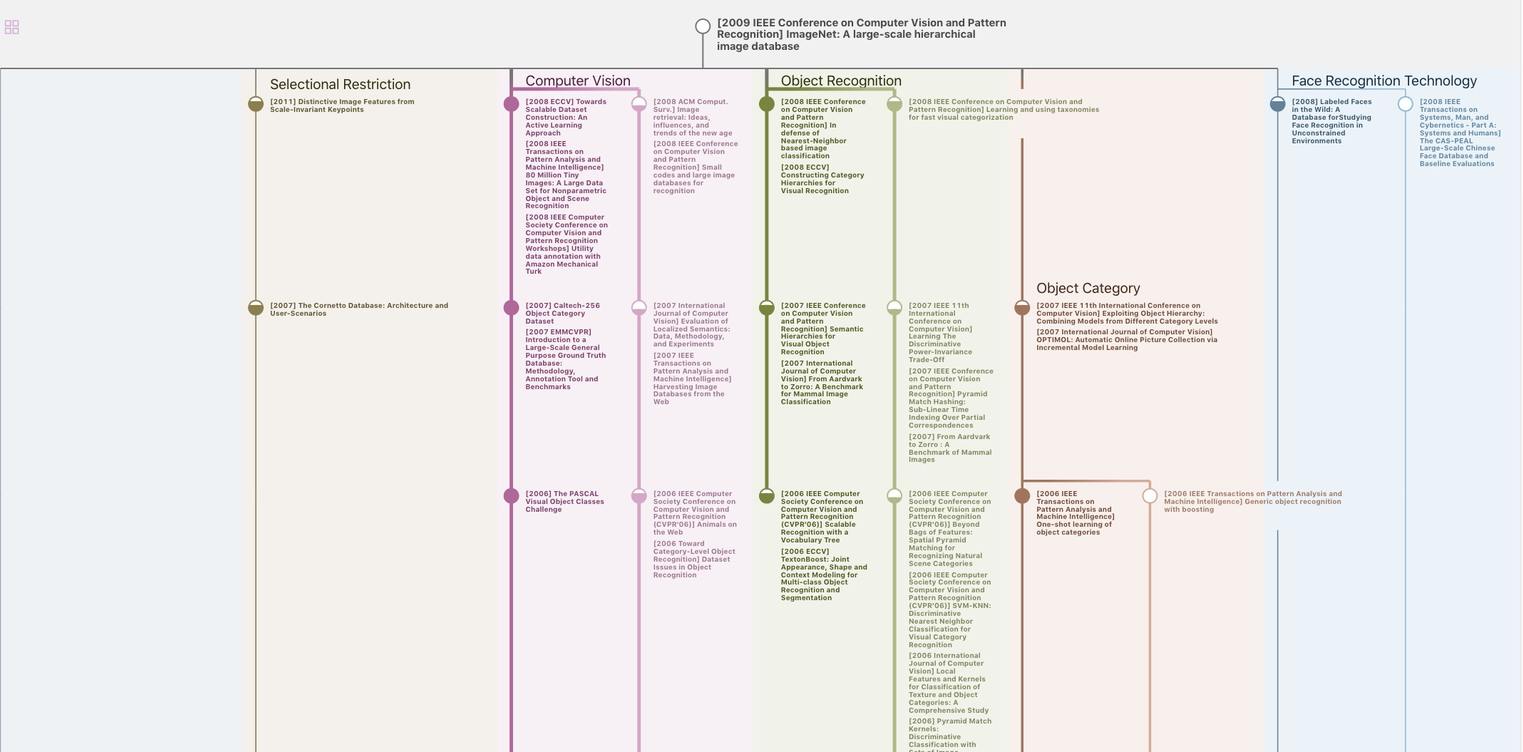
生成溯源树,研究论文发展脉络
Chat Paper
正在生成论文摘要