Deep learning for few-shot white blood cell image classification and feature learning
COMPUTER METHODS IN BIOMECHANICS AND BIOMEDICAL ENGINEERING-IMAGING AND VISUALIZATION(2023)
摘要
Differential counting of white blood cells (WBCs) in bone marrow using artificial intelligence (AI)-based models, such as convolutional neural network (CNN) and its various variants, can help physicians to efficiently diagnose many critical diseases such as leukaemia, AIDS and cancers. In this work, we implement a deep transfer learning to several CNN models to examine their effectiveness on automatically classifying WBCs into lymphocytes and non-lymphocytes groups. Our results show that transfer learning can enhance the training of the model and improve the model performance. We also discover that using image masking to remove irrelevant image pixels can further increase the accuracy of the model predictions. Moreover, we assess the impact of three data augmentation techniques to address the imbalance in the data set, which commonly occurs in many biological applications. Our results show that all the three examined data augmentation methods improve the classification results on both training and testing data sets. Altogether, we demonstrate that deep neural networks, when combined with transfer learning and imaging processing techniques, can serve as a powerful tool to conduct automatic differential counting of WBCs, and thus facilitate the diagnosis of the WBC-related disorders, monitor the disease progression and improve the effectiveness of therapeutics.
更多查看译文
关键词
deep learning,blood,feature learning,cell,few-shot
AI 理解论文
溯源树
样例
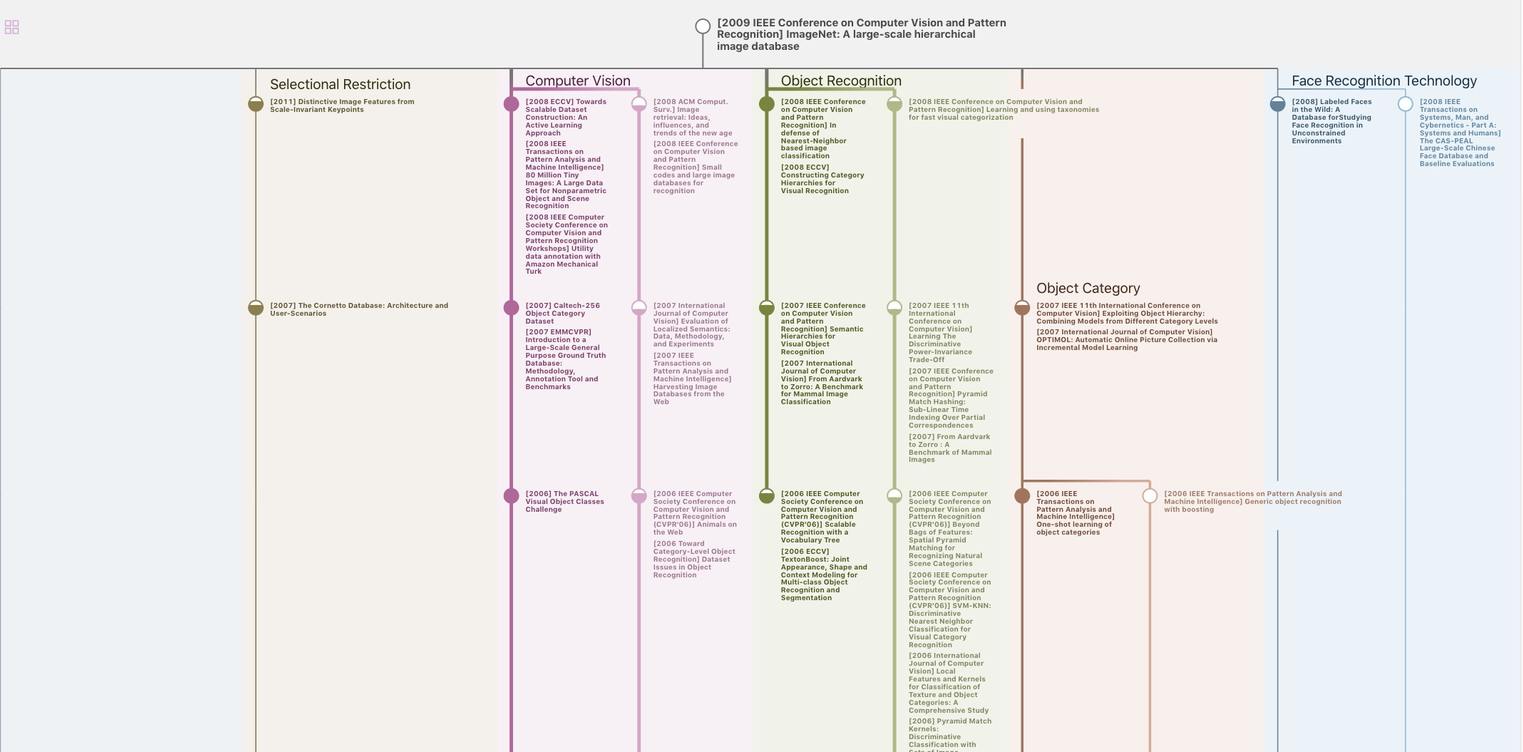
生成溯源树,研究论文发展脉络
Chat Paper
正在生成论文摘要