Reinforcement Learning-Based Model-Free Controller for Feedback Stabilization of Robotic Systems.
IEEE transactions on neural networks and learning systems(2022)
摘要
This article presents a reinforcement learning (RL) algorithm for achieving model-free control of robotic applications. The RL functions are adapted with the least-square temporal difference (LSTD) learning algorithms to develop a model-free state feedback controller by establishing linear quadratic regulator (LQR) as a baseline controller. The classical least-square policy iteration technique is adapted to establish the boundary conditions for complexities incurred by the learning algorithm. Furthermore, the use of exact and approximate policy iterations estimates the parameters of the learning functions for a feedback policy. To assess the operation of the proposed controller, the trajectory tracking and balancing control problems of unmanned helicopters and balancer robotic applications are solved for real-time experiment. The results showed the robustness of the proposed approach in achieving trajectory tracking and balancing control.
更多查看译文
关键词
Costs,Complexity theory,Robots,Aerospace electronics,Trajectory,Q-learning,Process control,Discrete algebraic Riccati equation,dynamic Lyapunov equation,least-square policy iteration,linear quadratic regulator (LQR),reinforcement learning (RL)
AI 理解论文
溯源树
样例
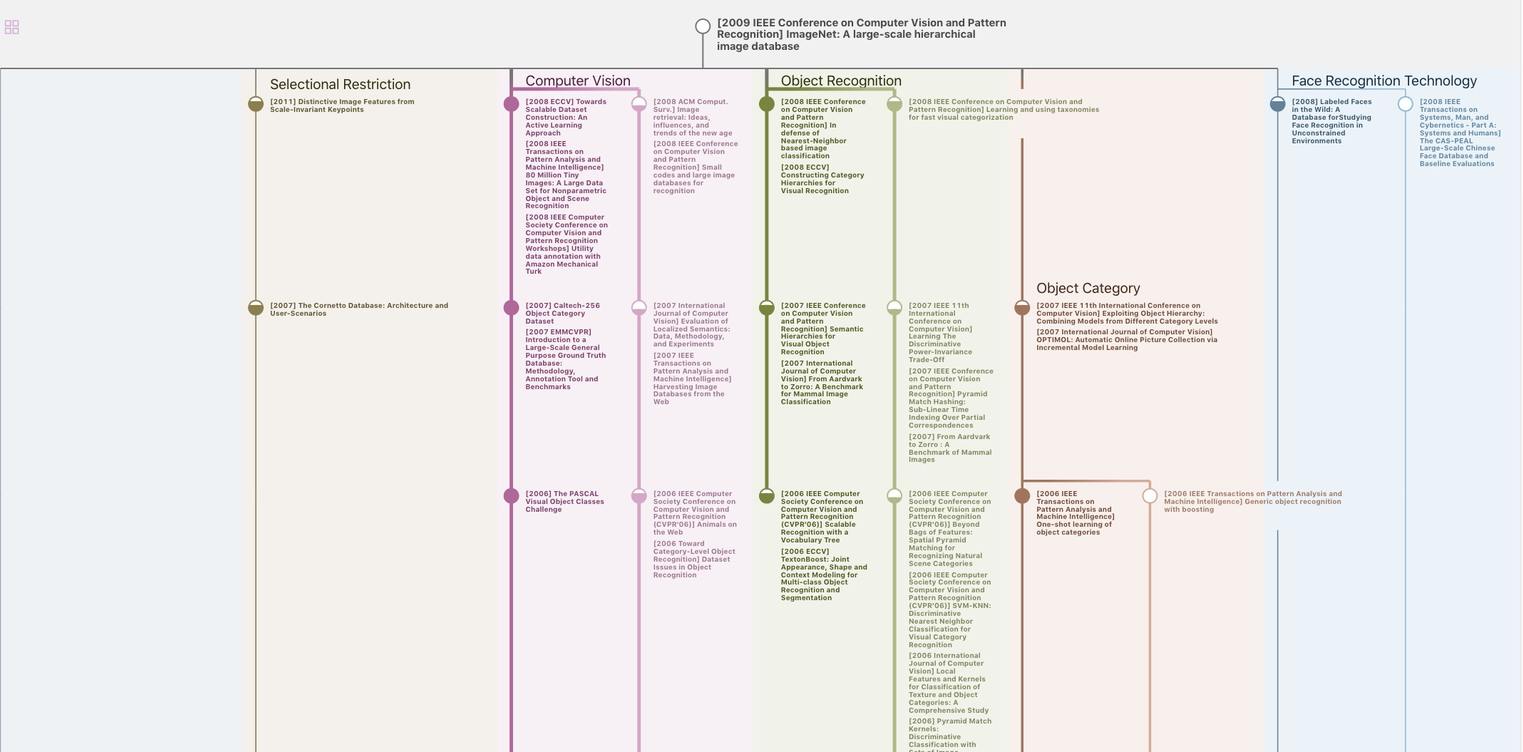
生成溯源树,研究论文发展脉络
Chat Paper
正在生成论文摘要