Explore deep network for a class of fractional partial differential equations
Chaos, Solitons & Fractals(2023)
摘要
In this paper, we present a novel approach for solving a class of fractional partial differential equations (FPDEs) and their inverse problems using deep neural networks (DNNs). Our proposed framework utilizes the discrete Caputo fractional derivative method to approximate fractional partial derivatives, while leveraging automatic differentiation of neural networks to obtain integer derivatives. This approach offers several advantages, including avoiding the direct solution of the original FPDEs and overcoming the limitations faced by traditional numerical methods in handling FPDEs. To validate our approach, we provide numerical examples with known analytical solutions, accompanied by graphical and numerical results. Our findings demonstrate that the proposed method is easily implementable, exhibits fast convergence, robustness, and effectiveness in solving multidimensional FPDEs and their inverse problems.
更多查看译文
关键词
Deep neural networks,Fractional partial differential equations,Inverse problems,Numerical simulation,Discrete Caputo,Convergence
AI 理解论文
溯源树
样例
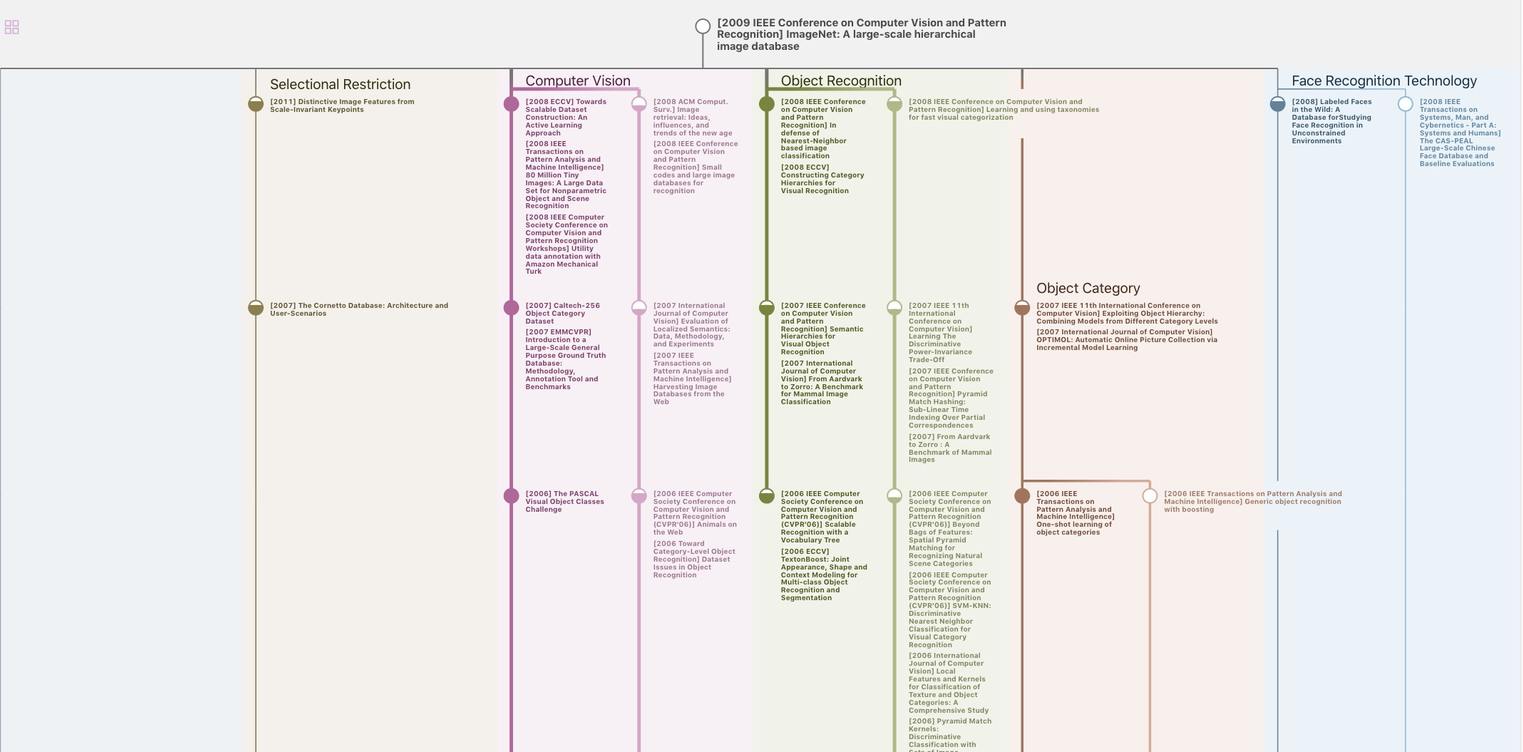
生成溯源树,研究论文发展脉络
Chat Paper
正在生成论文摘要