Incremental Retraining, Clinical Implementation, and Acceptance Rate of Deep Learning Auto-Segmentation for Male Pelvis in a Multiuser Environment.
Medical physics on CD-ROM/Medical physics(2023)
摘要
BackgroundDeep learning auto-segmentation (DLAS) models have been adopted in the clinic; however, they suffer from performance deterioration owing to the clinical practice variability. Some commercial DLAS software provide an incremental retraining function that enables users to train a custom model using their institutional data to account for clinical practice variability. PurposeThis study was performed to evaluate and implement the commercial DLAS software with the incremental retraining function for definitive treatment of patients with prostate cancer in a multi-user environment. MethodsCT-based target organs and organs-at-risk (OAR) delineation of 215 prostate cancer patients were utilized. The performance of three commercial DLAS software built-in models was validated with 20 patients. A retrained custom model was developed using 100 patients and evaluated on the remaining data (n = 115). Dice similarity coefficient (DSC), Hausdorff distance (HD), mean surface distance (MSD), and surface DSC (SDSC) were utilized for quantitative evaluation. A multi-rater qualitative evaluation was blindly performed with a five-level scale. Visual inspection was performed in consensus and non-consensus unacceptable cases to identify the failure modes. ResultsThree commercial DLAS vendor built-in models achieved sub-optimal performance in 20 patients. The retrained custom model had a mean DSC of 0.82 for prostate, 0.48 for seminal vesicles (SV), and 0.92 for rectum, respectively. This represents a significant improvement over the built-in model with DSC of 0.73, 0.37, and 0.81 for the corresponding structures. Compared to the acceptance rate of 96.5% and consensus unacceptable rate (i.e., both reviewers rated as unacceptable) of 3.5% achieved by manual contours, the custom model achieved a 91.3% acceptance rate and 8.7% consensus unacceptable rate. The failure modes of retrained custom model were attributed to the following: cystogram (n = 2), hip prosthesis (n = 2), low dose rate brachytherapy seeds (n = 2), air in endorectal balloon(n = 1), non-iodinated spacer (n = 2), and giant bladder(n = 1). ConclusionThe commercial DLAS software with the incremental retraining function was validated and clinically adopted for prostate patients in a multi-user environment. AI-based auto-delineation of the prostate and OARs is shown to achieve improved physician acceptance, overall clinical utility, and accuracy.
更多查看译文
关键词
deep learning auto-segmentation,inter-observer contour variation,prostate radiotherapy
AI 理解论文
溯源树
样例
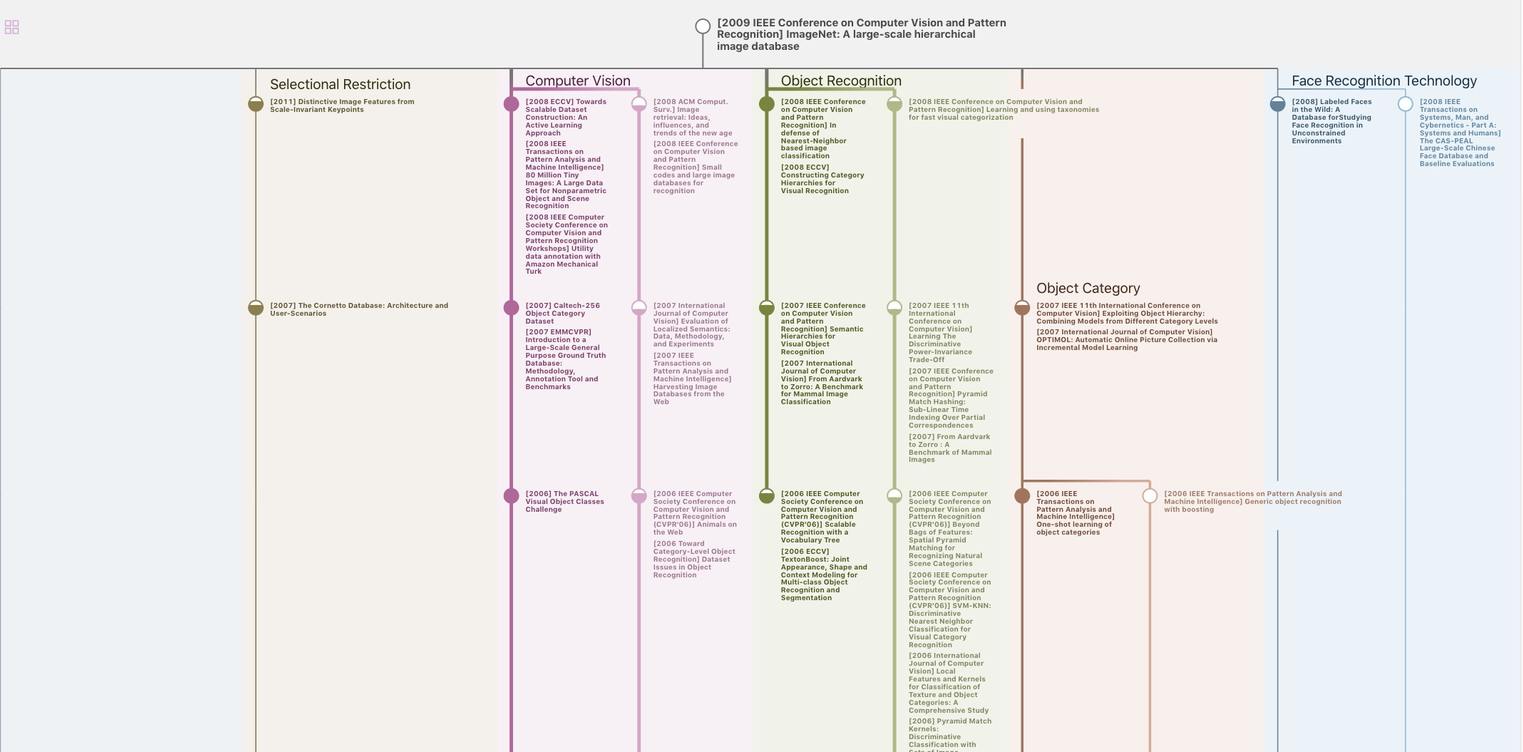
生成溯源树,研究论文发展脉络
Chat Paper
正在生成论文摘要