Technical note: Evaluation of missing data imputation methods for human osteometric measurements.
American journal of biological anthropology(2023)
摘要
It is not uncommon for biological anthropologists to analyze incomplete bioarcheological or forensic skeleton specimens. As many quantitative multivariate analyses cannot handle incomplete data, missing data imputation or estimation is a common preprocessing practice for such data. Using William W. Howells' Craniometric Data Set and the Goldman Osteometric Data Set, we evaluated the performance of multiple popular statistical methods for imputing missing metric measurements. Results indicated that multiple imputation methods outperformed single imputation methods, such as Bayesian principal component analysis (BPCA). Multiple imputation with Bayesian linear regression implemented in the R package norm2, the Expectation-Maximization (EM) with Bootstrapping algorithm implemented in Amelia, and the Predictive Mean Matching (PMM) method and several of the derivative linear regression models implemented in mice, perform well regarding accuracy, robustness, and speed. Based on the findings of this study, we suggest a practical procedure for choosing appropriate imputation methods.
更多查看译文
关键词
craniometrics,imputation,missing data,osteometrics
AI 理解论文
溯源树
样例
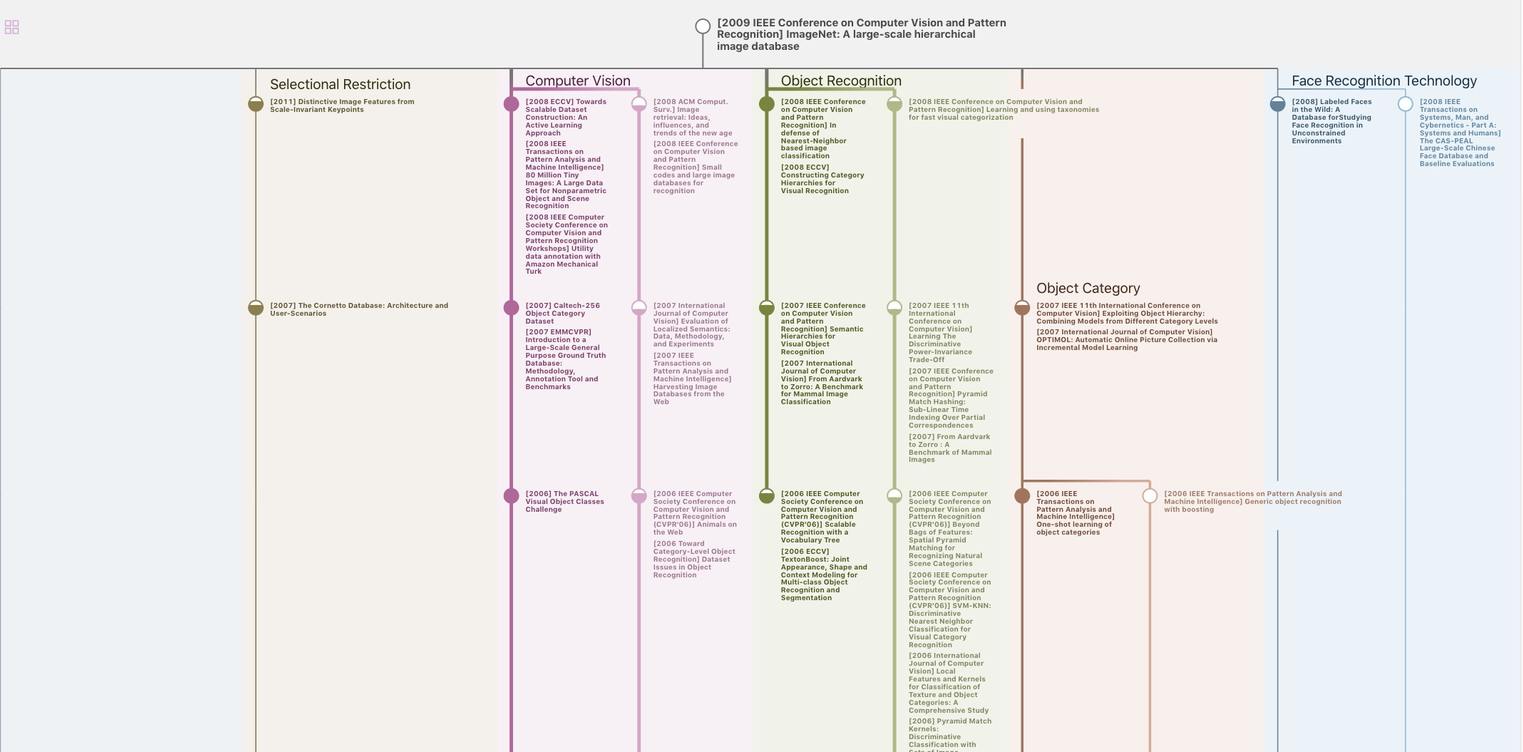
生成溯源树,研究论文发展脉络
Chat Paper
正在生成论文摘要