Investigating human prediction: sequence termination provides insights into predictive statistics
crossref(2021)
摘要
Prediction is a fundamental process in human cognition. Prediction means extracting one or more statistics from the distribution of past inputs and using that information to make a decision. What are the statistics underlying human predictions, and how do they change with training? To investigate these questions, we designed a sequence termination task, where participants watch temporally unfolding sequences and terminate them when they can predict the next item. We then test how well the participants’ termination points are predicted by computational models. We contrast frequency estimation models (How often did this symbol appear in the sequence?), transition models (How often did symbol A follow symbol B?), and a chunking model (What are the patterns of symbols?). In an online experiment with 65 adults, we find that participants are best fit by a transition-counting model. To assess the effect of training, we manipulated passive exposure to the sequences prior to the sequence termination task. Contrary to our expectations, prior exposure to sequences had no effect on termination performance– whether tested statistically or computationally, and despite good power. Lastly, training specifically on the termination task may shift responses towards chunking. These results provide insight into the representations, or information in mind, behind prediction. However, the lack of an effect of prior exposure makes it clear that sequence termination measures explicit, or conscious, prediction. Future work could examine whether representations in explicit prediction tasks like sequence termination are different from implicit, or unconscious, tasks like the serial reaction time task.
更多查看译文
AI 理解论文
溯源树
样例
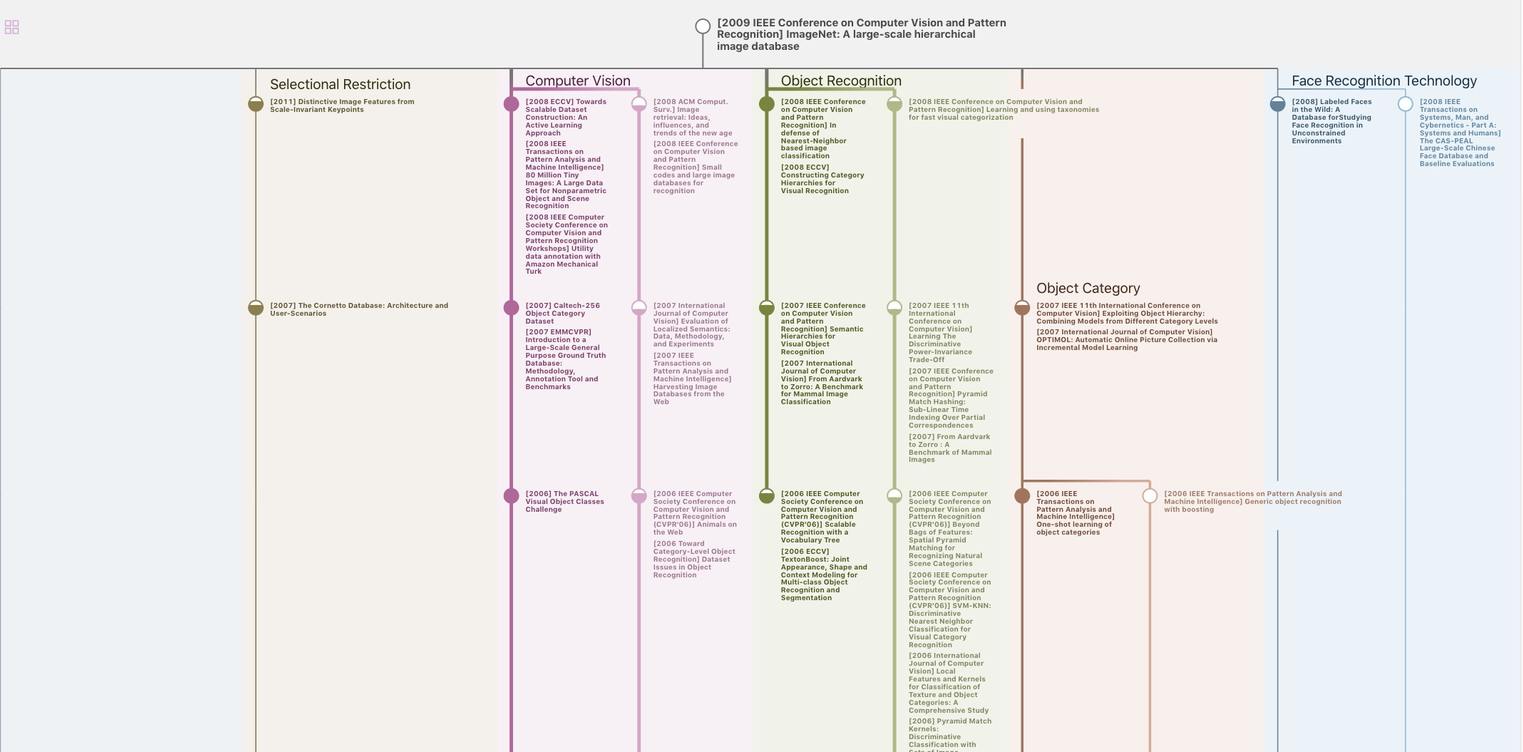
生成溯源树,研究论文发展脉络
Chat Paper
正在生成论文摘要