DECOR: Degree-Corrected Social Graph Refinement for Fake News Detection
PROCEEDINGS OF THE 29TH ACM SIGKDD CONFERENCE ON KNOWLEDGE DISCOVERY AND DATA MINING, KDD 2023(2023)
摘要
Recent efforts in fake news detection have witnessed a surge of interest in using graph neural networks (GNNs) to exploit rich social context. Existing studies generally leverage fixed graph structures, assuming that the graphs accurately represent the related social engagements. However, edge noise remains a critical challenge in real-world graphs, as training on suboptimal structures can severely limit the expressiveness of GNNs. Despite initial efforts in graph structure learning (GSL), prior works often leverage node features to update edge weights, resulting in heavy computational costs that hinder the methods' applicability to large-scale social graphs. In this work, we approach the fake news detection problem with a novel aspect of social graph refinement. We find that the degrees of news article nodes exhibit distinctive patterns, which are indicative of news veracity. Guided by this, we propose DECOR, a novel application of Degree-Corrected Stochastic Blockmodels to the fake news detection problem. Specifically, we encapsulate our empirical observations into a lightweight social graph refinement component that iteratively updates the edge weights via a learnable degree correction mask, which allows for joint optimization with a GNN-based detector. Extensive experiments on two real-world benchmarks validate the effectiveness and efficiency of DECOR.
更多查看译文
关键词
Fake News,Graph Neural Networks,Social Network
AI 理解论文
溯源树
样例
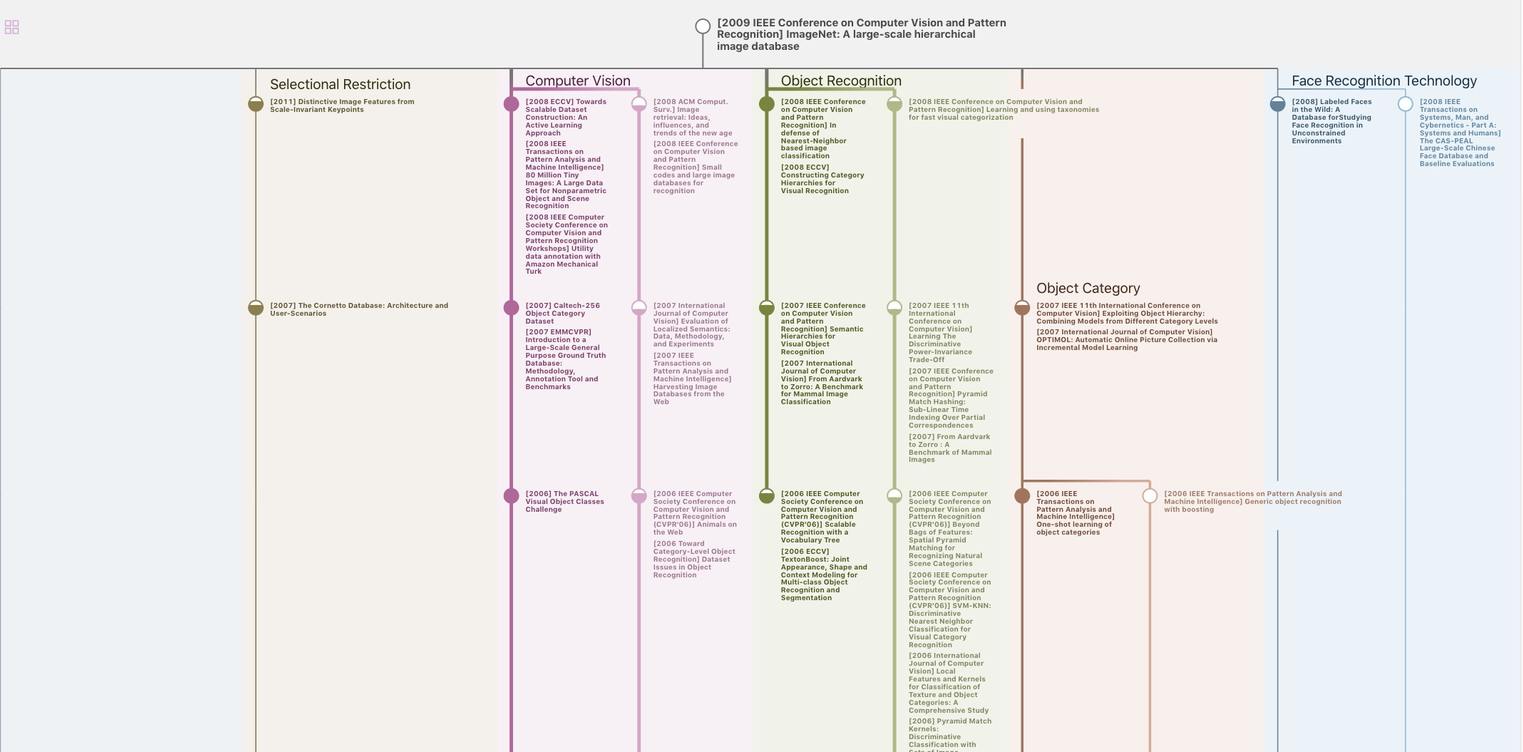
生成溯源树,研究论文发展脉络
Chat Paper
正在生成论文摘要