Bootstrapping the Cross-Validation Estimate
arXiv (Cornell University)(2023)
摘要
Cross-validation is a widely used technique for evaluating the performance of prediction models. It helps avoid the optimism bias in error estimates, which can be significant for models built using complex statistical learning algorithms. However, since the cross-validation estimate is a random value dependent on observed data, it is essential to accurately quantify the uncertainty associated with the estimate. This is especially important when comparing the performance of two models using cross-validation, as one must determine whether differences in error estimates are a result of chance fluctuations. Although various methods have been developed for making inferences on cross-validation estimates, they often have many limitations, such as stringent model assumptions This paper proposes a fast bootstrap method that quickly estimates the standard error of the cross-validation estimate and produces valid confidence intervals for a population parameter measuring average model performance. Our method overcomes the computational challenge inherent in bootstrapping the cross-validation estimate by estimating the variance component within a random effects model. It is just as flexible as the cross-validation procedure itself. To showcase the effectiveness of our approach, we employ comprehensive simulations and real data analysis across three diverse applications.
更多查看译文
关键词
estimate,cross-validation
AI 理解论文
溯源树
样例
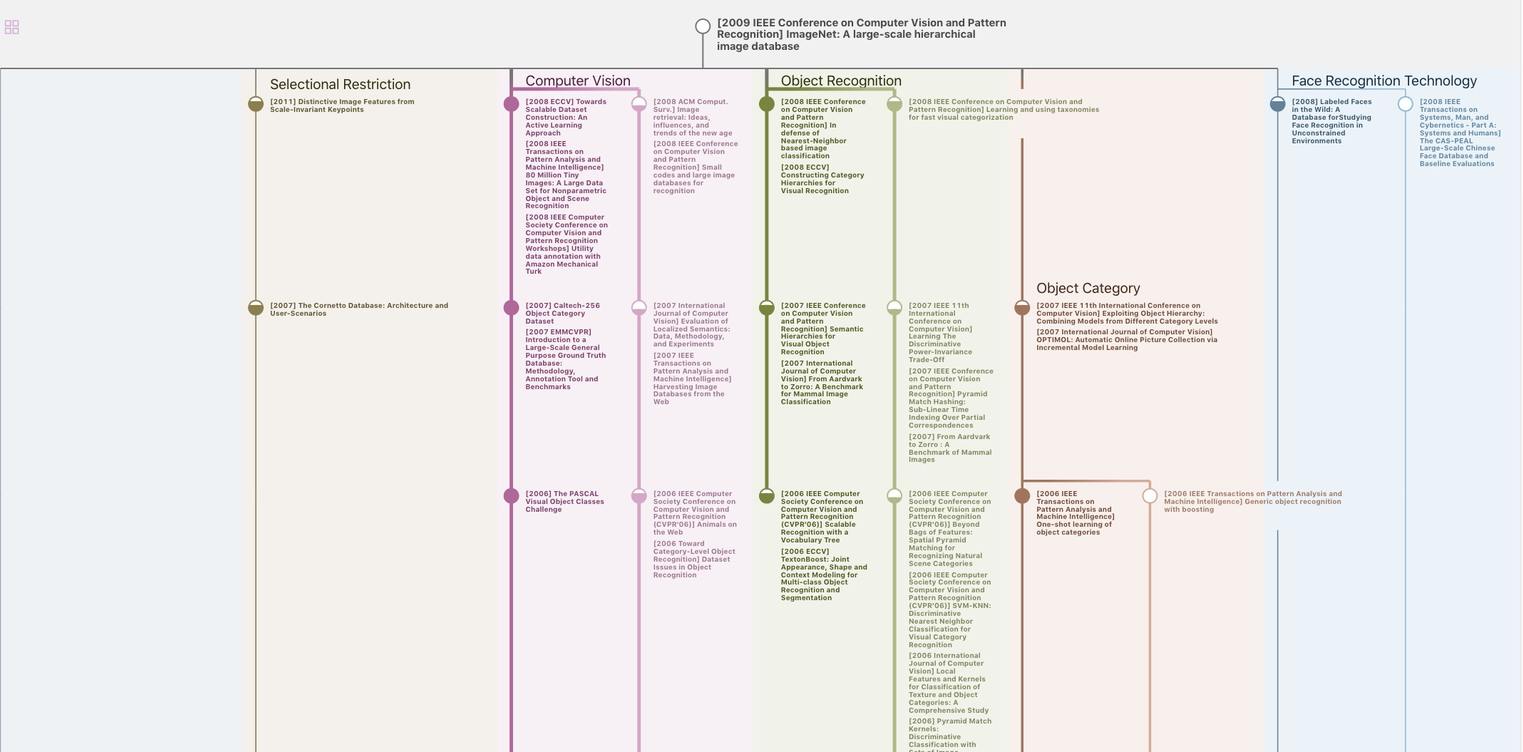
生成溯源树,研究论文发展脉络
Chat Paper
正在生成论文摘要