Towards Unbiased Exploration in Partial Label Learning
CoRR(2023)
摘要
We consider learning a probabilistic classifier from partially-labelled supervision (inputs denoted with multiple possibilities) using standard neural architectures with a softmax as the final layer. We identify a bias phenomenon that can arise from the softmax layer in even simple architectures that prevents proper exploration of alternative options, making the dynamics of gradient descent overly sensitive to initialisation. We introduce a novel loss function that allows for unbiased exploration within the space of alternative outputs. We give a theoretical justification for our loss function, and provide an extensive evaluation of its impact on synthetic data, on standard partially labelled benchmarks and on a contributed novel benchmark related to an existing rule learning challenge.
更多查看译文
关键词
unbiased exploration,label,learning
AI 理解论文
溯源树
样例
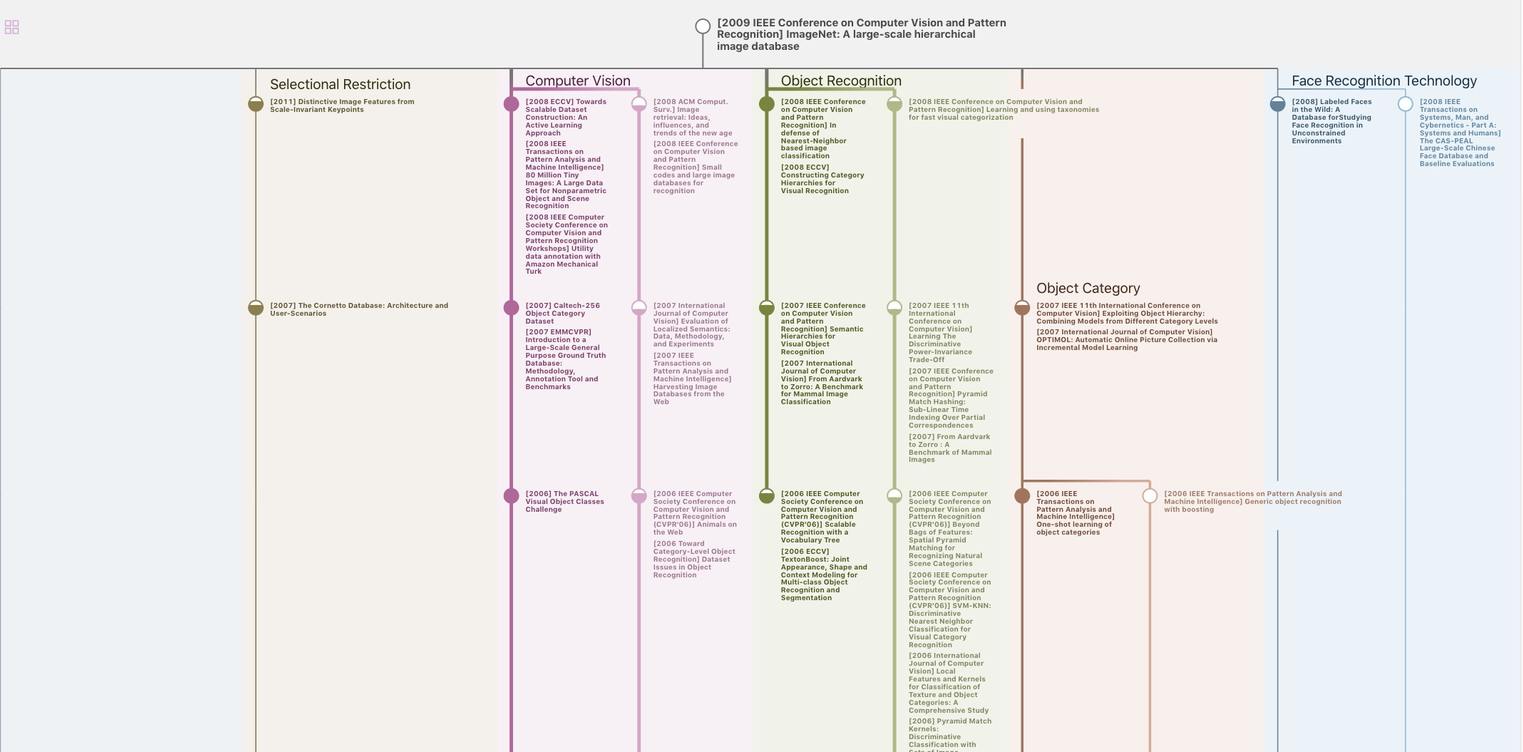
生成溯源树,研究论文发展脉络
Chat Paper
正在生成论文摘要