Referring Video Object Segmentation with Inter-Frame Interaction and Cross-Modal Correlation
CoRR(2023)
摘要
Referring video object segmentation (RVOS) aims to segment the target object in a video sequence described by a language expression. Typical query-based methods process the video sequence in a frame-independent manner to reduce the high computational cost, which however affects the performance due to the lack of inter-frame interaction for temporal coherence modeling and spatio-temporal representation learning of the referred object. Besides, they directly adopt the raw and high-level sentence feature as the language queries to decode the visual features, where the weak correlation between visual and linguistic features also increases the difficulty of decoding the target information and limits the performance of the model. In this paper, we proposes a novel RVOS framework, dubbed IFIRVOS, to address these issues. Specifically, we design a plug-and-play inter-frame interaction module in the Transformer decoder to efficiently learn the spatio-temporal features of the referred object, so as to decode the object information in the video sequence more precisely and generate more accurate segmentation results. Moreover, we devise the vision-language interaction module before the multimodal Transformer to enhance the correlation between the visual and linguistic features, thus facilitating the process of decoding object information from visual features by language queries in Transformer decoder and improving the segmentation performance. Extensive experimental results on three benchmarks validate the superiority of our IFIRVOS over state-of-the-art methods and the effectiveness of our proposed modules.
更多查看译文
AI 理解论文
溯源树
样例
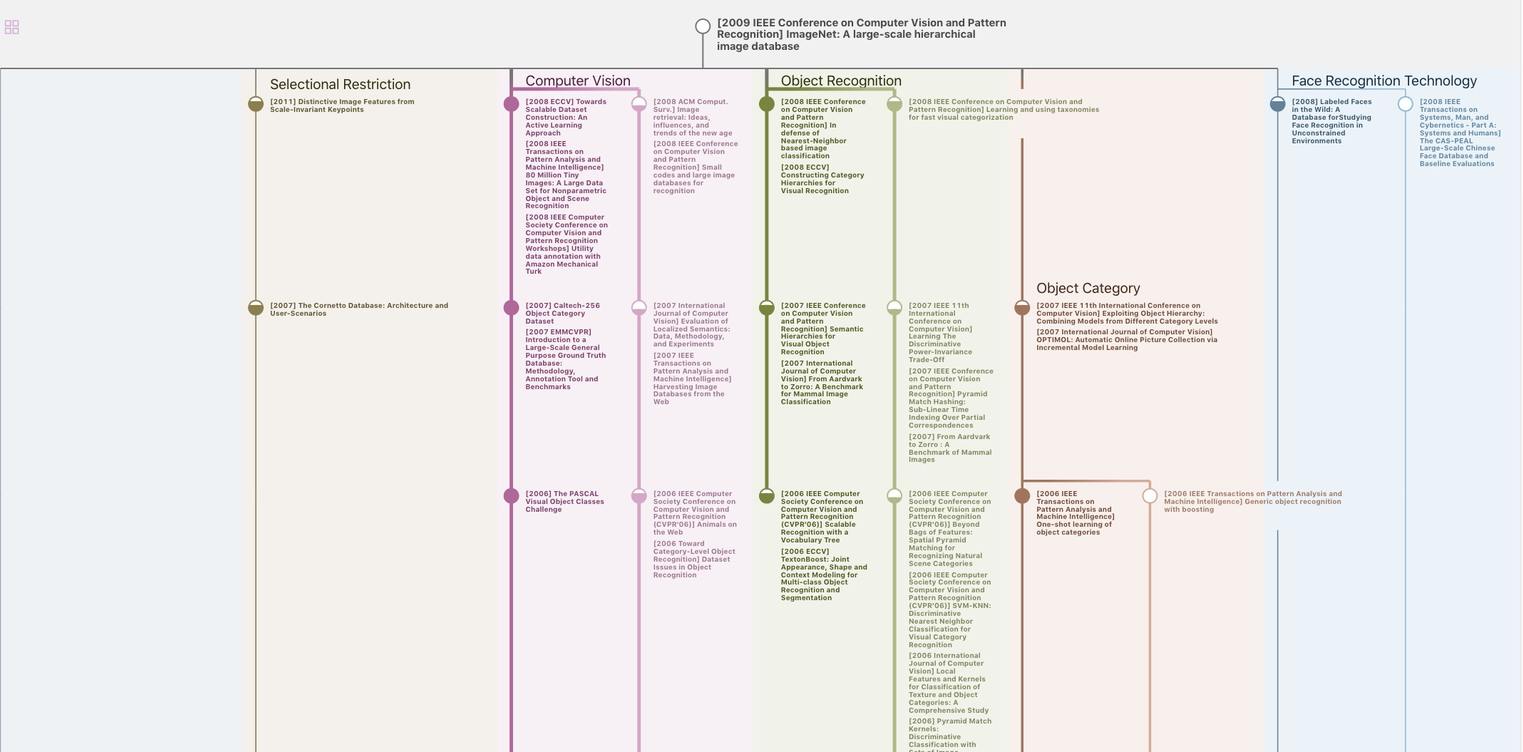
生成溯源树,研究论文发展脉络
Chat Paper
正在生成论文摘要