Active Sensing with Predictive Coding and Uncertainty Minimization
CoRR(2023)
摘要
We present an end-to-end procedure for embodied exploration based on two biologically inspired computations: predictive coding and uncertainty minimization. The procedure can be applied to any exploration setting in a task-independent and intrinsically driven manner. We first demonstrate our approach in a maze navigation task and show that our model is capable of discovering the underlying transition distribution and reconstructing the spatial features of the environment. Second, we apply our model to the more complex task of active vision, where an agent must actively sample its visual environment to gather information. We show that our model is able to build unsupervised representations that allow it to actively sample and efficiently categorize sensory scenes. We further show that using these representations as input for downstream classification leads to superior data efficiency and learning speed compared to other baselines, while also maintaining lower parameter complexity. Finally, the modularity of our model allows us to analyze its internal mechanisms and to draw insight into the interactions between perception and action during exploratory behavior.
更多查看译文
关键词
active sensing,predictive coding,uncertainty
AI 理解论文
溯源树
样例
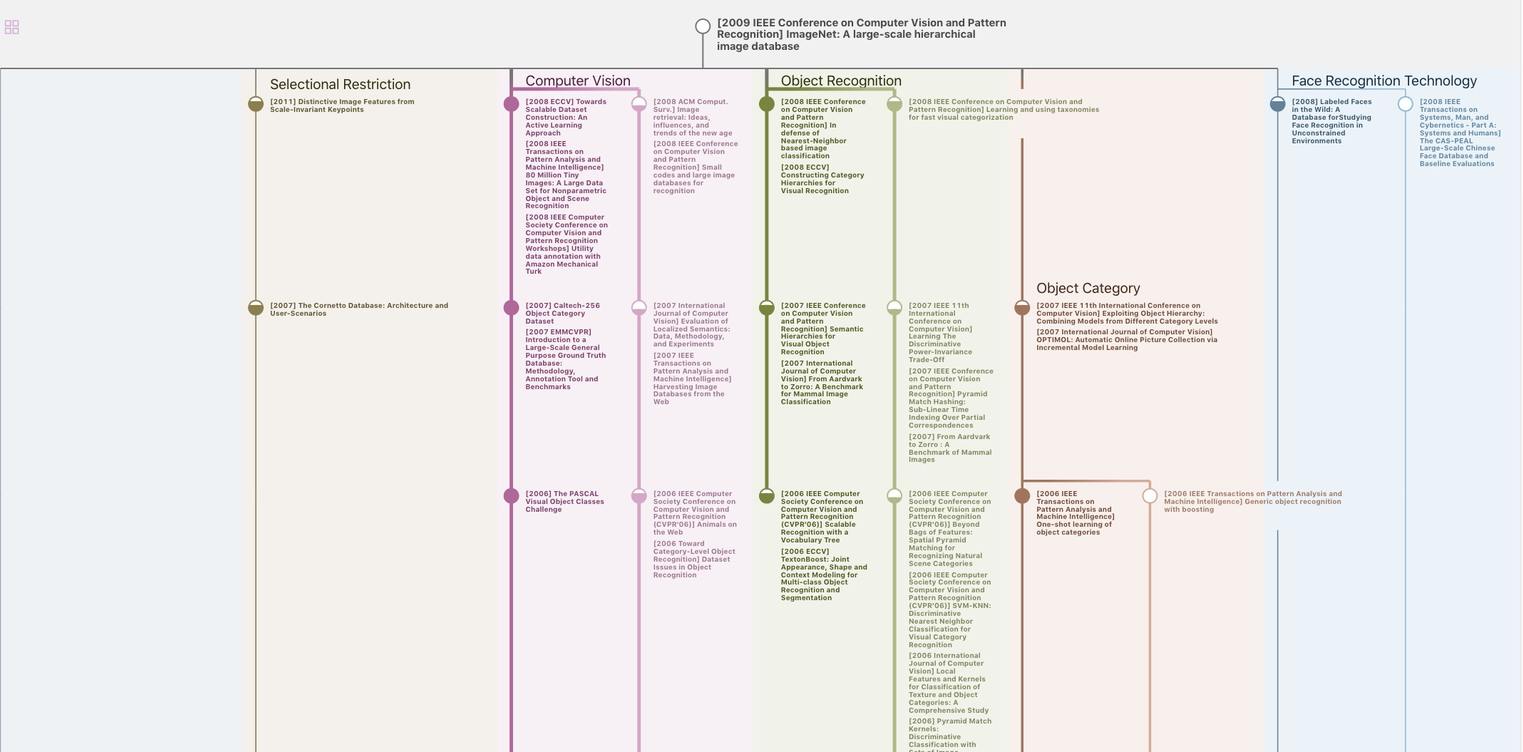
生成溯源树,研究论文发展脉络
Chat Paper
正在生成论文摘要