Engression: Extrapolation for Nonlinear Regression?
CoRR(2023)
摘要
Extrapolation is crucial in many statistical and machine learning applications, as it is common to encounter test data outside the training support. However, extrapolation is a considerable challenge for nonlinear models. Conventional models typically struggle in this regard: while tree ensembles provide a constant prediction beyond the support, neural network predictions tend to become uncontrollable. This work aims at providing a nonlinear regression methodology whose reliability does not break down immediately at the boundary of the training support. Our primary contribution is a new method called `engression' which, at its core, is a distributional regression technique for pre-additive noise models, where the noise is added to the covariates before applying a nonlinear transformation. Our experimental results indicate that this model is typically suitable for many real data sets. We show that engression can successfully perform extrapolation under some assumptions such as a strictly monotone function class, whereas traditional regression approaches such as least-squares regression and quantile regression fall short under the same assumptions. We establish the advantages of engression over existing approaches in terms of extrapolation, showing that engression consistently provides a meaningful improvement. Our empirical results, from both simulated and real data, validate these findings, highlighting the effectiveness of the engression method. The software implementations of engression are available in both R and Python.
更多查看译文
关键词
nonlinear regression,extrapolation
AI 理解论文
溯源树
样例
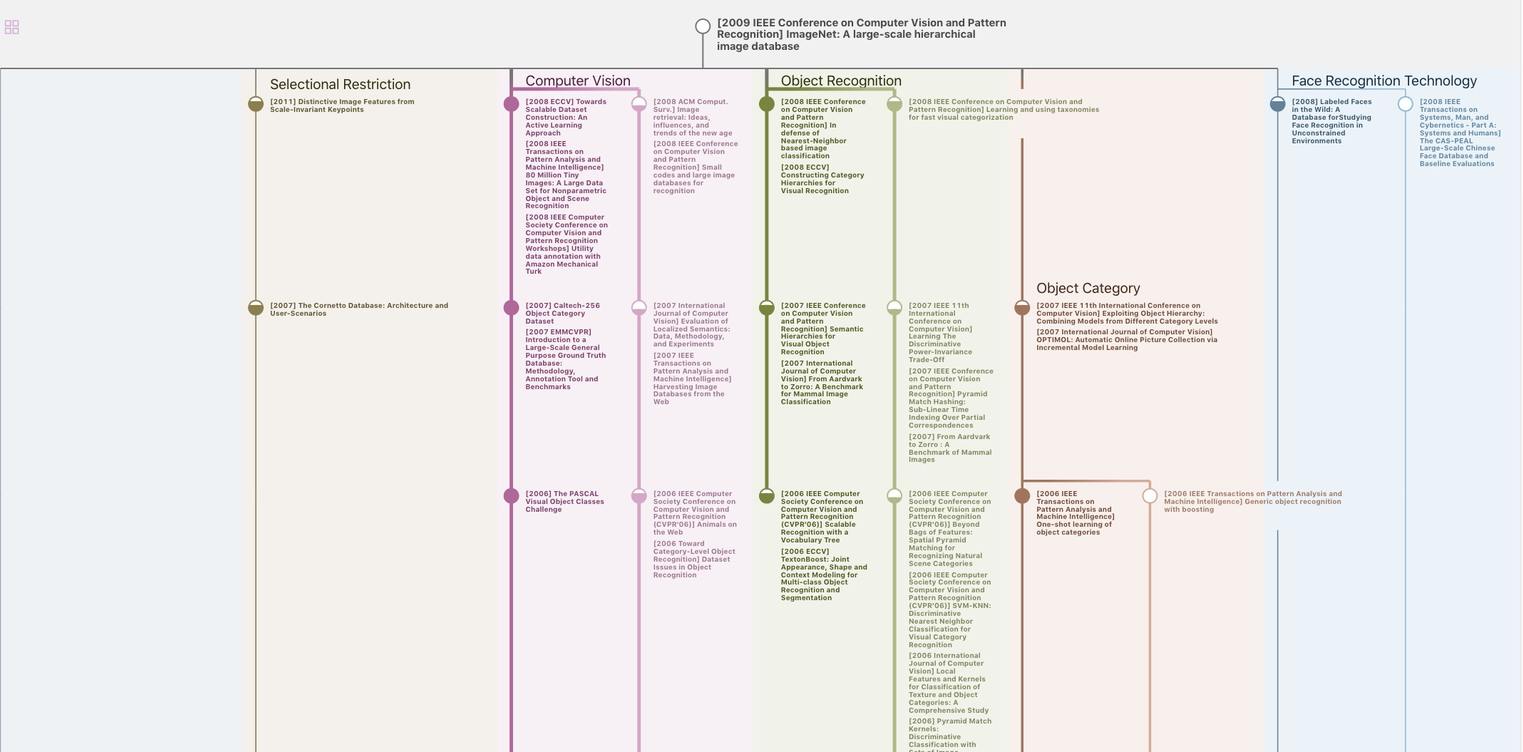
生成溯源树,研究论文发展脉络
Chat Paper
正在生成论文摘要