A non-intrusive bi-fidelity reduced basis method for time-independent problems
JOURNAL OF COMPUTATIONAL PHYSICS(2024)
摘要
Scientific and engineering problems often involve parametric partial differential equations (PDEs), such as uncertainty quantification, optimizations, and inverse problems. However, solving these PDEs repeatedly can be prohibitively expensive, especially for large-scale complex applications. To address this issue, reduced order modeling (ROM) has emerged as an effective method to reduce computational costs. However, ROM often requires significant modifications to the existing code, which can be time-consuming and complex, particularly for large-scale legacy codes. Non -intrusive methods have gained attention as an alternative approach. However, most existing non -intrusive approaches are purely data -driven and may not respect the underlying physics laws during the online stage, resulting in less accurate approximations of the reduced solution. In this study, we propose a new non -intrusive bi-fidelity reduced basis method for time -independent parametric PDEs. Our algorithm utilizes the discrete operator, solutions, and right-hand sides obtained from the high-fidelity legacy solver. By leveraging a low -fidelity model, we efficiently construct the reduced operator and right-hand side for new parameter values during the online stage. Unlike other non -intrusive ROM methods, we enforce the reduced equation during the online stage. In addition, the non -intrusive nature of our algorithm makes it straightforward and applicable to general nonlinear time -independent problems. We demonstrate its performance through several benchmark examples, including nonlinear and multiscale PDEs.
更多查看译文
关键词
Reduced order modeling,Non-intrusive methods,Multi-fidelity modeling
AI 理解论文
溯源树
样例
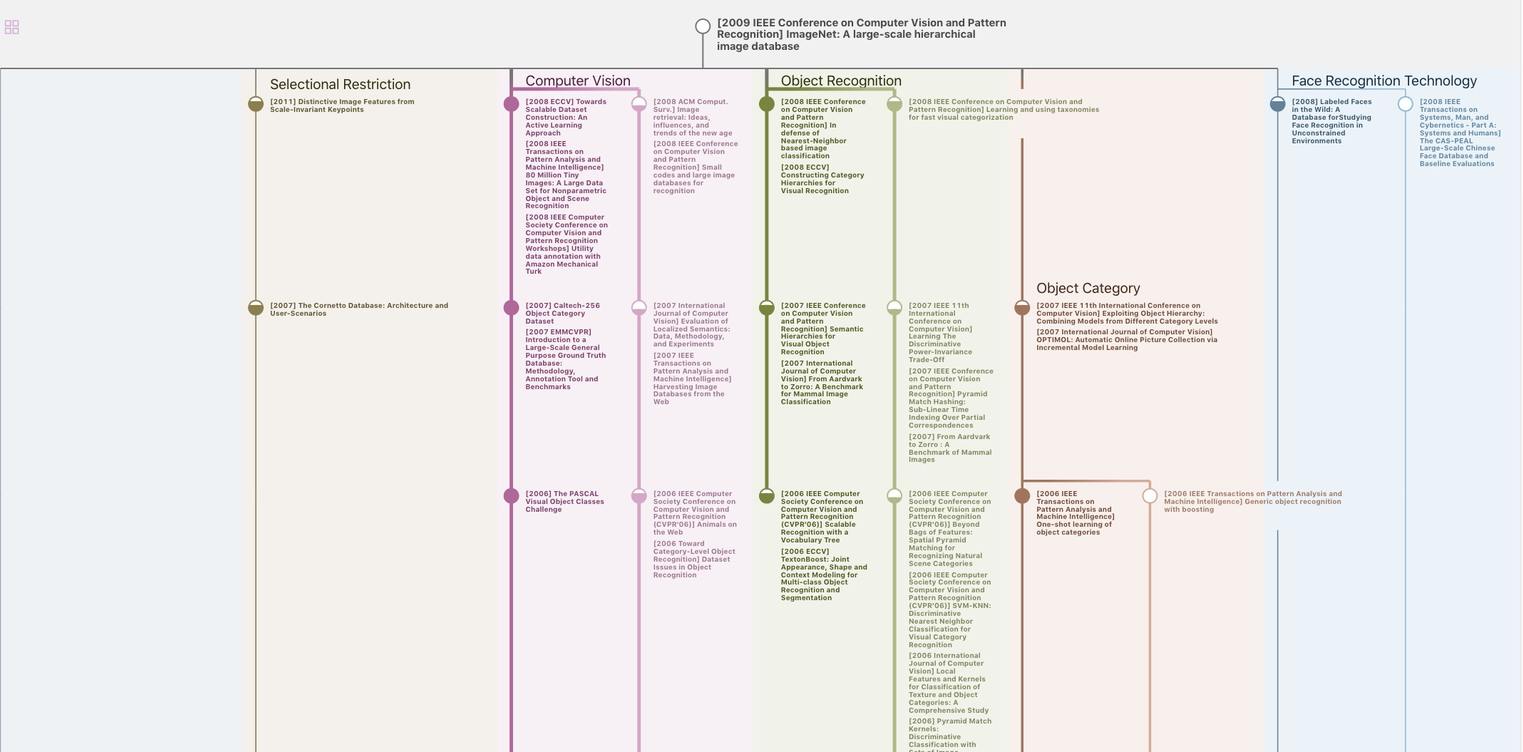
生成溯源树,研究论文发展脉络
Chat Paper
正在生成论文摘要