A Deep Neural Network-based Nonlinear Dynamics Model for the Prediction of Lateral-longitudinal Vehicle Dynamics
Research Square (Research Square)(2021)
摘要
Abstract The vehicle multibody model can be used for accurate coupling dynamics, but it has higher computational complexity. Numerical stability during integration is also very challenging, especially in complicated driving situations. This issue can be substantially alleviated by using a data-driven nonlinear dynamics model owing to its computational speed and robust generalization. In this work, we propose a deep neural network (DNN)-based modeling approach for predicting lateral-longitudinal vehicle dynamics. Dynamic simulations of vehicle systems are performed based on a semirecursive multibody formulation for data acquisition. The data are then used for training and testing the DNN model. The DNN inputs are the torque applied on wheels and the initial vehicle speed that imitates a double lane change maneuver with acceleration and deceleration. The DNN outputs are the longitudinal driving distance, lateral driving distance, final longitudinal velocities, final lateral velocities, and yaw angle. The dynamic responses obtained from the DNN model are compared with the multibody results. Furthermore, the accuracy of the DNN model is investigated in terms of error functions. The DNN model is finally verified via the results of a commercial software package. The results show that the DNN vehicle dynamics model predicts accurate dynamic responses in real time. The DNN model can be used for real-time simulation and preview control in autonomous vehicles.
更多查看译文
关键词
nonlinear dynamics model,nonlinear dynamics,network-based,lateral-longitudinal
AI 理解论文
溯源树
样例
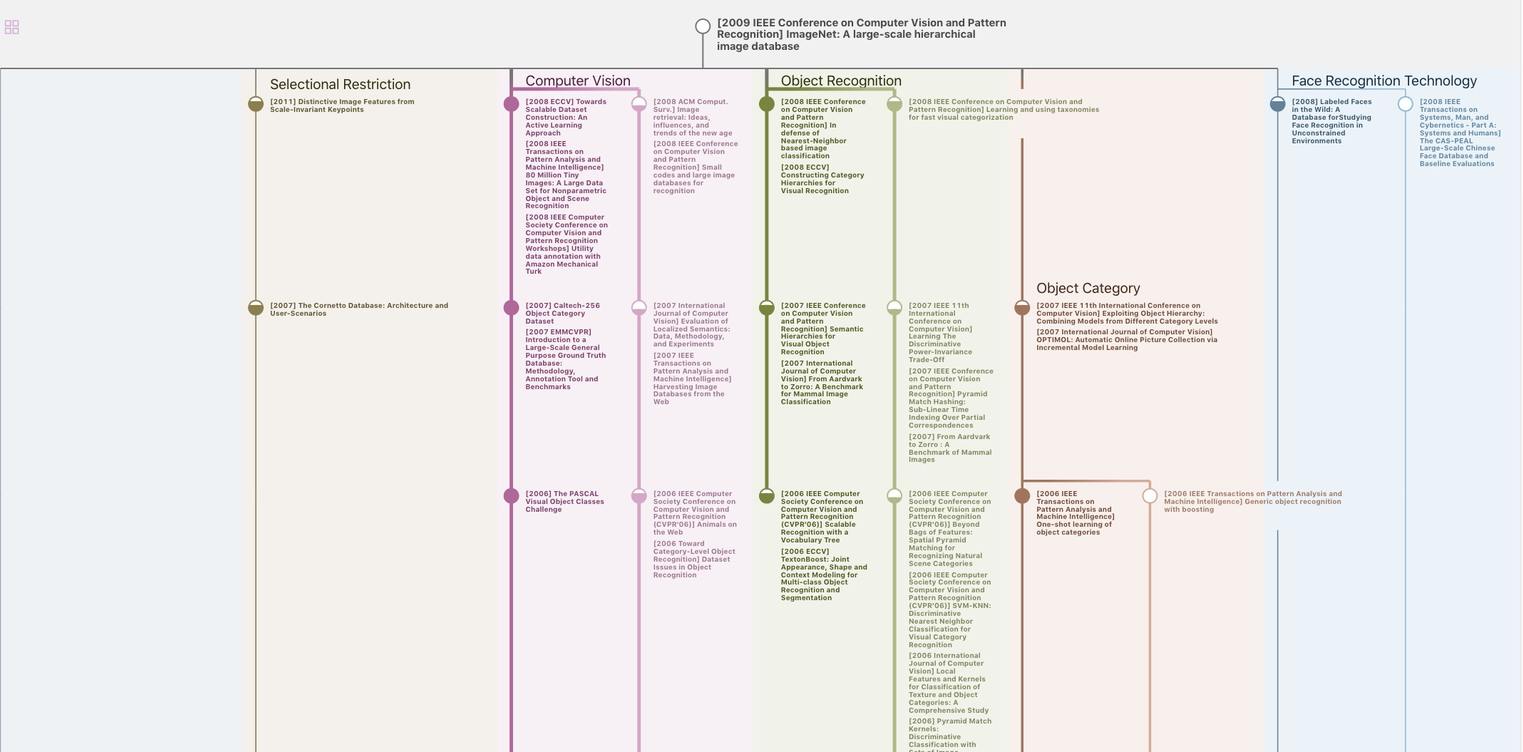
生成溯源树,研究论文发展脉络
Chat Paper
正在生成论文摘要