Quickly Transforming Discriminator in Pre-Trained GAN to Encoder
crossref(2021)
摘要
Abstract-- Fine-designed deep Generative Adversarial Networks (GANs) can generate high-quality (HQ) images. However, the discriminator in GAN only plays a role to distinguish candidates produced by the generator from the true data distribution, and numerous generated samples are still not clear and true. From pre-trained GAN, we offer a self-supervised method to quickly transform the discriminator into an encoder and fine-tune the pre-trained GAN to an auto-encoder. The parameters of the pre-trained discriminator are reused and converted into an encoder for outputting reformed latent space. The transformation changes the previous GAN to a symmetrical architecture and the generator can reconstruct the HQ image by reforming latent space. By fixing the generator, the reformed latent space can perform better representation than the pre-trained GAN, and the performance of the pre-trained GAN can be improved by the transformed encoder.
更多查看译文
AI 理解论文
溯源树
样例
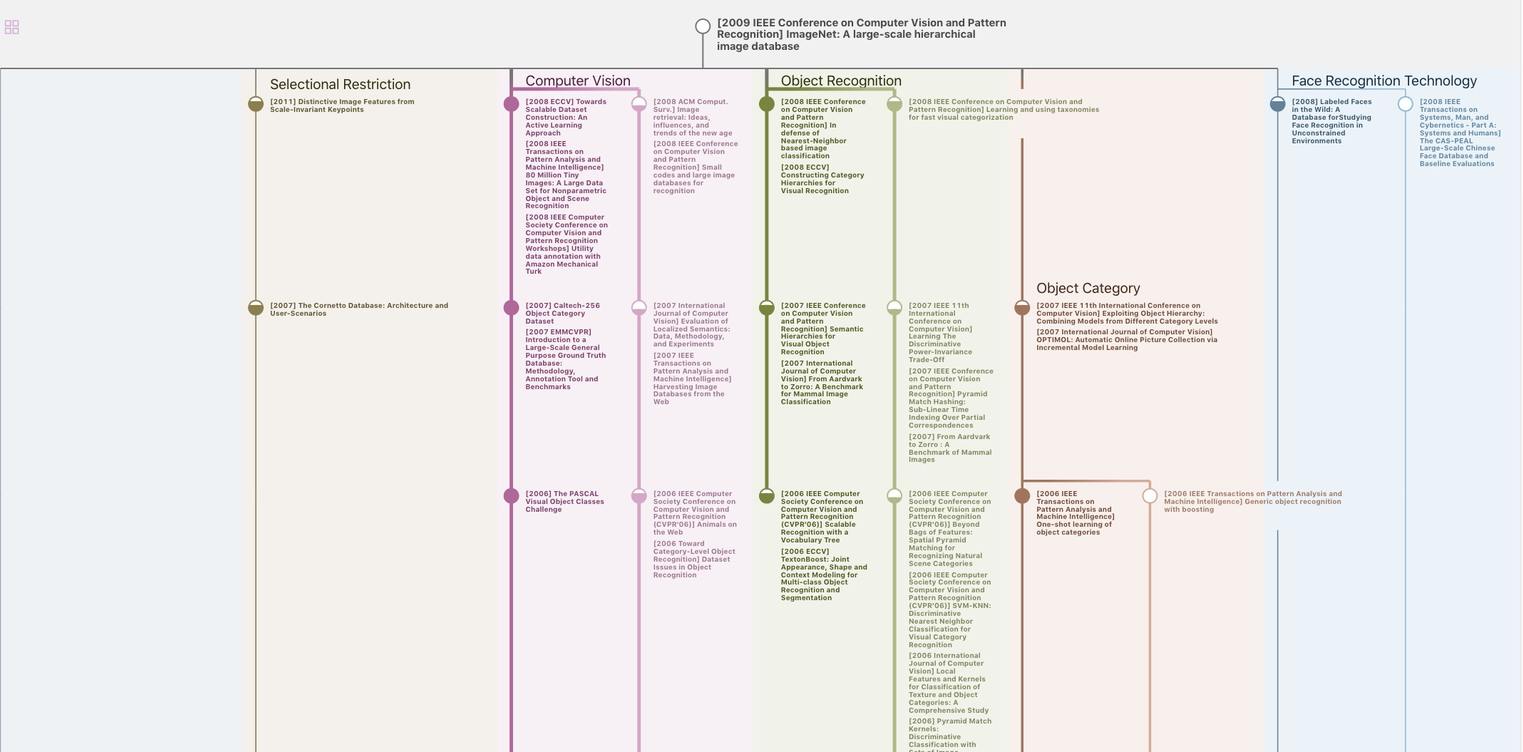
生成溯源树,研究论文发展脉络
Chat Paper
正在生成论文摘要