Multi-objective optimization for improving machining benefit based on WOA-BBPN and a Deep Double Q-Network.
Appl. Soft Comput.(2023)
摘要
To scientifically and reasonably set and adjust the machining parameters, and improve the machining benefit of the batch machining process including machining quality, performance and efficiency, this paper proposes a low cost and high accurate multi-objective optimization framework by integrating intelligent technologies from three perspectives of physical experiment design, objective function construction and multi-objective optimization. In this framework, multilayer design (MLD) which is a computer experiment design method with low redundancy, good uniformity and filling is applied to the machining physics experiment design to reduce the experiment cost. Whale optimization algorithm (WOA) optimized backpropagation neural network (BBPN) is utilized to construct the optimization objective functions to improve the accuracy of functions. And Deep Double Q-Network (DDQN) is employed to achieve multi-objective optimization of machining quality, efficiency, and performance. The effectiveness of MLD, WOA-BBPN and DDQN in multi-objective optimization of machining process are verified by comparisons of common modeling and multi-objective optimization algorithms based on the milling data. These techniques provide a good guidance for the selection of machining parameters to improve the machining benefit and demonstrates some advantages. The research of this paper also offers a good example of applying intelligent technology in machining.
更多查看译文
关键词
Backpropagation Neural Network, Deep double Q-Network, Machining benefit, Multilayer design, Whale optimization algorithm
AI 理解论文
溯源树
样例
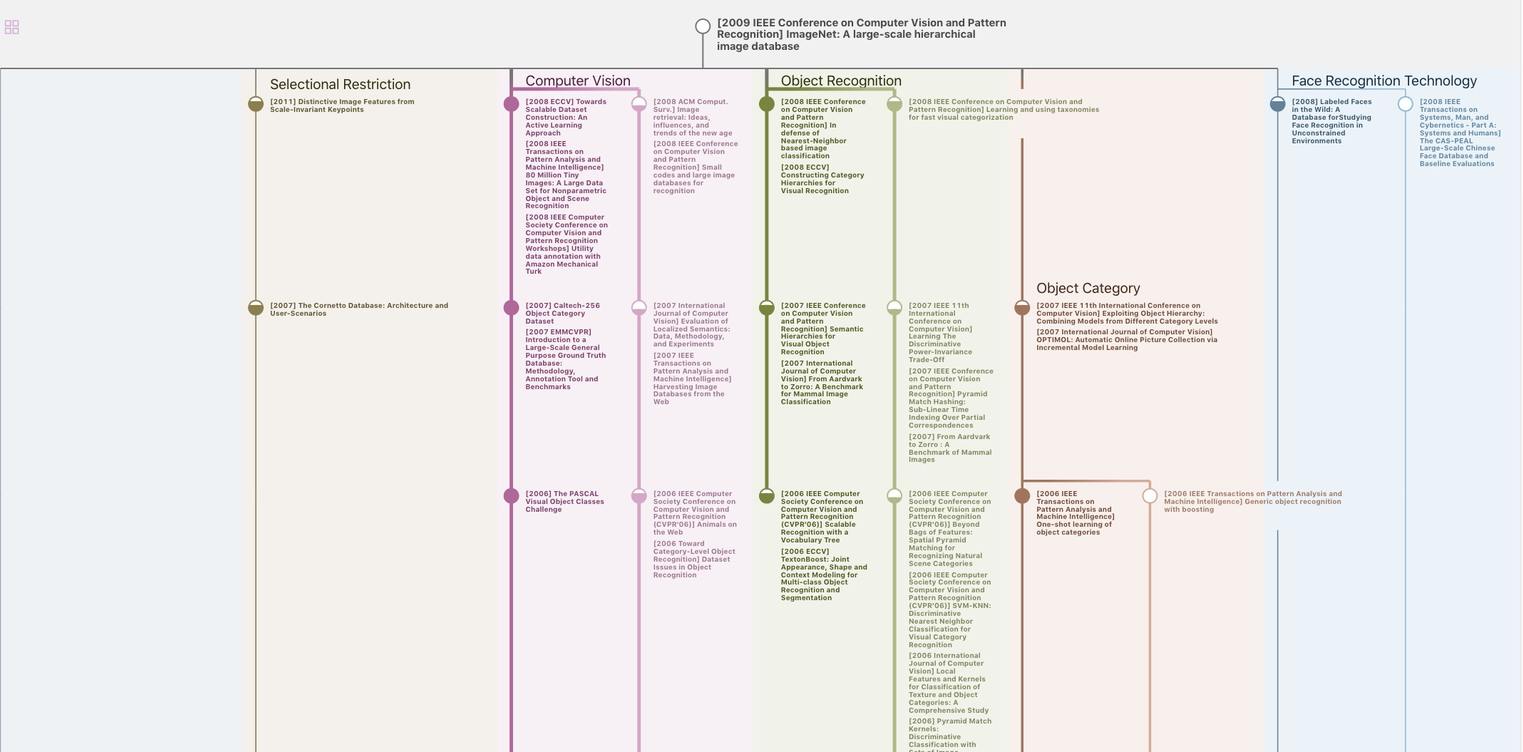
生成溯源树,研究论文发展脉络
Chat Paper
正在生成论文摘要