Optimal Wavelength Selection for Deep Learning from Hyperspectral Images.
IbPRIA(2023)
摘要
While hyperspectral images typically contain two orders of magnitude more information about the detailed colour spectrum of the object under study compared to RGB images, this inevitably leads to larger storage costs and larger bandwidth requirements. This, in consequence, typically makes it challenging to use such images for real-time industrial defect detection. A second challenge of working with images having tens to hundreds of colour channels is that most available deep-learning (DL) networks are designed for images with three colours. In this paper, we will demonstrate that training typical DL segmentation networks on the full hyperspectral colour channel stack performs differently than when training on only three channels. We will also test two different approaches (Greedy Selection and Bayesian Optimization) to select the N-best wavelengths. For a given use-case, we demonstrate how optimal 3-wavelength images can outperform baseline wavelength selection methods as RGB and PCA (principal component analysis) as well as outperform networks trained with the full colour channel stack.
更多查看译文
关键词
optimal wavelength selection,hyperspectral images,deep learning
AI 理解论文
溯源树
样例
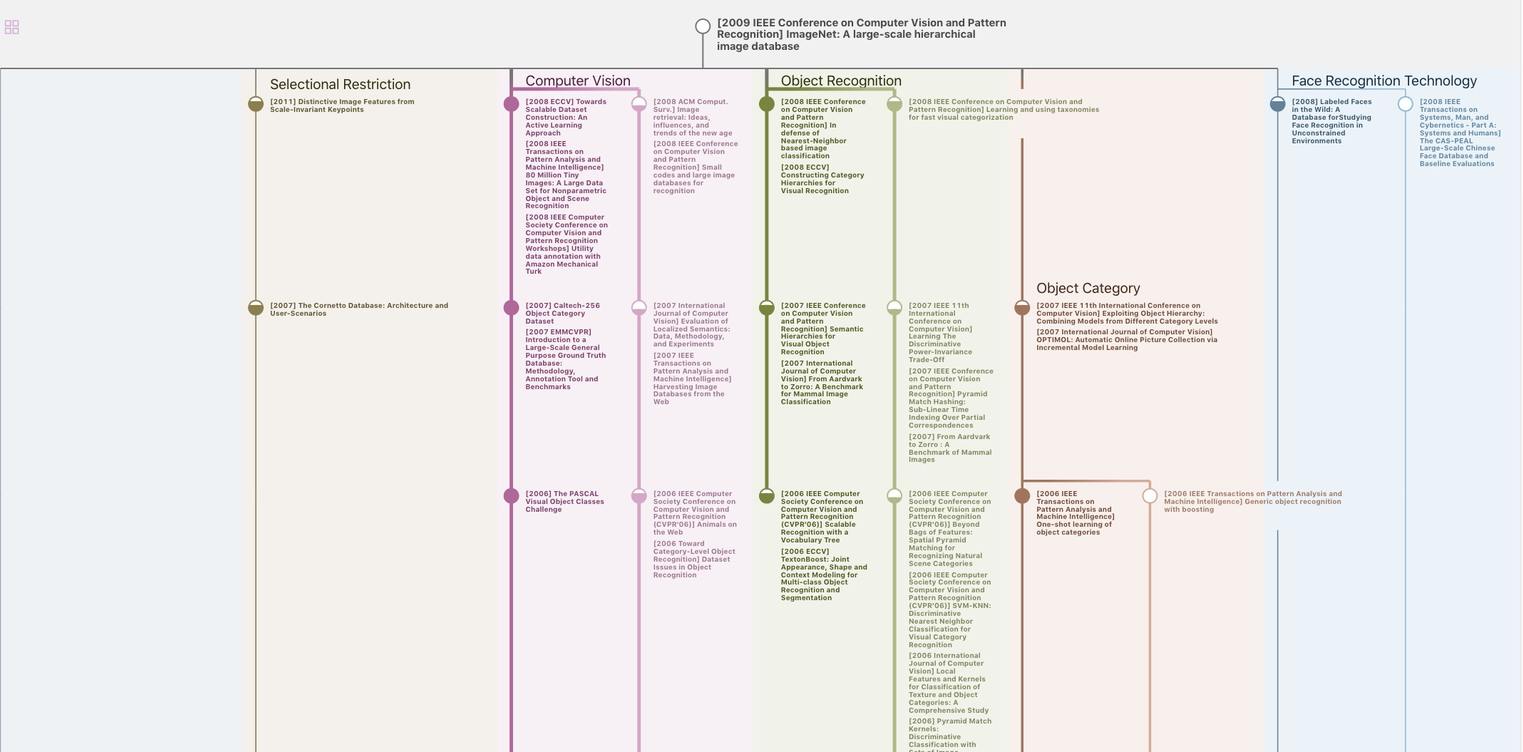
生成溯源树,研究论文发展脉络
Chat Paper
正在生成论文摘要