Overcoming Attenuation Bias in Regressions using Polygenic Indices: A Comparison of Approaches
bioRxiv (Cold Spring Harbor Laboratory)(2021)
摘要
Measurement error in polygenic indices (PGIs) attenuates the estimation of their effects in regression models. While this measurement error shrinks with growing Genome-wide Association Study (GWAS) sample sizes, the marginal returns to bigger sample sizes are rapidly decreasing. We analyze and compare two alternative approaches to reduce measurement error: Obviously Related Instrumental Variables (ORIV) and the PGI Repository Correction (PGI-RC). Through simulations, we show that both approaches outperform the typical (meta-analysis based) PGI in terms of bias and root mean squared error. Between families, the PGI-RC performs slightly better than ORIV, unless the prediction sample is very small (N < 1, 000), or when there is considerable assortative mating. Within families, ORIV is the default choice since the PGI-RC is not available in this setting. We verify the empirical validity of the simulations by predicting educational attainment (EA) and height in a sample of siblings from the UK Biobank. We show that applying ORIV between families increases the standardized effect of the PGI by 12% (height) and by 22% (EA) compared to a meta-analysis-based PGI, yet remains slightly below the PGI-RC estimates. Furthermore, within-family ORIV regression provides the tightest lower bound for the direct genetic effect, increasing the lower bound for the direct genetic effect on EA from 0.14 to 0.18, and for height from 0.54 to 0.61 compared to a meta-analysis-based PGI.
更多查看译文
关键词
polygenic indices,attenuation bias,regressions
AI 理解论文
溯源树
样例
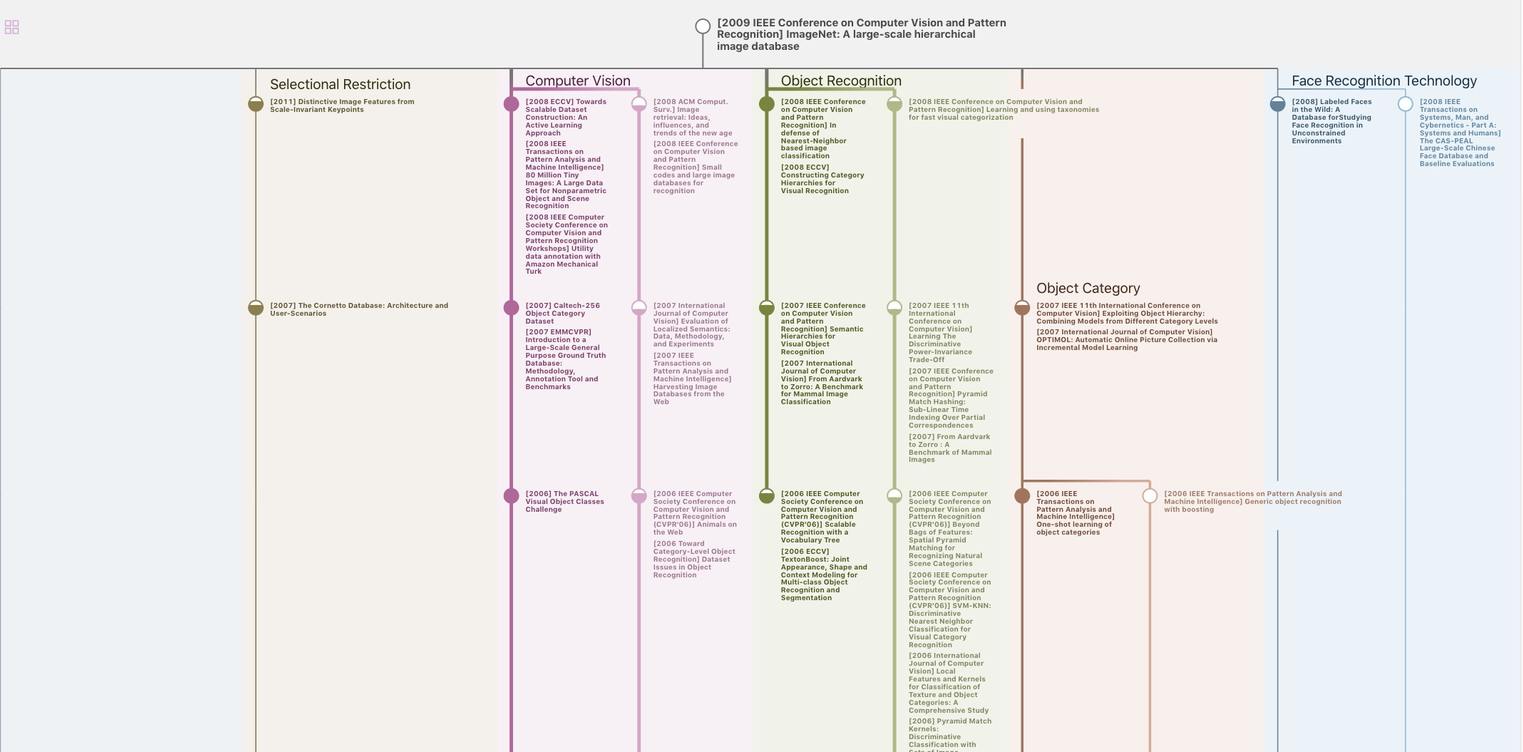
生成溯源树,研究论文发展脉络
Chat Paper
正在生成论文摘要