LSR-BO: Local Search Region Constrained Bayesian Optimization for Performance Optimization of Vapor Compression Systems
2023 AMERICAN CONTROL CONFERENCE, ACC(2023)
摘要
Bayesian optimization (BO) has recently been demonstrated as a powerful tool for efficient derivative-free optimization of expensive black-box functions, such as those prevalent in performance optimization of complex energy systems. Classical BO algorithms ignore the relationship between consecutive optimizer candidates, resulting in jumps in the admissible search space which can lead to fail-safe mechanisms being triggered, or undesired transient dynamics that violate operational constraints. In this paper, we propose LSR-BO, a novel global optimization methodology that enforces local search region (LSR) constraints by design, which restricts how much the optimizer candidate can be changed at every iteration. We demonstrate that naively incorporating LSR constraints into BO causes the algorithm to get stuck in local suboptimal solutions, and overcome this challenge through the development a novel exploration strategy that can gracefully navigate the trade-off between short-term "local", and long-term "global", performance improvement. Furthermore, we provide theoretical guarantees on the convergence of LSR-BO. Finally, we verify the effectiveness of our proposed LSR-BO method on an illustrative benchmark and a real-world energy minimization problem for a commercial vapor compression system.
更多查看译文
关键词
Probabilistic machine learning,Black-box optimization,Bayesian methods,Energy systems
AI 理解论文
溯源树
样例
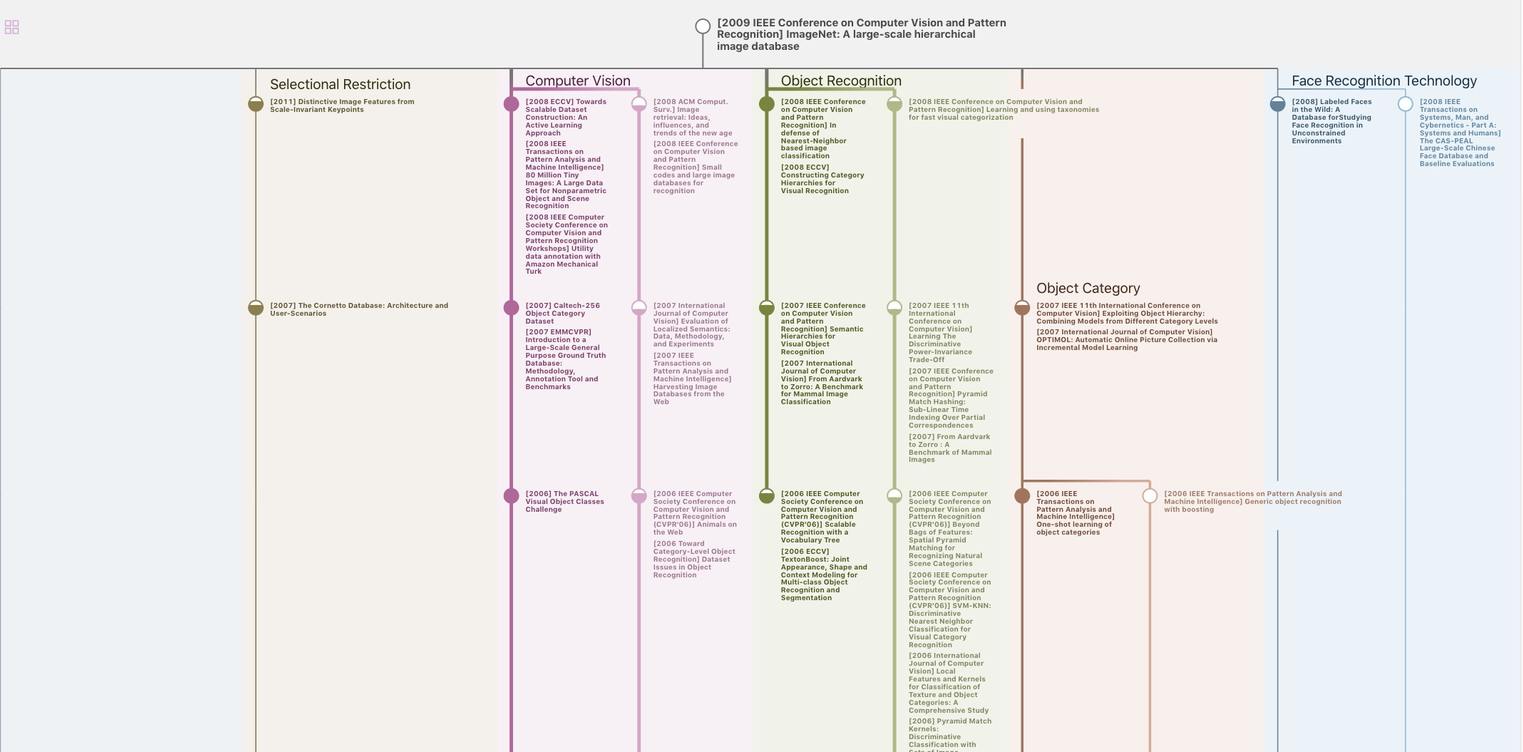
生成溯源树,研究论文发展脉络
Chat Paper
正在生成论文摘要