Comparing in situ phenology and remotely derived phenometrics across ecosystems
crossref(2021)
摘要
<p>The use of satellite sensors, near-surface cameras and other remote methods of monitoring vegetation phenology at landscape and higher scales has become increasingly common. These technologies provide a means to determine the timing of phenophases and growing season length at different spatial resolutions; coverage that is not attainable by human observers. However, in situ ground observations are necessary to validate remotely derived phenometrics. Despite increased knowledge and expertise there still remains the persistent challenge of reconciling ground observations at the individual plant level with remotely sensed (RS) phenometrics at landscape or larger scales. Here, we compared the timing of in situ phenophase estimates (spring and autumn) with a range of corresponding remote sensing (MODIS, VIIRS, PhenoCam) phenometrics across five terrestrial sites in the USA’s National Ecological Observatory Network (NEON). The sites represent a range of ecosystem types including, deciduous forest (Harvard Forest, MA), dry scrubland (Onaqui, UT), evergreen forest (Abby Road, WA) and seasonal wetlands (Disney Wilderness Preserve and Ordway-Swisher Biological Station, FL) focusing on a three year period from 2017-2019. Our main objective was compare a range of co-located RS pheometrics with in situ observations to explore potential reasons for the observed discrepancies and to determine which technologies were more aligned with ground observations. Statistically significant relationships were strongest (p<0.001) for spring phenophases compared to autumn. In general, satellite derived phenometrics tended to be earlier (RMSE 21.7 – 28.4 days) than in situ spring phenology whereas PhenoCam derived phenometrics were later (RMSE 24 days). Overall, discrepancies between in situ and RS phenometrics related to scale, species availability and the short duration of the time-series (3 years). However, as the NEON project progresses these challenges are expected to be reduced as more data become available.</p>
更多查看译文
AI 理解论文
溯源树
样例
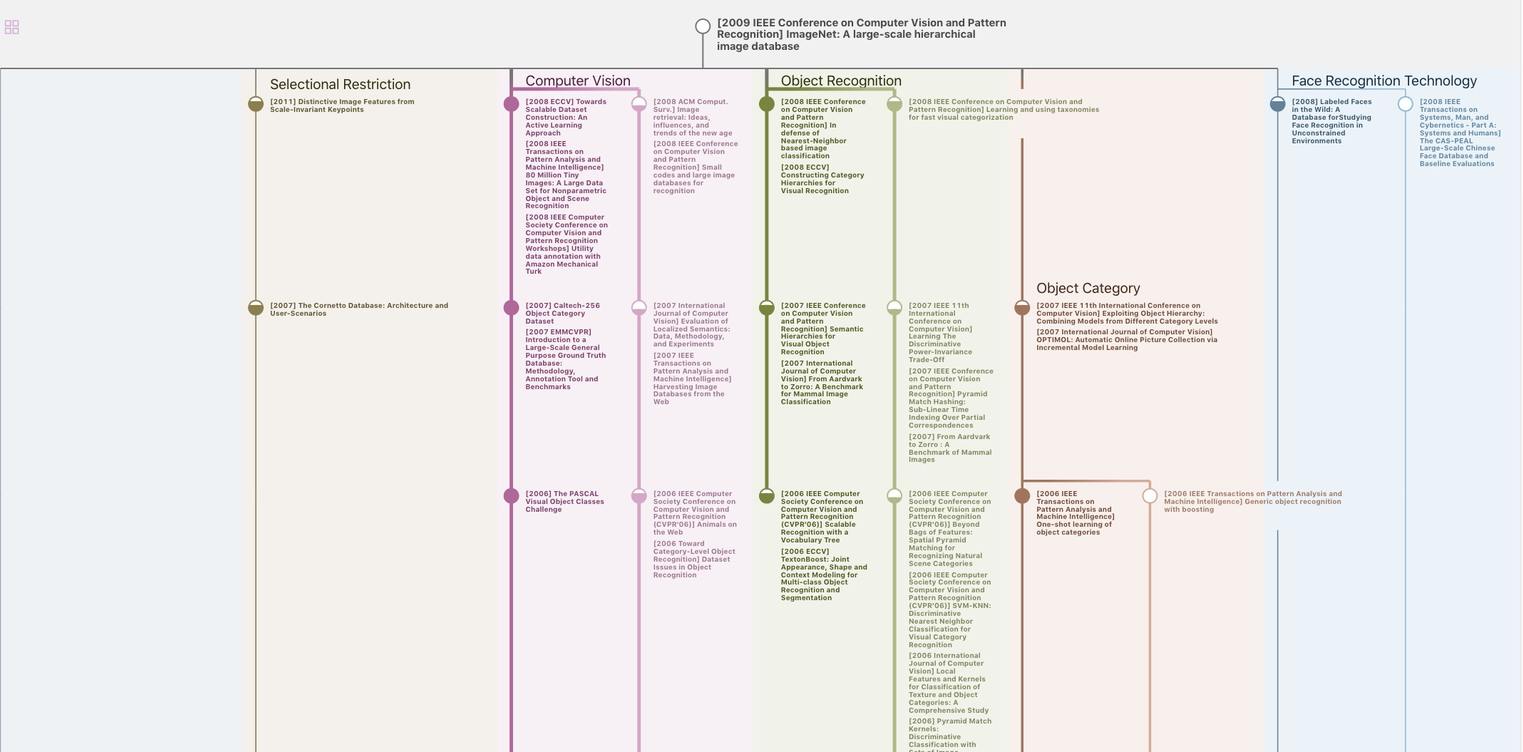
生成溯源树,研究论文发展脉络
Chat Paper
正在生成论文摘要