Early Warning Evaluation of Tuberculosis and Meteorological Factors in Shanxi Province Based on Dynamic Bayesian Network
crossref(2021)
摘要
Abstract Background: Tuberculosis is a major global public health problem. However, it is still in the exploratory stage that the study of the meteorological factors related to the incidence of tuberculosis in Shanxi Province. Therefore, it is very urgent to establish an early warning system that easily operate of tuberculosis. Method: The epidemiological characteristics of tuberculosis in Shanxi Province were described, and the Dynamic Bayesian Network early warning model was established by time series cross-correlation analysis and Bayesian Network.Results: 1. The reported incidence of tuberculosis in Shanxi Province showed an overall downward trend from 2008 to 2017, showing a phenomenon of high in the middle and low at both ends each year, with certain seasonal characteristics. 2. Based on the results of cross-correlation analysis, it is reasonable to use dynamic Bayesian model fitting with meteorological factors lagging for 2 months; the monthly average temperature and monthly precipitation are positively correlated with the incidence of tuberculosis, but the monthly mean air pressure is negatively. 3. Comparison of classification and recognition performance of the three models shows that DBN has the highest classification accuracy in the two regions, which indicates that DBN is better than the other two models in reflecting the performance of minority classes, and better for the comprehensive classification of minority classes and majority classes. Conclusion: 1. Shanxi Province has tuberculosis clustering in time, space and time and space. Incidence peak is in spring and early summer. March is the highest month in the year. Seven meteorological factors such as monthly precipitation are the main factors affecting the incidence of tuberculosis in Shanxi Province. 2. The classification and recognition performance of the Dynamic Bayesian Network early warning model of tuberculosis-meteorological factors established in this study is significantly better than that of static Bayesian Network and support vector machine model, and can better predict the future.
更多查看译文
AI 理解论文
溯源树
样例
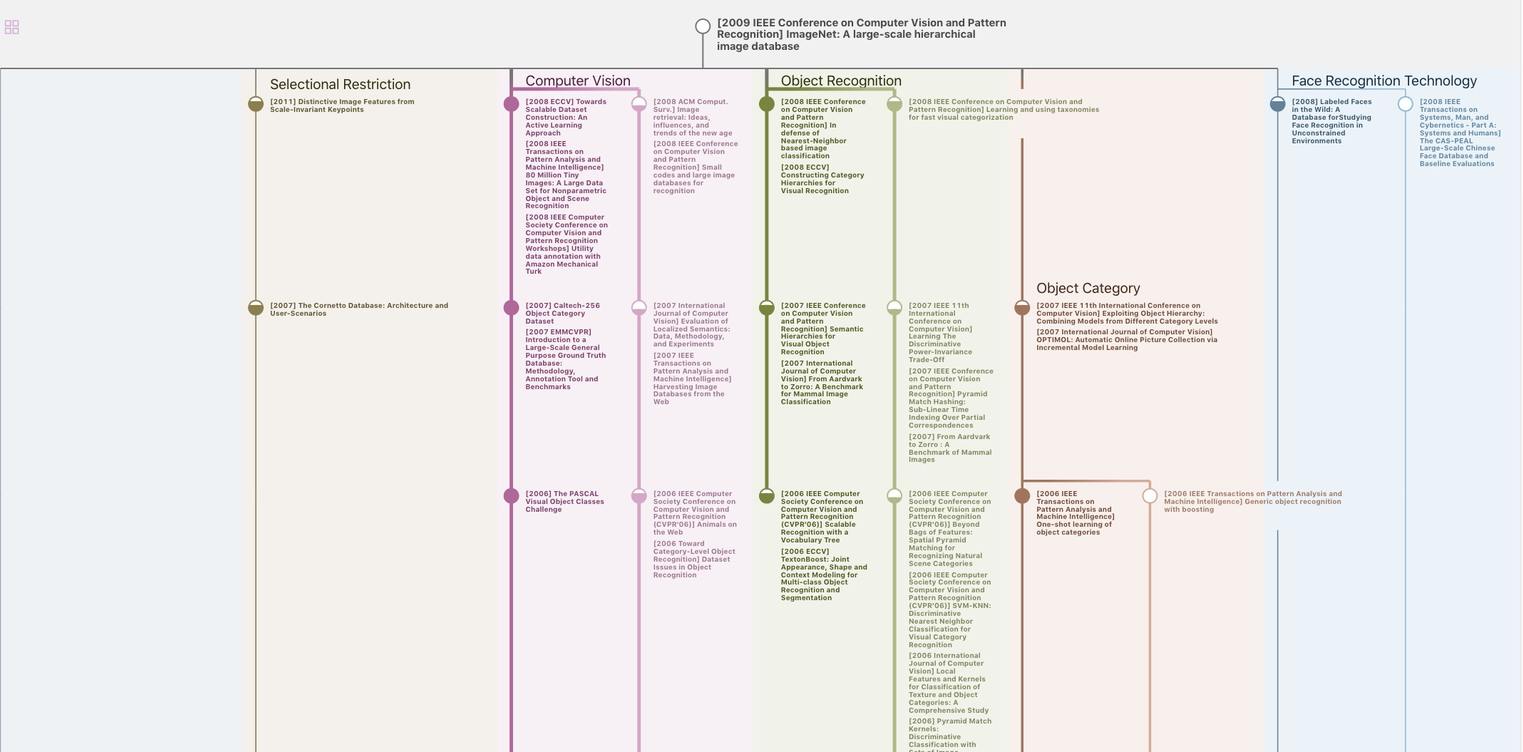
生成溯源树,研究论文发展脉络
Chat Paper
正在生成论文摘要