Developing a Novel Parameter-free Optimization Algorithm for Flood Routing
Research Square (Research Square)(2021)
摘要
Abstract The Muskingum model is a popular hydrologic flood routing method; however, the accurate estimation of Muskingum model parameters is a critical task in the successful and precise implementation of flood routing. Evolutionary and metaheuristic optimization algorithms (EMOAs) are well suited for parameter estimation associated with various complex models including the nonlinear Muskingum model. Among EMOAs, teaching-learning-based optimization (TLBO) is a relatively new parameterless metaheuristic optimization algorithm, inspired by the relationship between teacher and students in a classroom to improve the overall knowledge of a topic in a class. This paper presents an application of TLBO to estimate Muskingum model parameters by minimizing the prediction error of outflow measurements. Several examples evaluate and confirm the successful performance of TLBO for the estimation of Muskingum-routing parameters precisely. The results show TLBO-Muskingum’s high accuracy for estimating accurately Muskingum’s parameters based on the Nash-Sutcliffe Efficiency (NSE) to evaluate the TLBO’s predictive skill with benchmark problems.
更多查看译文
关键词
optimization,algorithm,parameter-free
AI 理解论文
溯源树
样例
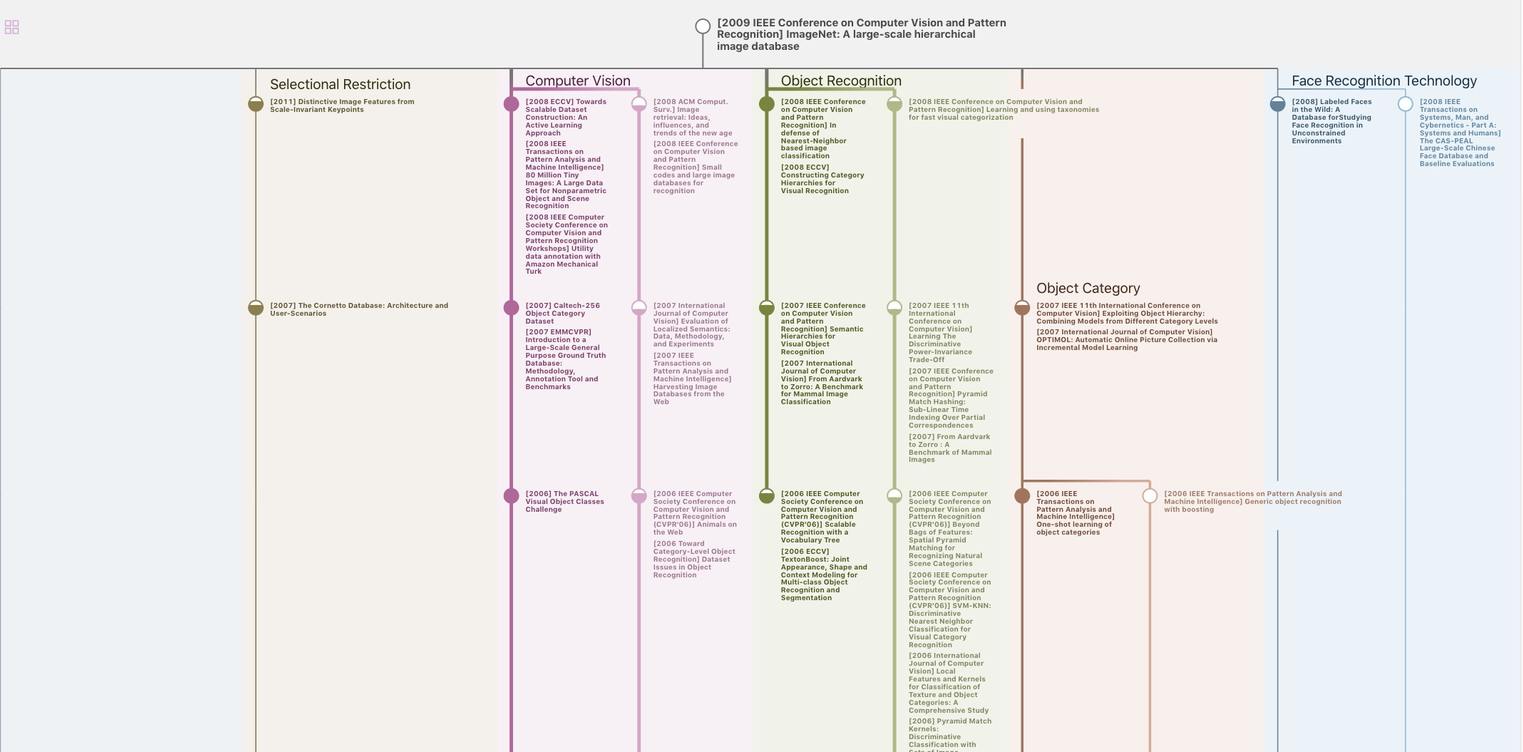
生成溯源树,研究论文发展脉络
Chat Paper
正在生成论文摘要