Multi-biological Classification for the Diagnosis of Schizophrenia Using Multi-classifier, Multi-feature Selection and Multi-cross Validation: an Integrated Machine Learning Framework Study
Research Square (Research Square)(2021)
摘要
Abstract Finding effective and objective biomarkers to inform the diagnosis of schizophrenia is of great importance yet remains challenging. However, there is relatively little work on multi-biological data for the diagnosis of schizophrenia. This was a cross-sectional study in which we extracted multiple features from three types of biological data including gut microbiota data, blood data, and electroencephalogram data. Then, an integrated framework of machine learning, consisting of five classifiers, three feature selection algorithms, and four cross-validation methods was used to discriminate patients with schizophrenia from healthy controls. Our results showed that the performance of the classifier using multi-biological data was better than that of the classifiers using single biological data, with 91.7% accuracy and 96.5% AUC. The most discriminative features (top 5%) for the classification include gut microbiota features (Lactobacillus, Haemophilus, and Prevotella), blood features (superoxide dismutase, monocyte-lymphocyte ratio, and neutrophil), and electroencephalogram features (nodal local efficiency, nodal efficiency, and nodal shortest path length in the temporal and frontal-parietal areas).The proposed integrated framework may be help in understanding the pathophysiology of schizophrenia and developing biomarkers for schizophrenia using multi-biological data.
更多查看译文
关键词
schizophrenia,classification,multi-biological,multi-classifier,multi-feature,multi-cross
AI 理解论文
溯源树
样例
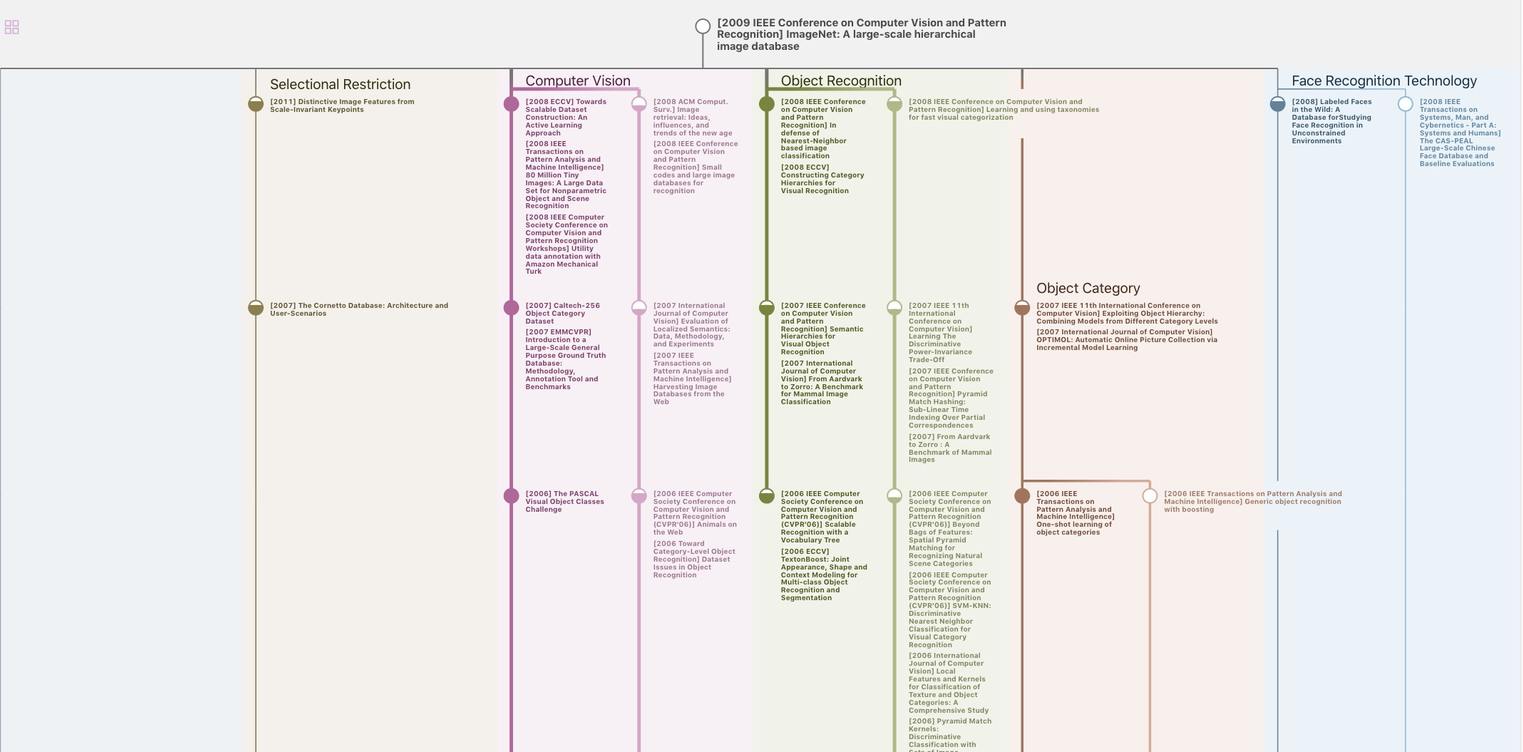
生成溯源树,研究论文发展脉络
Chat Paper
正在生成论文摘要