Conditional independence as an assessment of evidence integration processes
biorxiv(2023)
摘要
Intuitively, combining multiple sources of evidence should lead to more accurate decisions than considering single sources of evidence individually. In practice, however, the proper computation may be difficult, or may require additional data that are inaccessible. Here, based on the concept of conditional independence, we consider model-free expressions that can serve either as simplified recipes for integrating evidence or as benchmarks for characterizing evidence integration processes. Consider three events, A, B , and C . If A and B are conditionally independent with respect to C , then the probability that C occurs given that both A and B are known, P ( C | A, B ), can be easily calculated without the need to measure the full three-way dependency between A, B , and C . This simplified approach can be used in two general ways: to generate predictions by combining multiple (independent) sources of evidence, or to test whether separate sources of evidence are functionally independent of each other. These applications are demonstrated with four computer-simulated examples, which include detecting a disease based on repeated diagnostic testing, inferring biological age based on multiple biomarkers of aging, discriminating two spatial locations based on multiple cue stimuli (multisensory integration), and examining how behavioral performance in a visual search task depends on selection histories. The results indicate that this methodology could be useful for analyzing data in a wide range of experimental systems and conditions.
### Competing Interest Statement
The authors have declared no competing interest.
更多查看译文
AI 理解论文
溯源树
样例
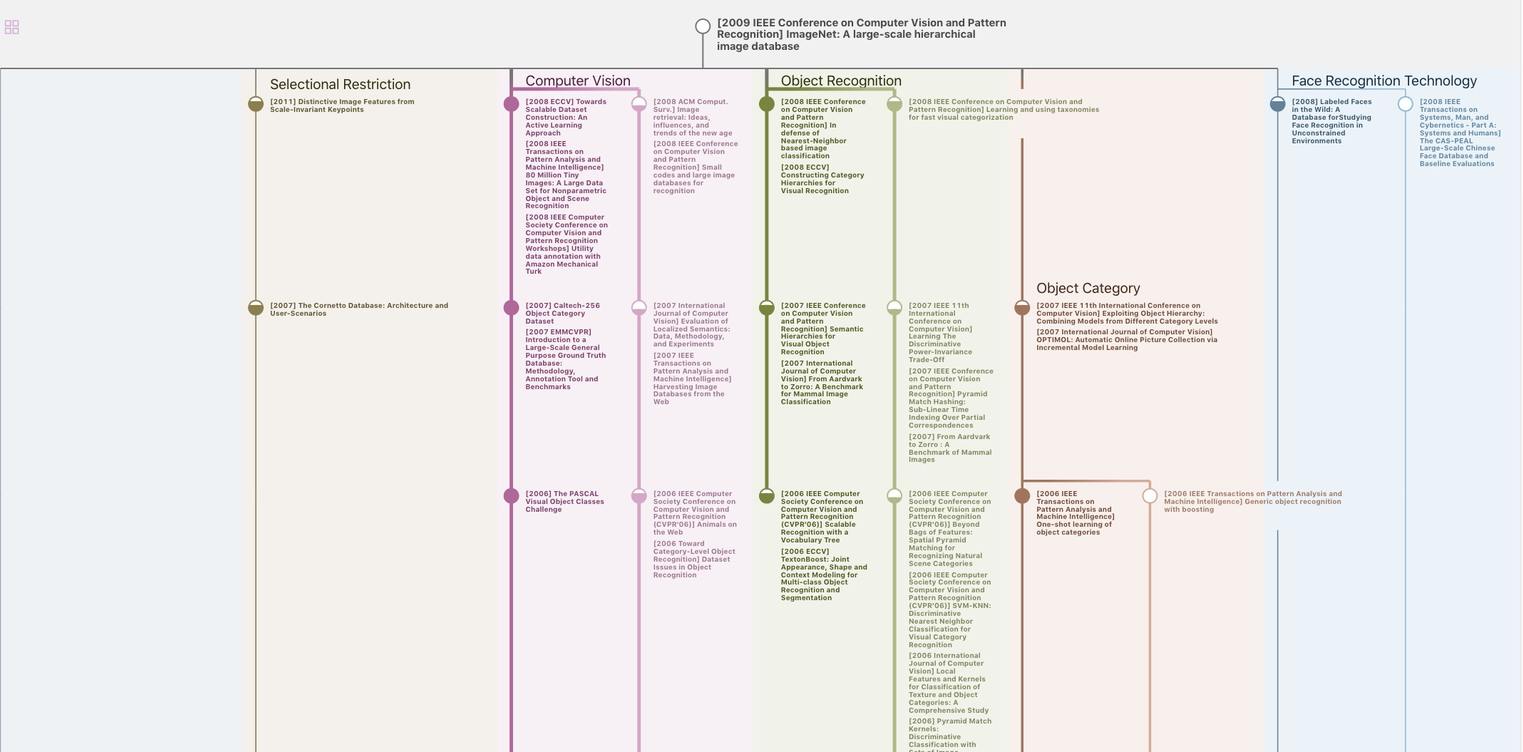
生成溯源树,研究论文发展脉络
Chat Paper
正在生成论文摘要