Automatic Image Classification Using Neural Networks Increases Accuracy for Allergenic Pollen Monitoring
Research Square (Research Square)(2020)
摘要
Abstract Monitoring of airborne pollen concentrations provides an important source of information for the globally increasing number of hay fever patients. Airborne pollen are traditionally counted under the microscope, but with the latest developments in image recognition methods, automating this process has become feasible. A challenge that persists, however, is that many pollen grains cannot be distinguished beyond the genus or family level using a microscope. Here, we assess the use of a Convolutional Neural Network (CNN) to increase taxonomic accuracy for airborne pollen. As a case study we use the nettle family (Urticaceae), which contains two main genera (Urtica and Parietaria) common in European landscapes which pollen cannot be separated by trained specialists. While pollen from Urtica species have very low allergenic relevance, those from several species of Parietaria are severely allergenic. We collect pollen from both fresh as well as from herbarium specimens and use these to train the CNN model VGG16. The model shows that Urticaceae pollen can be distinguished with 98.3% accuracy. We then apply our model on Urticaceae pollen collected from aerobiological samples and show that the genera can be confidently distinguished, despite the more challenging input images that are often overlain by debris. Our method can also be applied to other pollen families in the future and will thus help to make allergenic pollen monitoring more specific.
更多查看译文
关键词
neural networks,neural networks increases accuracy,classification
AI 理解论文
溯源树
样例
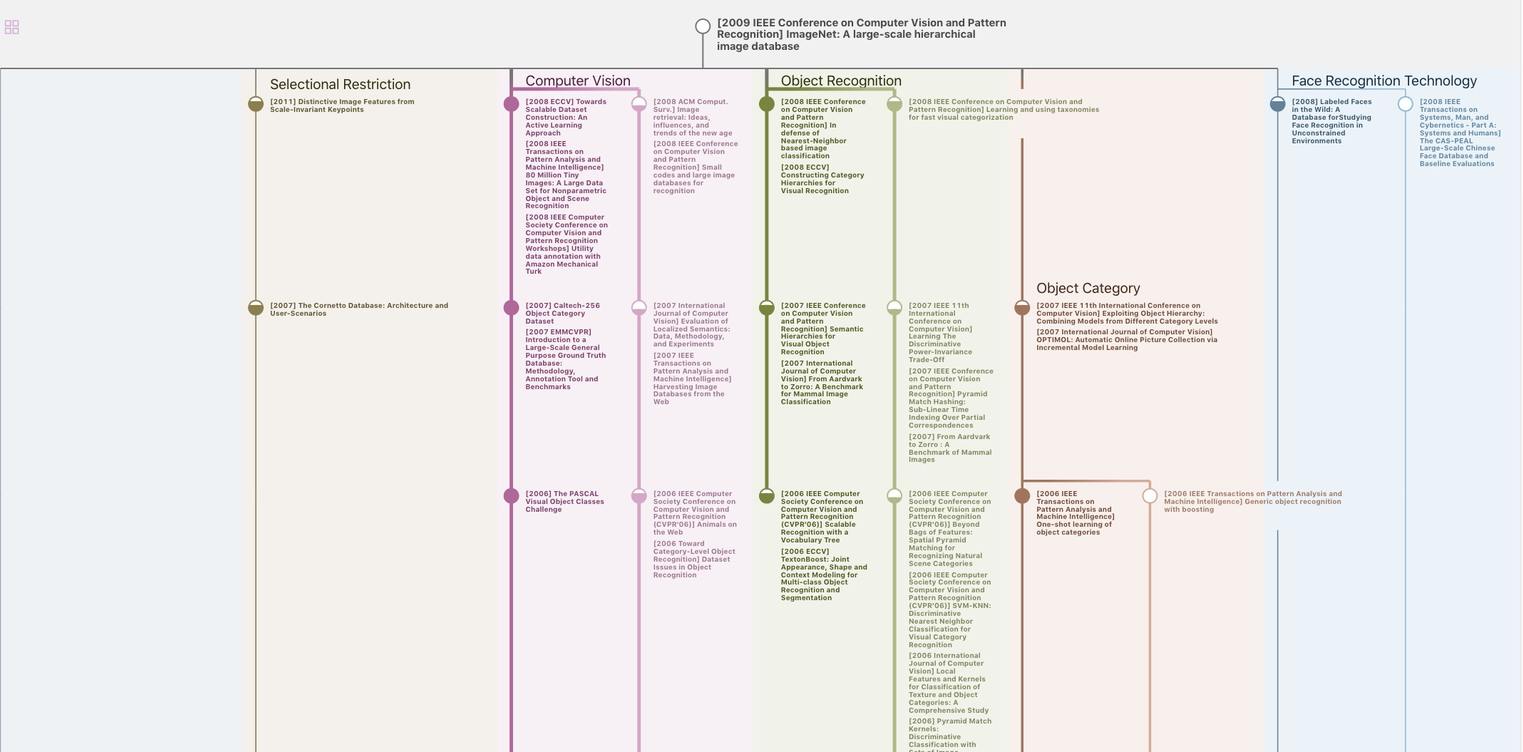
生成溯源树,研究论文发展脉络
Chat Paper
正在生成论文摘要