RNAseqCovarImpute: a multiple imputation procedure that outperforms complete case and single imputation differential expression analysis
bioRxiv (Cold Spring Harbor Laboratory)(2023)
摘要
Missing covariate data is a common problem that has not been addressed in observational studies of gene expression. Here we present a multiple imputation (MI) method that accommodates high dimensional transcriptomic data by binning genes, creating separate MI datasets and differential expression models within each bin, and pooling results with Rubin’s rules. Simulation studies using real and synthetic data show that this method outperforms complete case and single imputation analyses at uncovering true positive differentially expressed genes, limiting false discovery rates, and minimizing bias. This method is easily implemented via an R package, “RNAseqCovarImpute” that integrates with the limma-voom pipeline.
### Competing Interest Statement
The authors have declared no competing interest.
更多查看译文
关键词
multiple imputation procedure,single imputation,expression analysis,complete case
AI 理解论文
溯源树
样例
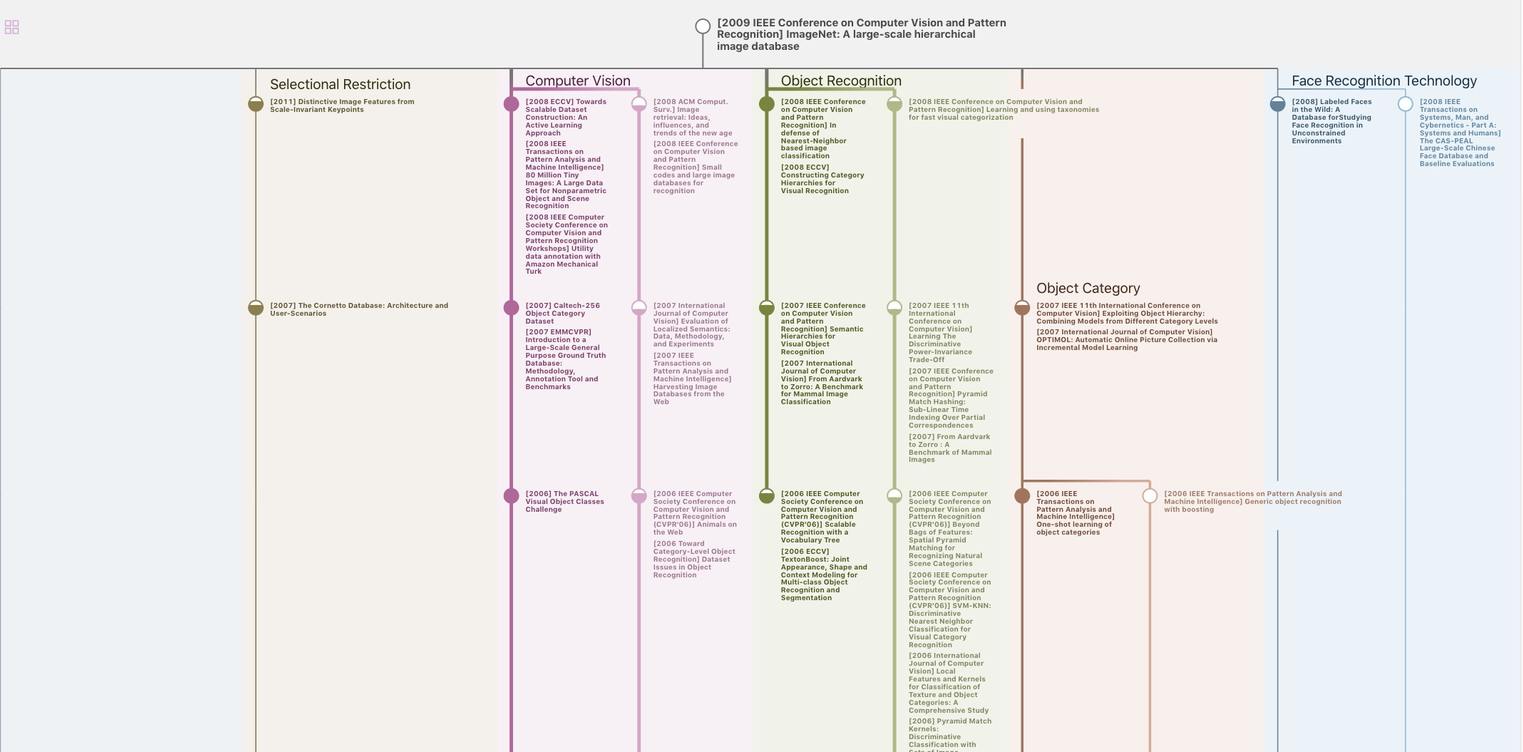
生成溯源树,研究论文发展脉络
Chat Paper
正在生成论文摘要