Drug Repositioning using Consilience of Knowledge Graph Completion Methods
bioRxiv (Cold Spring Harbor Laboratory)(2023)
摘要
While link prediction methods in knowledge graphs have been increasingly utilized to locate potential associations between compounds and diseases, they suffer from lack of sufficient evidence to prove why a drug and a disease may be indicated. This is especially true for knowledge graph embedding (KGE) based methods where a drug-disease indication is linked only by information gleaned from a vector representation. Complementary pathwalking algorithms can increase the confidence of drug repositioning candidates by traversing a knowledge graph. However, these methods heavily weigh the relatedness of drugs, through their targets, pharmacology or shared diseases. Furthermore, these methods rely on arbitrarily extracted paths as evidence of a compound to disease indication and lack the ability to make predictions on rare diseases. In this paper, we evaluate seven link prediction methods on a large biomedical knowledge graph on a drug repositioning task. We follow the principle of consilience, and combine the reasoning paths and predictions provided by probabilistic case-based reasoning (probCBR) with those of a KGE method, TransE, to identify putative drug repositioning indications. Finally, we highlight the utility of our approach through two potential repurposing indications.
### Competing Interest Statement
The authors have declared no competing interest.
更多查看译文
关键词
drug repositioning,knowledge,completion,graph
AI 理解论文
溯源树
样例
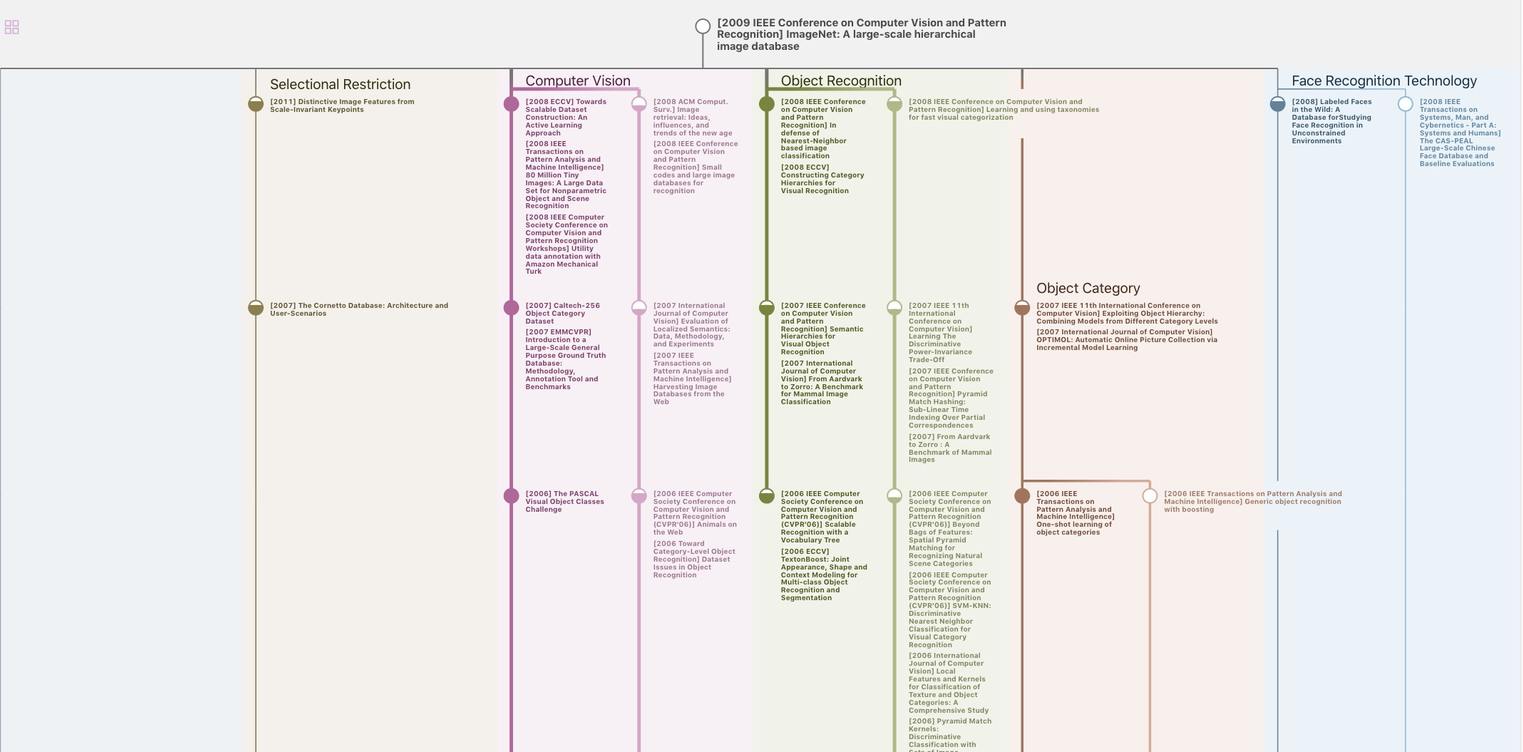
生成溯源树,研究论文发展脉络
Chat Paper
正在生成论文摘要