Heterogeneity-Preserving Discriminative Feature Selection for Subtype Discovery
bioRxiv (Cold Spring Harbor Laboratory)(2023)
摘要
Subtype discovery is crucial to disease diagnosis and targeted therapy as each cell or patient can display a wide range of responses to specific treatments. It is also vital to investigate the heterogeneity, or diverse variety, of disease states to better understand pathological processes. While there are many new opportunities for subtype discovery due to the explosion of single-cell data (single-cell RNA-seq, proteomic, and imaging datasets), selecting features for disease subtyping from high-dimensional datasets is challenging. Feature selection is the process of reducing the number of features for downstream computational analyses. Most feature selection algorithms are focused on identifying features to aid in the classification of known disease phenotypes. However, this effectively eliminates heterogeneous features and collapses the disease feature space, hindering valuable subtyping. Our work aimed to identify feature sets that preserve heterogeneity while maintaining the discrimination of known disease states. We initially applied a data-driven approach to determine the statistical characteristics of features essential in preserving heterogeneity by combining feature clustering and deep metric learning. This analysis revealed that features with a significant difference in interquartile range (IQR) between classes could contain critical subtype information. Utilizing this knowledge, we developed a statistical method, PHet (Preserving Heterogeneity), that performs recurrent sub-sampling differential analysis of IQR between classes to identify a minimal set of heterogeneity-preserving features while maximizing the quality of subtype clustering. Using public datasets of microarray and single-cell RNA-seq, we demonstrated that PHet effectively identifies disease subtypes and outperforms the previous outlier-based methods. In summary, our study provides a novel feature selection method for disease subtyping, which will enable not only personalized medicine but also a detailed understanding of the underlying heterogeneous mechanisms of diseases.
### Competing Interest Statement
The authors have declared no competing interest.
更多查看译文
关键词
subtype discovery,selection,feature,heterogeneity-preserving
AI 理解论文
溯源树
样例
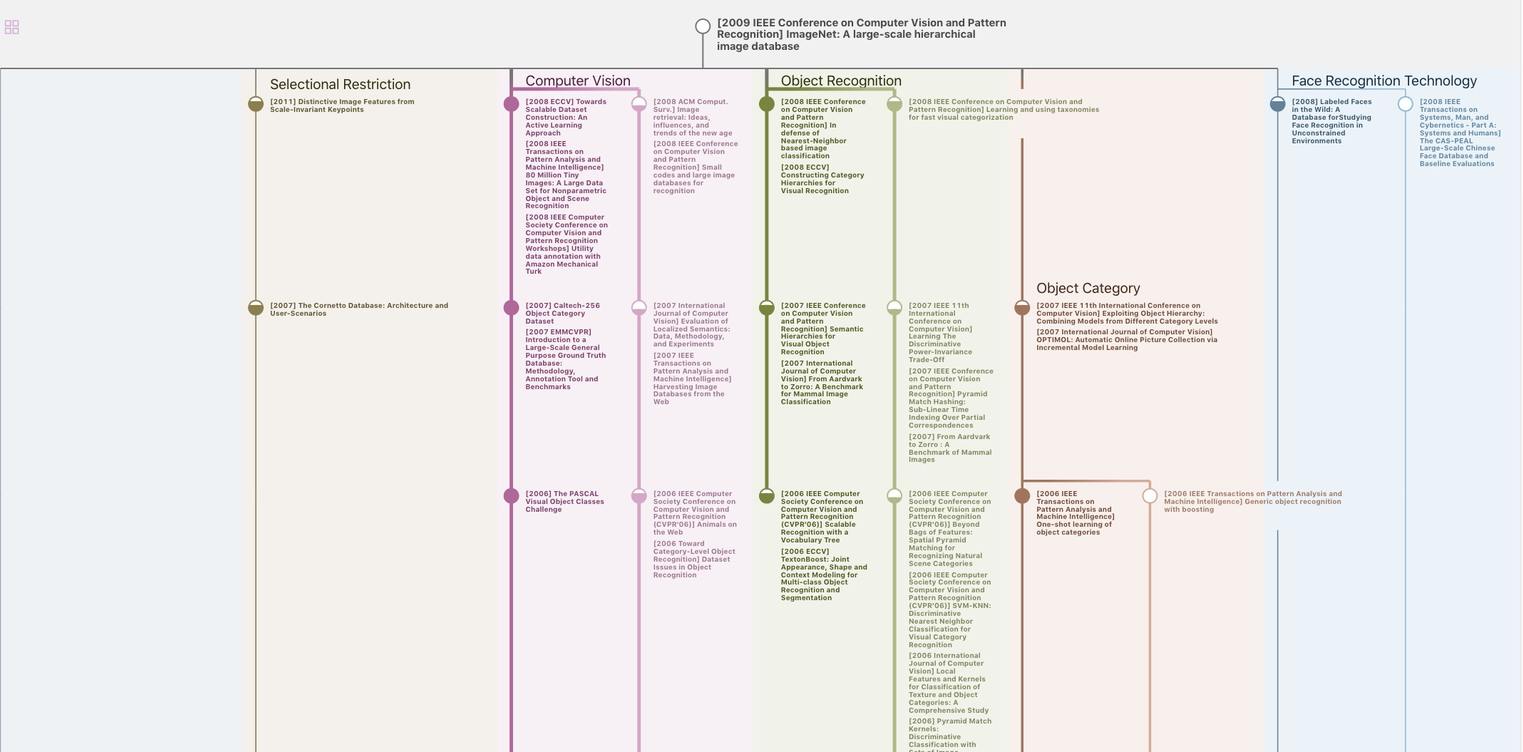
生成溯源树,研究论文发展脉络
Chat Paper
正在生成论文摘要