Creation of predictive cardiac excitation models at the tissue scale with machine learning in augmented state space
biorxiv(2023)
摘要
When creating in-silico models of excitable media, often only one spatio-temporal variable can be measured easily. This may for instance be optical voltage mapping data of the electrical waves in cardiac muscle tissue controlling the heart beat. We use exponential moving averages and gradients computed using the least-squares method to extract additional states from this one variable to span a sparse discretised 3D state space. To this augmented state space, we fit a s imple polynomial model to predict the evolution of the one state variable. For optical voltage mapping data of human atrial myocyte monolayers stimulated using a stochastic burst pacing protocol, the surrogate model is able to describe the excitation of the system, as well as wave propagation.
### Competing Interest Statement
The authors have declared no competing interest.
更多查看译文
AI 理解论文
溯源树
样例
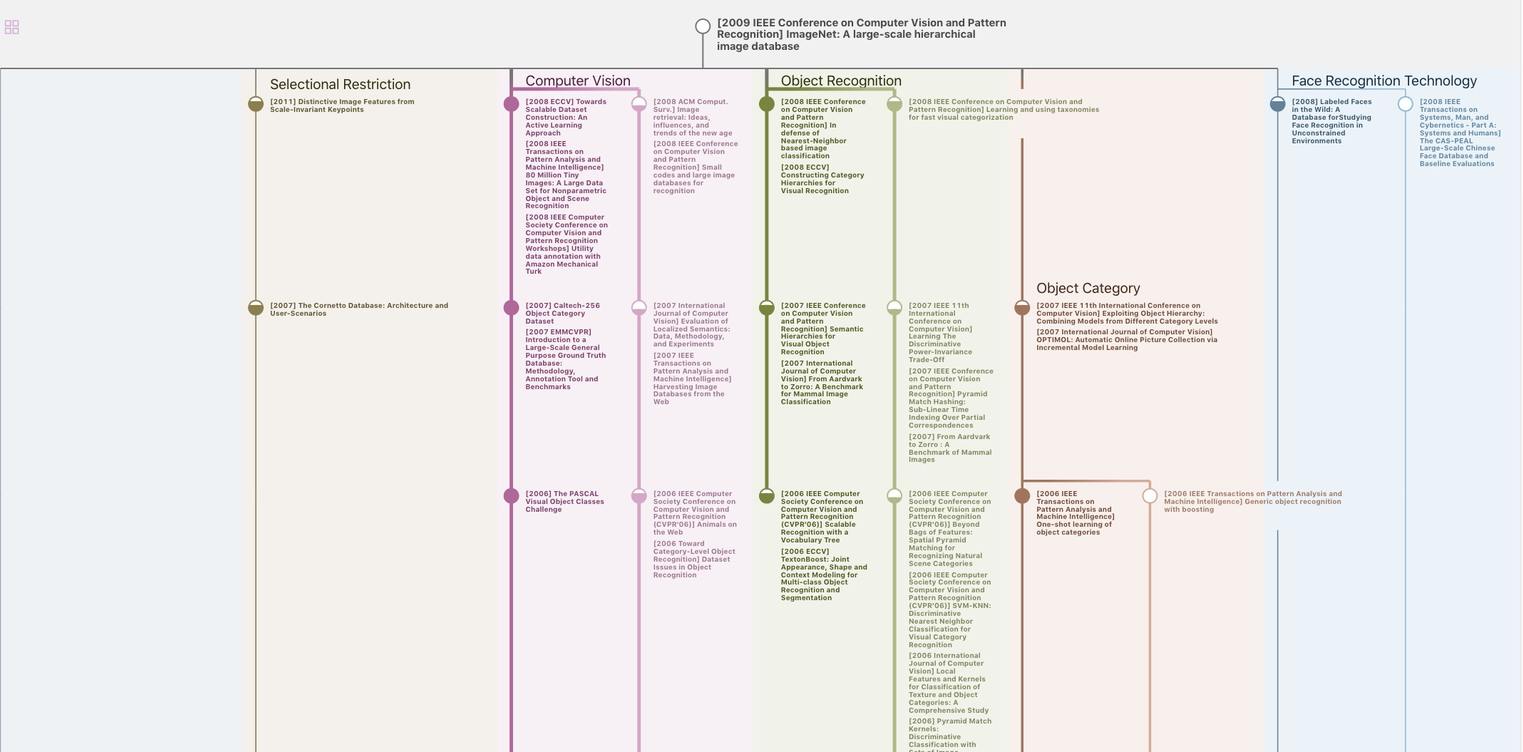
生成溯源树,研究论文发展脉络
Chat Paper
正在生成论文摘要