Discovering Biomarker Proteins and Peptides for Parkinson’s Disease Prognosis Prediction with Machine Learning and Interpretability Methods
biorxiv(2023)
摘要
Parkinson’s disease is a neurodegenerative disorder that affects millions of people worldwide, posing significant challenges for diagnosis and treatment. This study presents a machine learning pipeline for identifying candidate biomarker proteins and peptides from cerebrospinal fluid mass spectrometry (CSF-MS) tests in Parkinson’s disease patients. Our pipeline comprises two main stages: (1) model training using mutual information-based feature selection and five different machine learning regressors and (2) identification of candidate biomarkers by combining three types of interpretability methods. Our regression models demonstrated promising effectiveness in predicting the Movement Disorder Society-Unified Parkinson’s Disease Rating Scale (MDS-UPDRS) scores, with UPDRS-1 receiving the best predictions, followed by UPDRS-3 and UPDRS-2. Furthermore, our pipeline identified 11 proteins and peptides as potential biomarkers for Parkinson’s disease, excluding Levodopa usage which trivially has the most significant impact on the prognosis prediction. Comparisons with four additional pipelines confirmed the effectiveness of our approach in terms of both model performance and biomarker identification. In conclusion, our study presents a comprehensive machine learning pipeline that demonstrates effectiveness in predicting the severity of Parkinson’s disease using CSF-MS tests. Our approach also identifies potential biomarkers, which could aid in the development of new diagnostic tools and treatments for patients with Parkinson’s disease.
### Competing Interest Statement
The authors have declared no competing interest.
更多查看译文
关键词
disease prognosis prediction,parkinsons disease,biomarker,machine learning
AI 理解论文
溯源树
样例
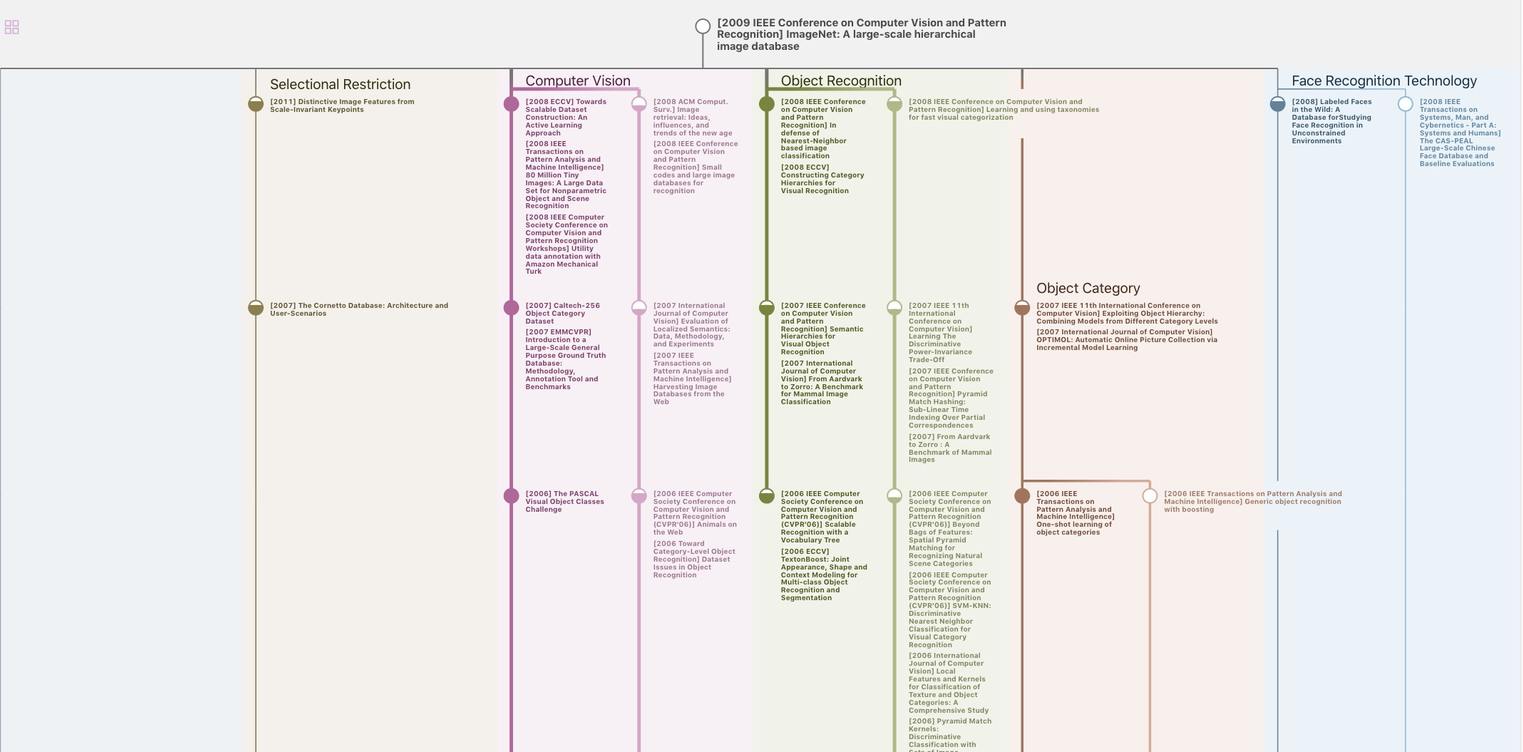
生成溯源树,研究论文发展脉络
Chat Paper
正在生成论文摘要