PENet: A Phenotype Encoding Network for Automatic Extraction and Representation of Morphological Discriminative Features
METHODS IN ECOLOGY AND EVOLUTION(2023)
摘要
Discriminative traits are important in biodiversity and macroevolution, but extracting and representing these features from huge natural history collections using traditional methods can be challenging and time-consuming. To fully utilize the collections and their associated metadata, it is urgent now to increase the efficiency of automatic feature extraction and sample retrieval. We developed a Phenotype Encoding Network (PENet), a deep learning-based model that combines hashing methods to automatically extract and encode discriminative features into hash codes. We tested the performance of PENet on six datasets, including a newly constructed beetle dataset with six subfamilies and 6566 images, which covers more than 60% of the genera in the family Scarabaeidae. PENet showed excellent performance in feature extraction and image retrieval. Two visualization methods, t-SNE, and Grad-CAM, were used to evaluate the representation ability of the hash codes. Further, by using the hash codes generated from PENet, a phenetic distance tree was constructed based on the beetle dataset. The result indicated the hash codes could reveal the phenetic distances and relationships among categories to a certain extent. PENet provides an automatic way to extract and represent morphological discriminative features with higher efficiency, and the generated hash codes serve as a low-dimensional carrier of discriminative features and phenotypic distance information, allowing for broader applications in systematics and ecology. ### Competing Interest Statement The authors have declared no competing interest.
更多查看译文
关键词
deep learning,discriminative features,encoding,feature-based retrieval,hash
AI 理解论文
溯源树
样例
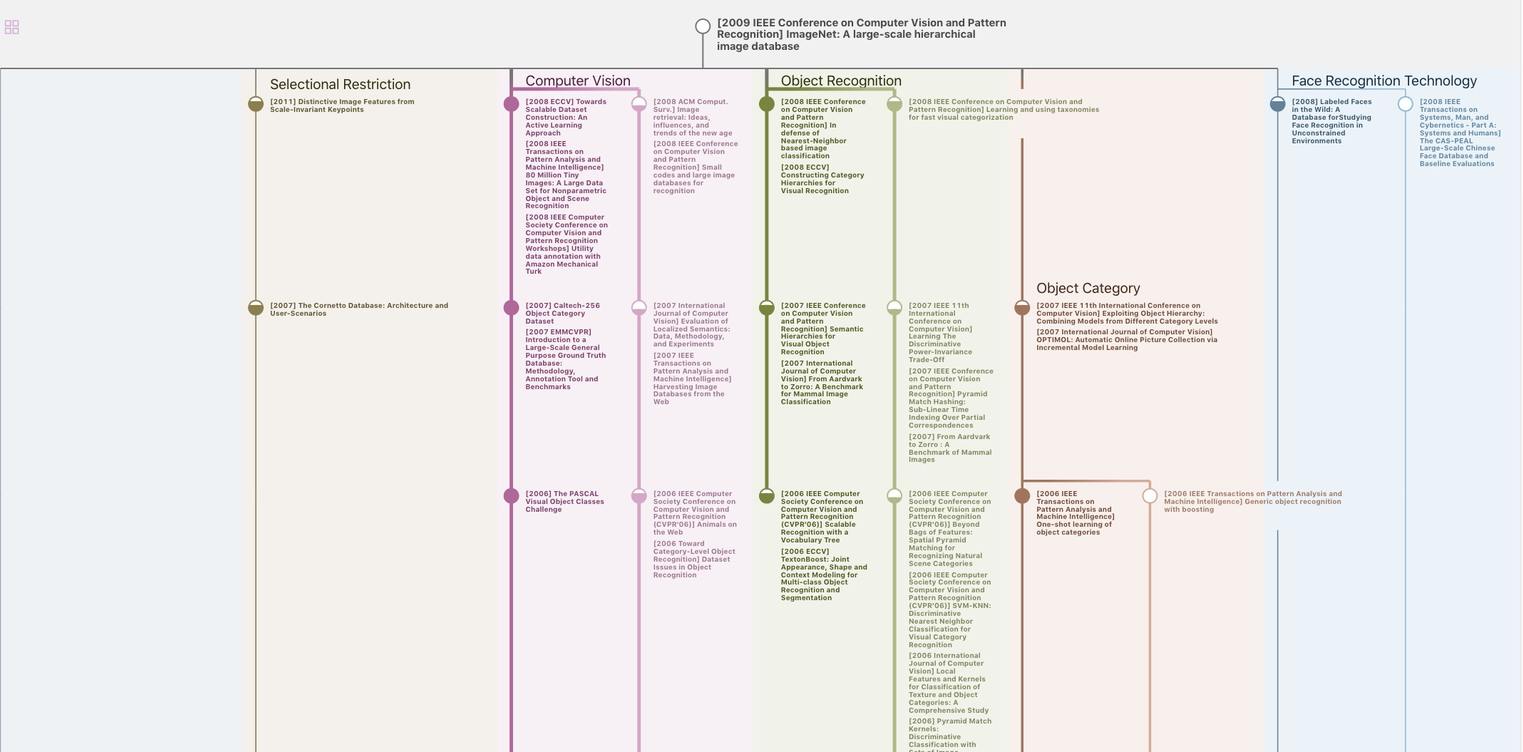
生成溯源树,研究论文发展脉络
Chat Paper
正在生成论文摘要