A hierarchical Bayesian brain parcellation framework for fusion of functional imaging datasets
bioRxiv (Cold Spring Harbor Laboratory)(2023)
摘要
One important barrier in the development of complex models of human brain organization is the lack of a large and comprehensive task-based neuro-imaging dataset. Therefore, current atlases of functional brain organization are mainly based on single and homogeneous resting-state datasets. Here, we propose a hierarchical Bayesian framework that can learn a probabilistically defined brain parcellation across numerous task-based and resting-state datasets, exploiting their combined strengths. The framework is partitioned into a spatial arrangement model that defines the probability of a specific individual brain parcellation, and a set of dataset-specific emission models that defines the probability of the observed data given the individual brain organization. We show that the framework optimally combines information from different datasets to achieve a new population-based atlas of the human cerebellum. Furthermore, we demonstrate that, using only 10 min of individual data, the framework is able to generate individual brain parcellations that outperform group atlases.
### Competing Interest Statement
The authors have declared no competing interest.
更多查看译文
关键词
imaging,brain,fusion
AI 理解论文
溯源树
样例
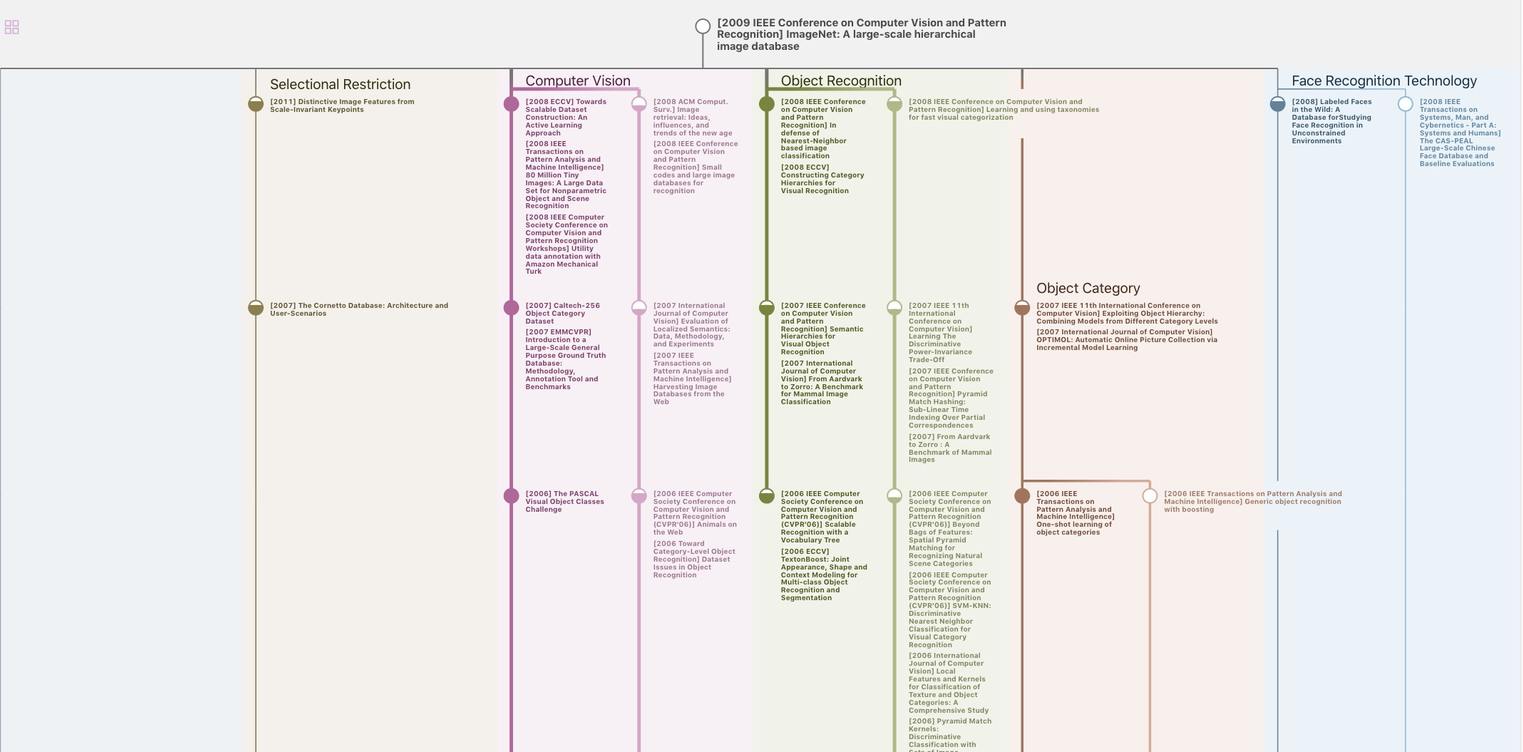
生成溯源树,研究论文发展脉络
Chat Paper
正在生成论文摘要