Boosting Single-Cell RNA Sequencing Analysis with Simple Neural Attention
bioRxiv : the preprint server for biology(2023)
摘要
A limitation of current deep learning (DL) approaches for single-cell RNA sequencing (scRNAseq) analysis is the lack of interpretability. Moreover, existing pipelines are designed and trained for specific tasks used disjointly for different stages of analysis. We present scANNA, a novel interpretable DL model for scR-NAseq studies that leverages neural attention to learn gene associations. After training, the learned gene importance (interpretability) is used to perform downstream analyses (e.g., global marker selection and cell-type classification) without retraining. ScANNA’s performance is comparable to or better than state-of-the-art methods designed and trained for specific standard scRNAseq analyses even though scANNA was not trained for these tasks explicitly. ScANNA enables researchers to discover meaningful results without extensive prior knowledge or training separate task-specific models, saving time and enhancing scRNAseq analyses.
### Competing Interest Statement
E.J.F is on the Scientific Advisory Board for ResistanceBio and Viosera Therapeutics, and a paid consultant for Mestag Therapeutics and Merck. Other authors declare no competing interests.
更多查看译文
关键词
rna,attention,single-cell
AI 理解论文
溯源树
样例
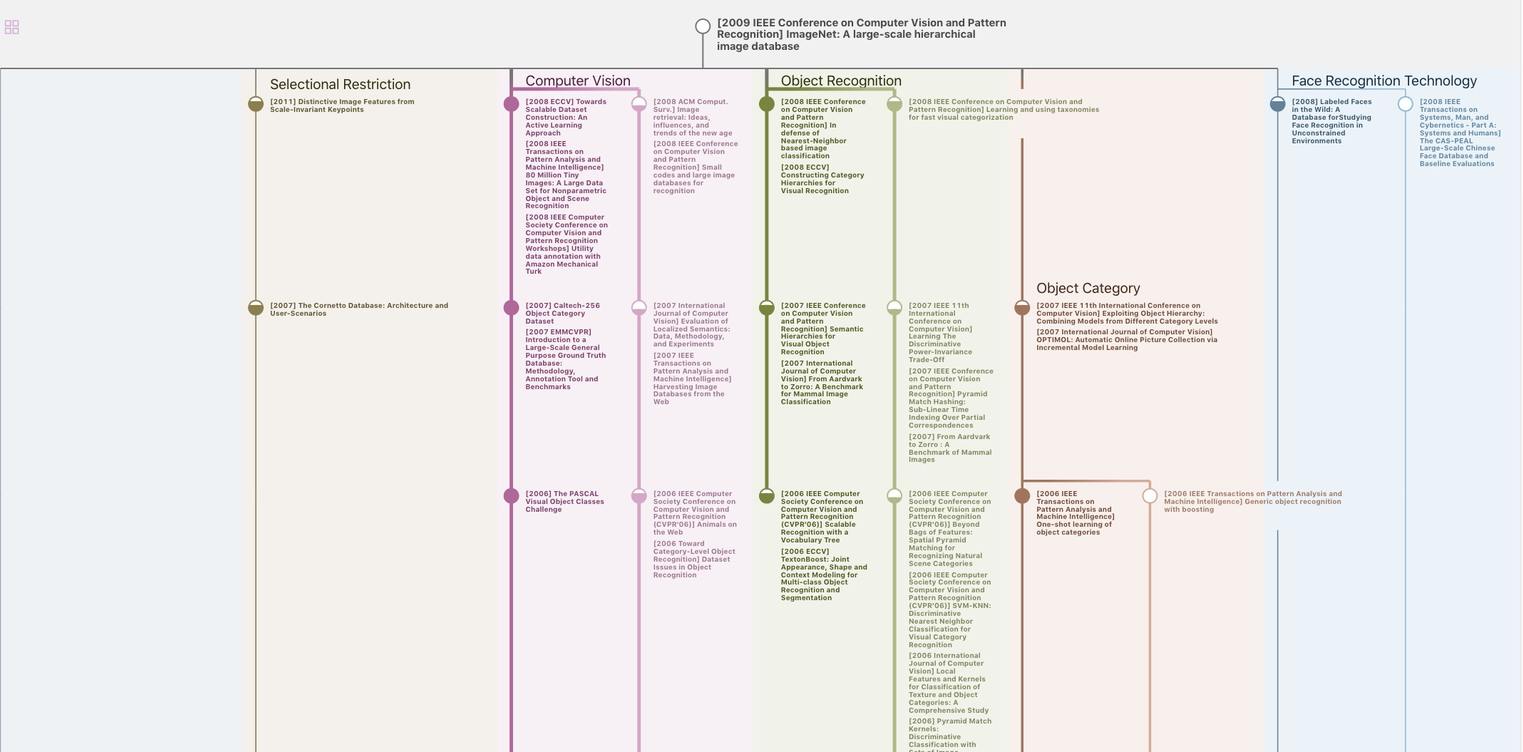
生成溯源树,研究论文发展脉络
Chat Paper
正在生成论文摘要