Cognitive Model Discovery via Disentangled RNNs.
NeurIPS(2023)
Abstract
Computational cognitive models are a fundamental tool in behavioral neuroscience. They instantiate in software precise hypotheses about the cognitive mechanisms underlying a particular behavior. Constructing these models is typically a difficult iterative process that requires both inspiration from the literature and the creativity of an individual researcher. Here, we adopt an alternative approach to learn parsimonious cognitive models directly from data. We fit behavior data using a recurrent neural network that is penalized for carrying information forward in time, leading to sparse, interpretable representations and dynamics. When fitting synthetic behavioral data from known cognitive models, our method recovers the underlying form of those models. When fit to laboratory data from rats performing a reward learning task, our method recovers simple and interpretable models that make testable predictions about neural mechanisms.
### Competing Interest Statement
The authors have declared no competing interest.
MoreTranslated text
AI Read Science
Must-Reading Tree
Example
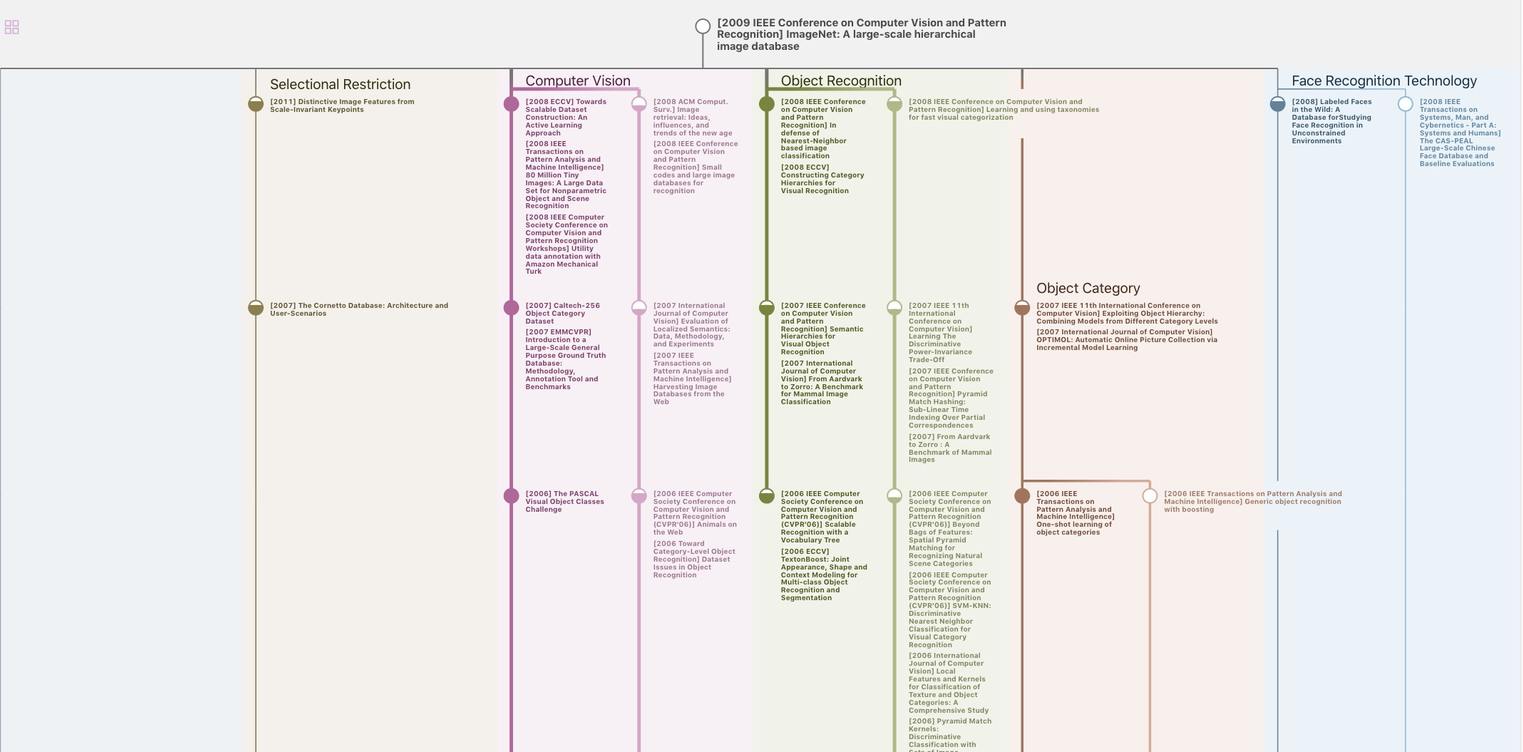
Generate MRT to find the research sequence of this paper
Chat Paper
Summary is being generated by the instructions you defined