Mixed structure- and sequence-based approach for protein graph neural networks with application to antibody developability prediction
bioRxiv (Cold Spring Harbor Laboratory)(2023)
摘要
There are hundreds of thousands of known proteins but significantly fewer unique protein folds. Furthermore, proteins often have conserved and even repeating geometric patterns, which can be captured by models of protein structure and function. In this work, we use Delaunay tessellations and α-shapes, which capture these conserved geometric patterns, to define graph adjacency in Graph Convolutional Networks (GCN). We demonstrate the utility of the resulting GCN model on antibody developability prediction. Compared to the frequently used graph adjacencies based on k-nearest-neighbors or a fixed cutoff radius, the Delaunay tessellation and α-shapes better capture residue-specific interactions at a lower computational cost for a given system size. The resulting models achieve state-of-the-art performance on an antibody developability prediction task. Finally, we propose an extension of the model which does not require known or predicted structures but uses an "archetypical" antibody structure to infer likely contacts.
### Competing Interest Statement
Genetech Inc.
更多查看译文
关键词
protein graph,neural networks,sequence-based
AI 理解论文
溯源树
样例
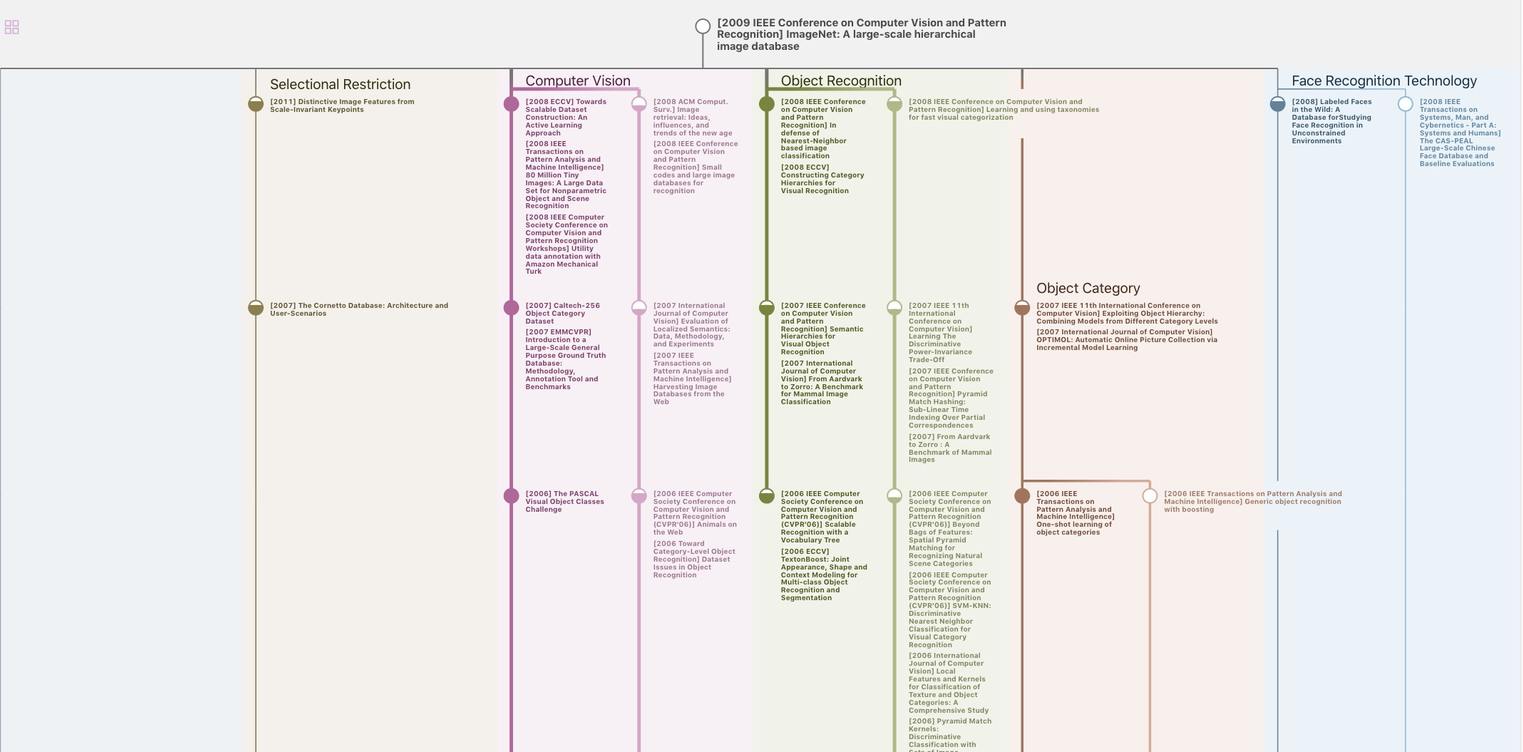
生成溯源树,研究论文发展脉络
Chat Paper
正在生成论文摘要