Recurrent neural networks that learn multi-step visual routines with reinforcement learning
bioRxiv (Cold Spring Harbor Laboratory)(2024)
摘要
Many problems can be decomposed into series of subproblems that are solved sequentially. When the subproblems are solved, relevant intermediate results have to be stored and propagated from one subproblem to the next, until the overarching goal has been completed. The same applies for visual tasks that can be decomposed into sequences of elemental visual operations: experimental evidence suggests that for visual tasks, intermediate results are stored in working memory as an enhancement of neural activity in the visual cortex. The focus of enhanced activity is then available for subsequent subroutines to act upon. However, it remains unknown how those dynamics can emerge in neural networks that are trained with only rewards, as animals are. Here, we propose a new recurrent architecture capable of solving composite visual tasks in a reinforcement learning context. We trained neural networks on three visual tasks for which electrophysiological recordings of monkeys' visual cortex are available and updated weights following RELEARNN, a biologically plausible four-factor Hebbian learning rule, which is local both in time and space. The networks learned an abstract, general rule and selected the appropriate sequence of elemental operations, solely based on the characteristics of the visual stimuli and the reward structure. The activity of the units of the neural network resembled the activity of neurons in the visual cortex of monkeys solving the same tasks. Relevant information that needed to be exchanged between subroutines was maintained as a focus of enhanced activity and passed on to the subsequent subroutines. Our results demonstrate how a biologically plausible learning rule can train a recurrent neural network on multistep visual tasks.
### Competing Interest Statement
The authors have declared no competing interest.
更多查看译文
关键词
recurrent neural networks,reinforcement learning,neural networks,multi-step
AI 理解论文
溯源树
样例
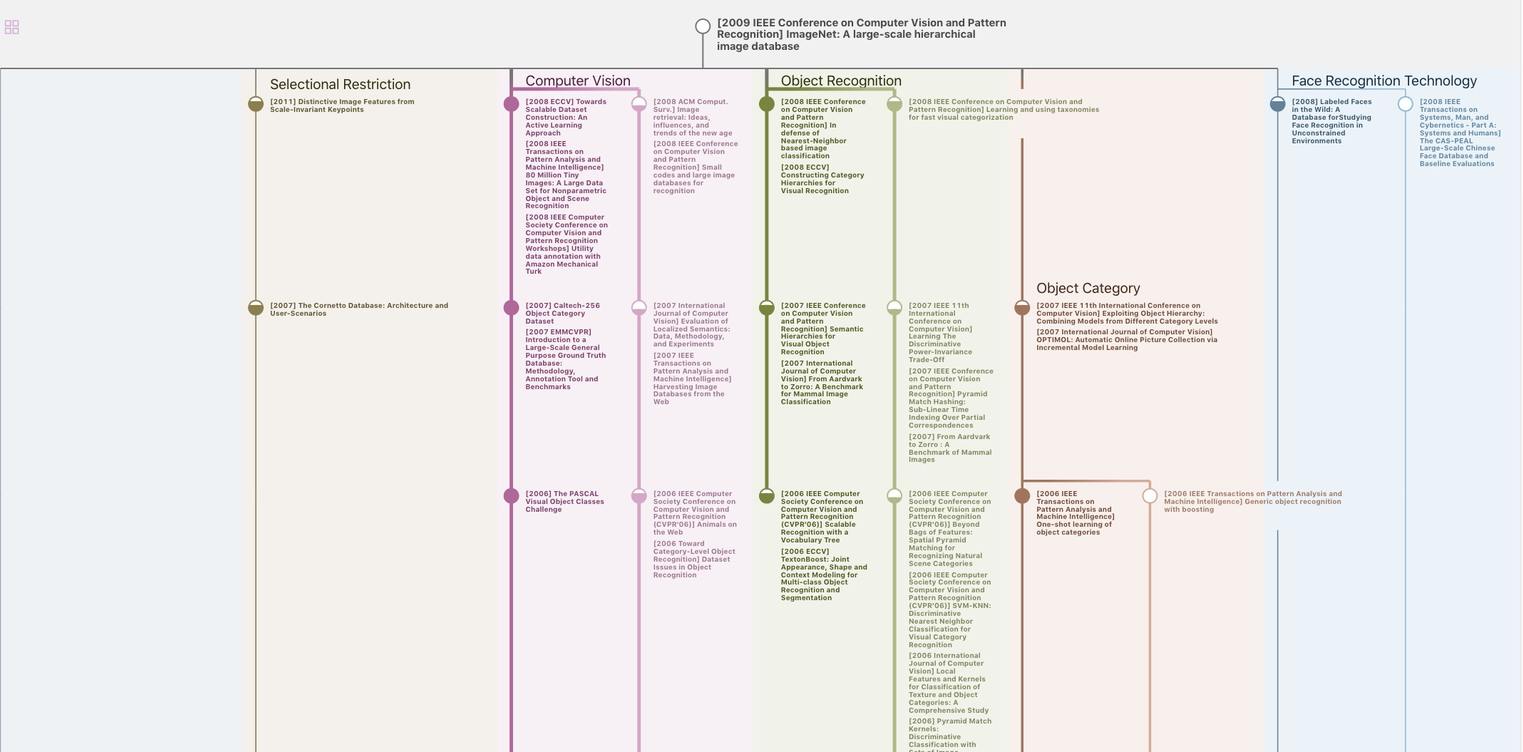
生成溯源树,研究论文发展脉络
Chat Paper
正在生成论文摘要