New genome scale network modeling and mining workflow for detecting metabolic changes induced by exposure to chemicals
bioRxiv (Cold Spring Harbor Laboratory)(2023)
摘要
The growing abundance of in vitro omics data, coupled with the necessity to reduce animal testing in the safety assessment of chemical compounds and even eliminate it in the evaluation of cosmetics, highlights the need for abundant computational methodologies. Data from omics technologies allow the exploration of a wide range of biological processes, therefore providing a better understanding of mechanisms of action (MoA) related to chemical exposure in biological systems. However, the analysis of these large datasets remains difficult due to the complexity of modulations spanning multiple biological processes. To address this, we propose a new computational workflow that combines knowledge on endogenous metabolism from a genome scale metabolic network (GSMN) and in vitro transcriptomics data with the aim of better identifying the metabolic MoA (mMoA) of chemicals. Our workflow proceeds in three main steps. The first step consists of building cell condition-specific models representing the metabolic impact of each exposure condition while taking into account the diversity of possible optimal solutions with a partial enumeration algorithm. In a second step, based on these enumerations, two conditions can be compared by extracting differentially activated reactions (DARs) between the two sets of enumerated possible condition-specific models. Finally, in the third step, DARs are grouped into clusters of functionally interconnected metabolic reactions using the distance-based clustering and subnetwork extraction method. The first part of the workflow was exemplified on eight molecules selected for their known human hepatotoxic outcomes associated with specific MoAs well described in the literature and for which we retrieved primary human hepatocytes (PHH) transcriptomic data in Open TG-GATEs. Then, we applied this new workflow to model and visualize associated mMoA for two of these eight molecules (amiodarone and valproic acid). Despite large disparities in transcriptomic effects for these two chemicals, i.e., two differentially expressed genes (DEGs) for amiodarone vs 5709 DEGs for valproic acid, our results well fitted evidence from the literature regarding known MoA. Beyond these confirmations, the workflow highlighted potential other unexplored mMoA.
### Competing Interest Statement
The authors have declared no competing interest.
更多查看译文
关键词
metabolic changes,mining workflow
AI 理解论文
溯源树
样例
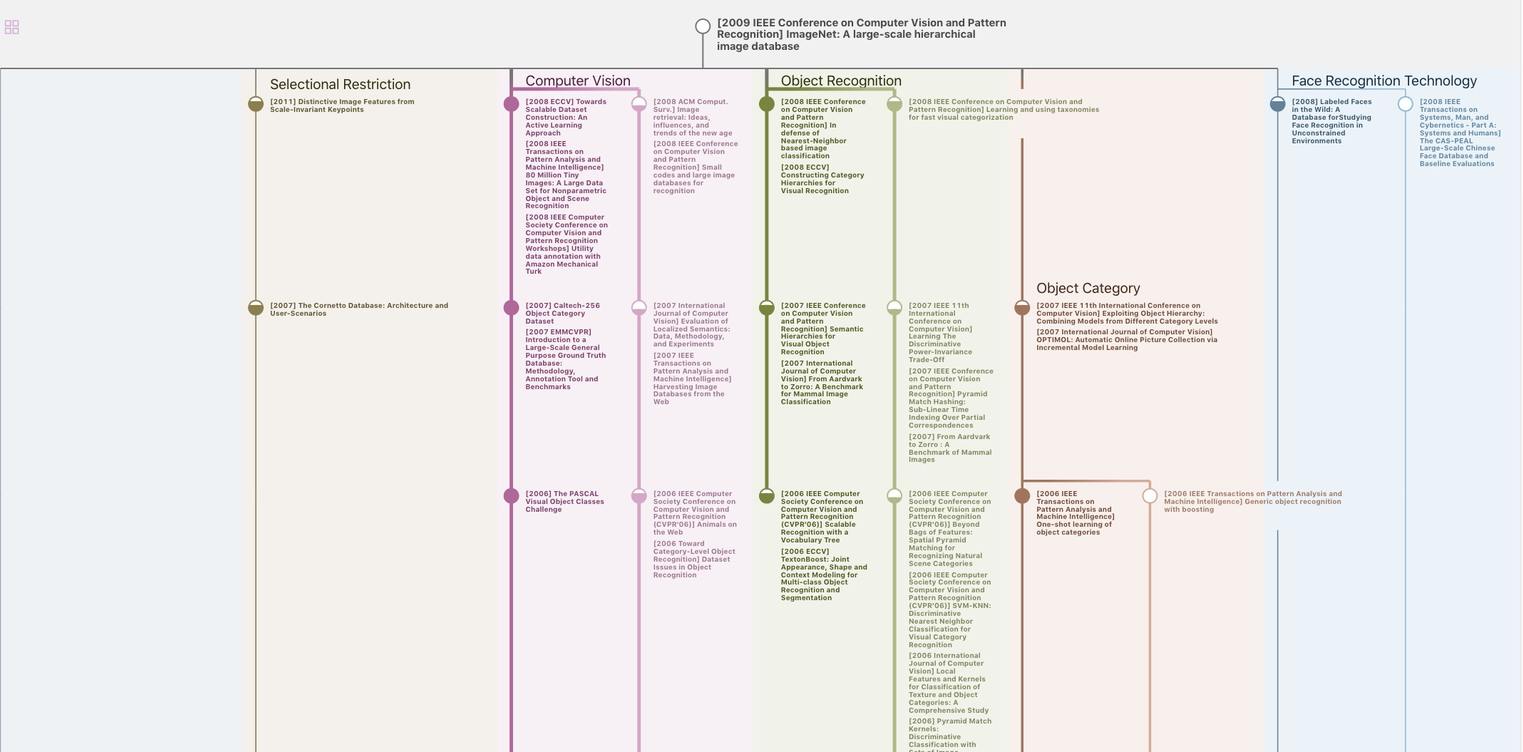
生成溯源树,研究论文发展脉络
Chat Paper
正在生成论文摘要