Time Series Analysis of Hemorrhagic Fever with Renal Syndrome in Mainland China by using XGBoost Forecasting Model
Research Square (Research Square)(2020)
摘要
Abstract Background: Hemorrhagic fever with renal syndrome is still attracting public attention because of its outbreak in various cities in China. It is one of the effective preventive measures to predict the peak incidence rate in the future based on the past incidence data, and implement targeted actions. In this study, we propose a multi-step prediction strategy based on XGBoost for hemorrhagic fever with renal syndrome as an extension of the one-step prediction model. Moreover, the fitting and prediction accuracy of XGBoost model will be compared with seasonal ARIMA model by different evaluation indicators. Methods: We collected monthly hemorrhagic fever with renal syndrome incidence data from 2004 to 2018 in mainland China .The part from 2004 to 2017 was divided as training set to establish the seasonal ARIMA model and XGBoost model. The rest 2018 data was used to test the prediction outcomes. In multi-step forecasting XGBoost model, one-hot encoding was used to handle seasonal features. Furthermore, series of evaluation index(MAE,MPE,MAPE,RMSE,MASE, ACF1, Theil’s U) were performed to evaluate the accuracy of multi-step forecast XGBoost model.Results: There were totally 200237 HFRS cases in China from 2004 to 2018. A slightly long-term downward trend and obvious bimodal peak seasonal character were identified in the original time series. According to the minimum CAIC value, the optimal ARIMA (3,1,0) × (1,1,0)12 model is selected. the ME , MAE, MPE, MAPE, MASE of XGBoost were higher than ARIMA model in the fitting part, whereas the RMSE of XGBoost was lower. The evaluation indicators (MAE, MPE, MAPE, RMSE , MASE) of the one-step prediction and multi-step prediction XGBoost model are all notably lower than the ARIMA model in prediction performance. Conclusions: The multi-step prediction XGBoost model showed a much better prediction accuracy and model stability in HFRS disease . In general, compared to the seasonal ARIMA model, the XGBoost model performs better when predicting complicated and non-linear data like Hemorrhagic fever with renal syndrome. Additionally Multi-step prediction models are more practical than one-step prediction in forecasting infectious diseases.
更多查看译文
关键词
hemorrhagic fever,renal syndrome
AI 理解论文
溯源树
样例
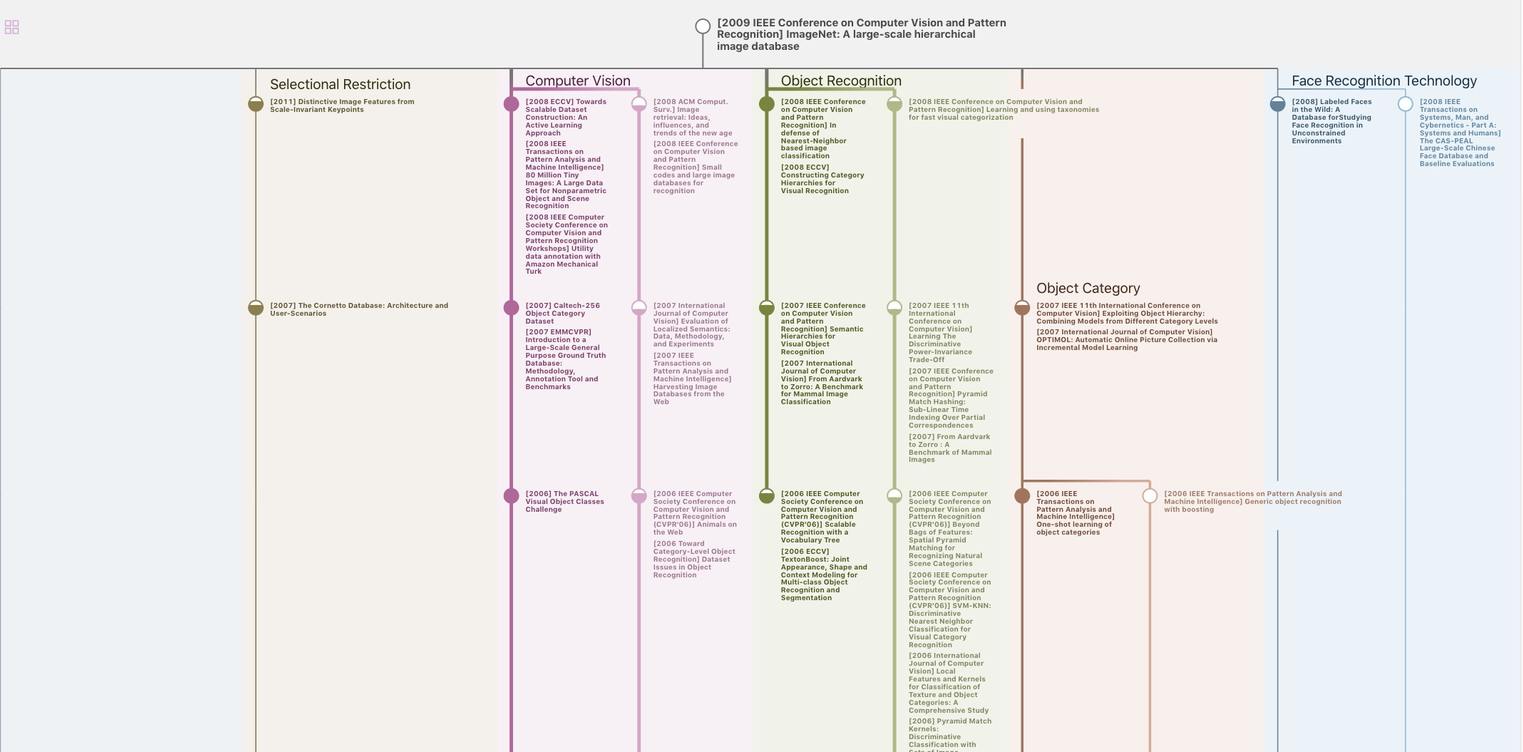
生成溯源树,研究论文发展脉络
Chat Paper
正在生成论文摘要