Machine Learning and Integrative Analysis Reveals Stemness Index-associated Signature in Acute Myeloid Leukemia
Research Square (Research Square)(2020)
摘要
Abstract Background: The rapidly growing research in leukemia has provided unequivocal proof that cancer stem cells exist in acute myeloid leukemia. However, the mechanisms of the growth and self-renewal of acute myeloid leukemia stem cells (LSCs), which play an important role in the development of AML, were still elusive. Methods: We first used a trained stemness index model based on a recently described machine learning method one-class logistic regression (OCLR) to score AML samples. We then obtained the mRNA expression based-index (mRNAsi), DNA methylation based-index (mDNAsi), and epigenetic regulation based-index (EREG-mRNAsi). With the acquired three stemness indices, we investigated the relationship between the stemness index and the tumor immune microenvironment/somatic mutation, clinical characteristics of AML patients. Furthermore, a weighted gene co-expression network analysis (WGCNA) and protein-protein interaction (PPI) network were performed to obtain key genes associated with the stemness index. Bedsides, GO and KEGG enrichment analysis was performed to explore the biological functions. Finally, we validated the key genes by applying it to the Oncomine and GEO datasets.Result: The results suggested that EREG-mRNAsi might accurately predict overall survival (OS) in AML patients from TCGA, while the mRNAsi and mDNAsi was not significantly statistically associated with the OS. Furthermore, the mRNAsi was significantly correlated with the clinical characteristics of the FAB category, age, gender, immune score, WT1, and CEBPA mutation. Additionally, the EREG-mRNAsi was significantly associated with the cytogenetics risk category, TP53 mutation. The mDNAsi was significantly associated with the FAB-category, NPM1, IDH1, IDH2, and DNMT3A mutation. Several key genes related to the stemness index of AML were indicated to be possible and promising therapeutic targets for inhibiting LSCs in AML.Conclusions: Our research based on machine learning and WGCNA analysis revealed a set of key genes contributed to the stemness index of AML. These genes may become therapeutic targets to inhibit the LSCs of AML in the future.
更多查看译文
关键词
acute myeloid leukemia,machine learning,integrative analysis,index-associated
AI 理解论文
溯源树
样例
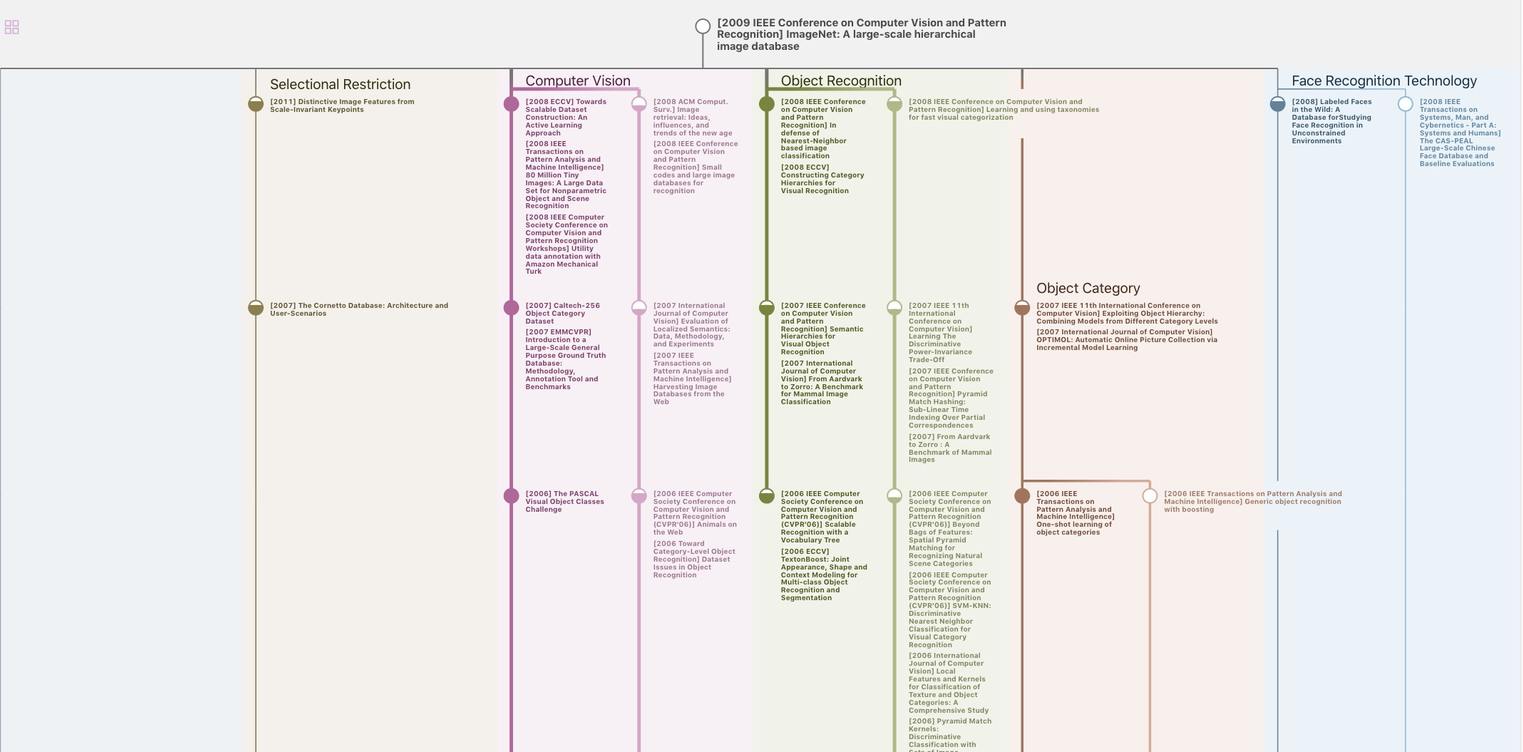
生成溯源树,研究论文发展脉络
Chat Paper
正在生成论文摘要