Prototypes as Explanation for Time Series Anomaly Detection
CoRR(2023)
摘要
Detecting abnormal patterns that deviate from a certain regular repeating pattern in time series is essential in many big data applications. However, the lack of labels, the dynamic nature of time series data, and unforeseeable abnormal behaviors make the detection process challenging. Despite the success of recent deep anomaly detection approaches, the mystical mechanisms in such black-box models have become a new challenge in safety-critical applications. The lack of model transparency and prediction reliability hinders further breakthroughs in such domains. This paper proposes ProtoAD, using prototypes as the example-based explanation for the state of regular patterns during anomaly detection. Without significant impact on the detection performance, prototypes shed light on the deep black-box models and provide intuitive understanding for domain experts and stakeholders. We extend the widely used prototype learning in classification problems into anomaly detection. By visualizing both the latent space and input space prototypes, we intuitively demonstrate how regular data are modeled and why specific patterns are considered abnormal.
更多查看译文
关键词
time series anomaly detection
AI 理解论文
溯源树
样例
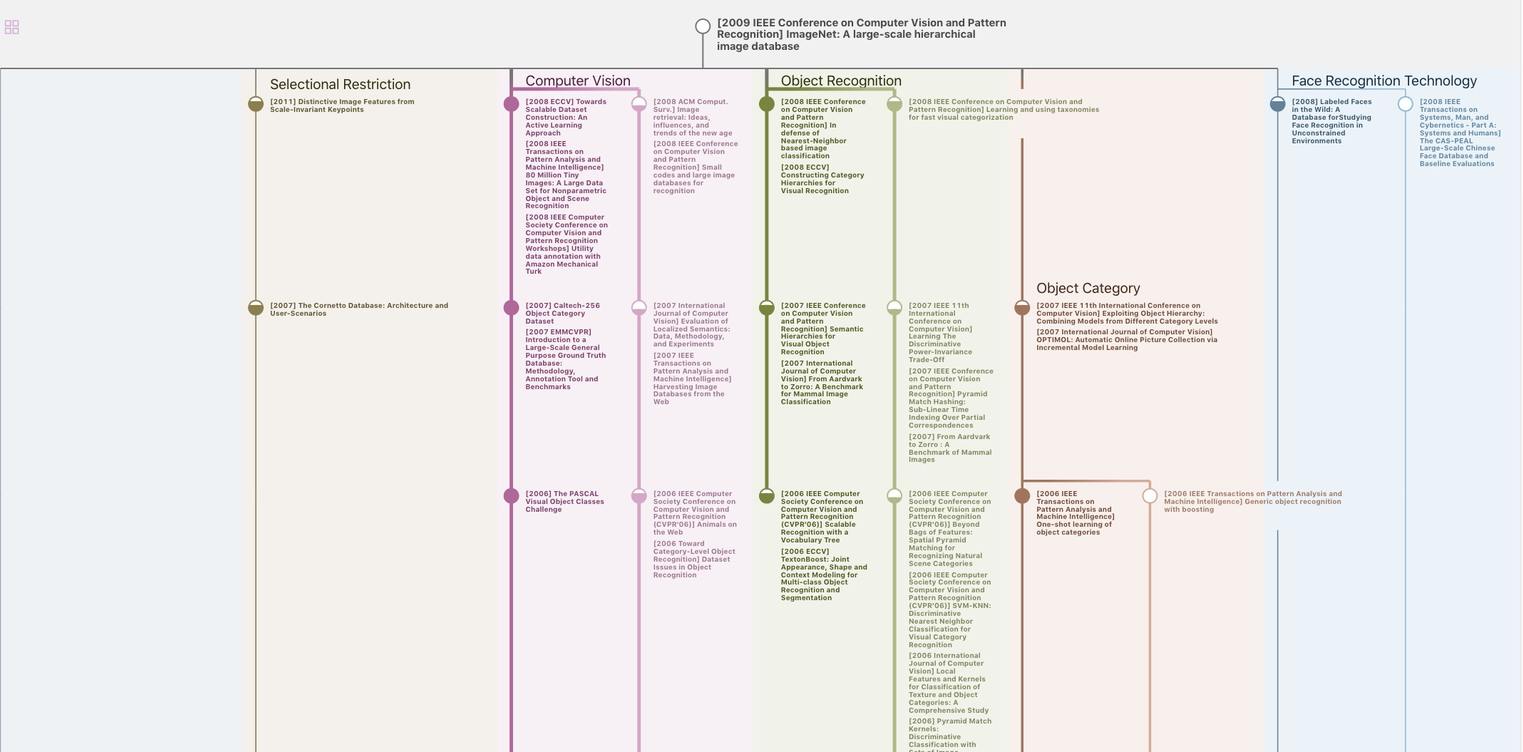
生成溯源树,研究论文发展脉络
Chat Paper
正在生成论文摘要