Shapley Sets: Feature Attribution via Recursive Function Decomposition
CoRR(2023)
摘要
Despite their ubiquitous use, Shapley value feature attributions can be misleading due to feature interaction in both model and data. We propose an alternative attribution approach, Shapley Sets, which awards value to sets of features. Shapley Sets decomposes the underlying model into non-separable variable groups using a recursive function decomposition algorithm with log linear complexity in the number of variables. Shapley Sets attributes to each non-separable variable group their combined value for a particular prediction. We show that Shapley Sets is equivalent to the Shapley value over the transformed feature set and thus benefits from the same axioms of fairness. Shapley Sets is value function agnostic and we show theoretically and experimentally how Shapley Sets avoids pitfalls associated with Shapley value based alternatives and are particularly advantageous for data types with complex dependency structure.
更多查看译文
关键词
feature attribution,decomposition
AI 理解论文
溯源树
样例
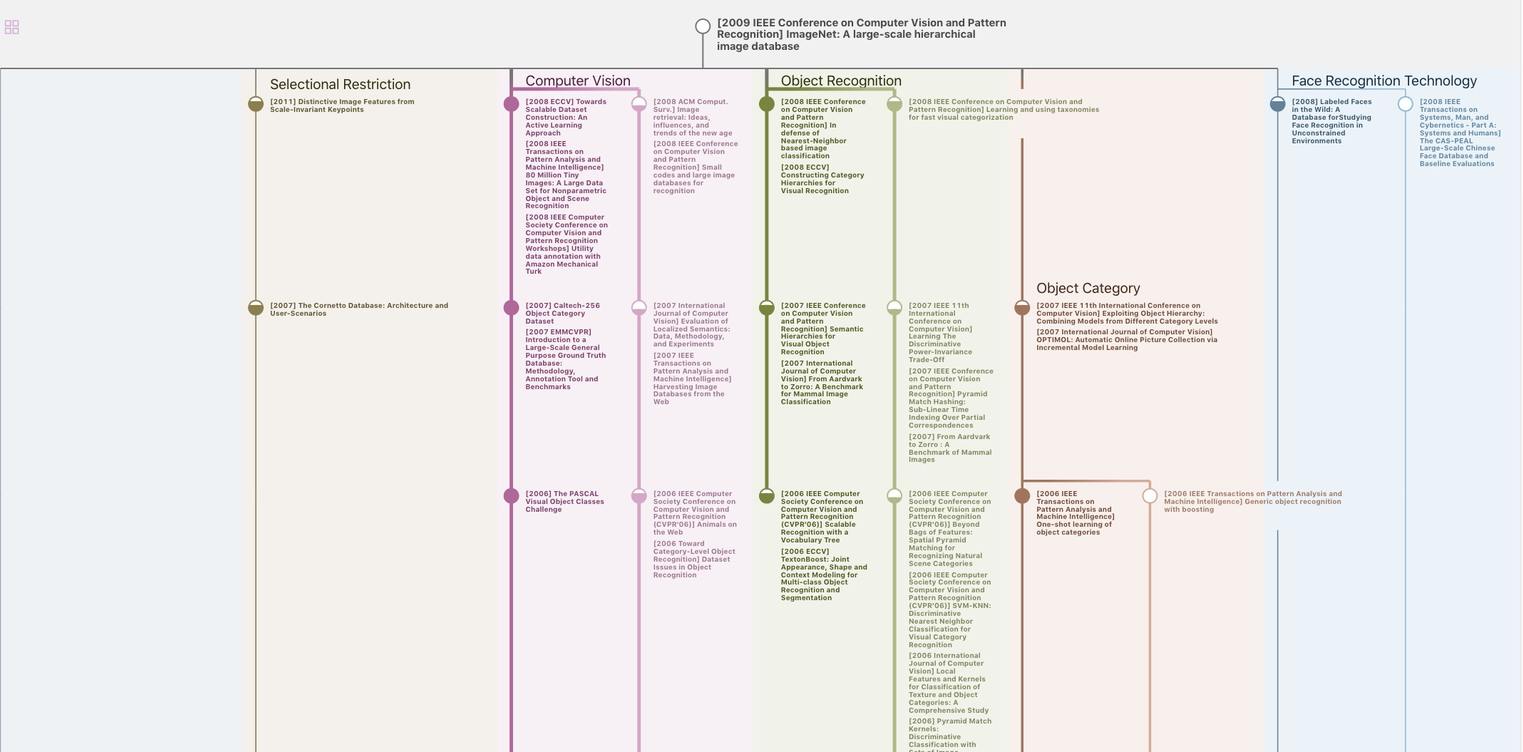
生成溯源树,研究论文发展脉络
Chat Paper
正在生成论文摘要