KDSTM: Neural Semi-supervised Topic Modeling with Knowledge Distillation
CoRR(2023)
摘要
In text classification tasks, fine tuning pretrained language models like
BERT and GPT-3 yields competitive accuracy; however, both methods require
pretraining on large text datasets. In contrast, general topic modeling methods
possess the advantage of analyzing documents to extract meaningful patterns of
words without the need of pretraining. To leverage topic modeling's
unsupervised insights extraction on text classification tasks, we develop the
Knowledge Distillation Semi-supervised Topic Modeling (KDSTM). KDSTM requires
no pretrained embeddings, few labeled documents and is efficient to train,
making it ideal under resource constrained settings. Across a variety of
datasets, our method outperforms existing supervised topic modeling methods in
classification accuracy, robustness and efficiency and achieves similar
performance compare to state of the art weakly supervised text classification
methods.
更多查看译文
关键词
knowledge distillation,topic,neural,semi-supervised
AI 理解论文
溯源树
样例
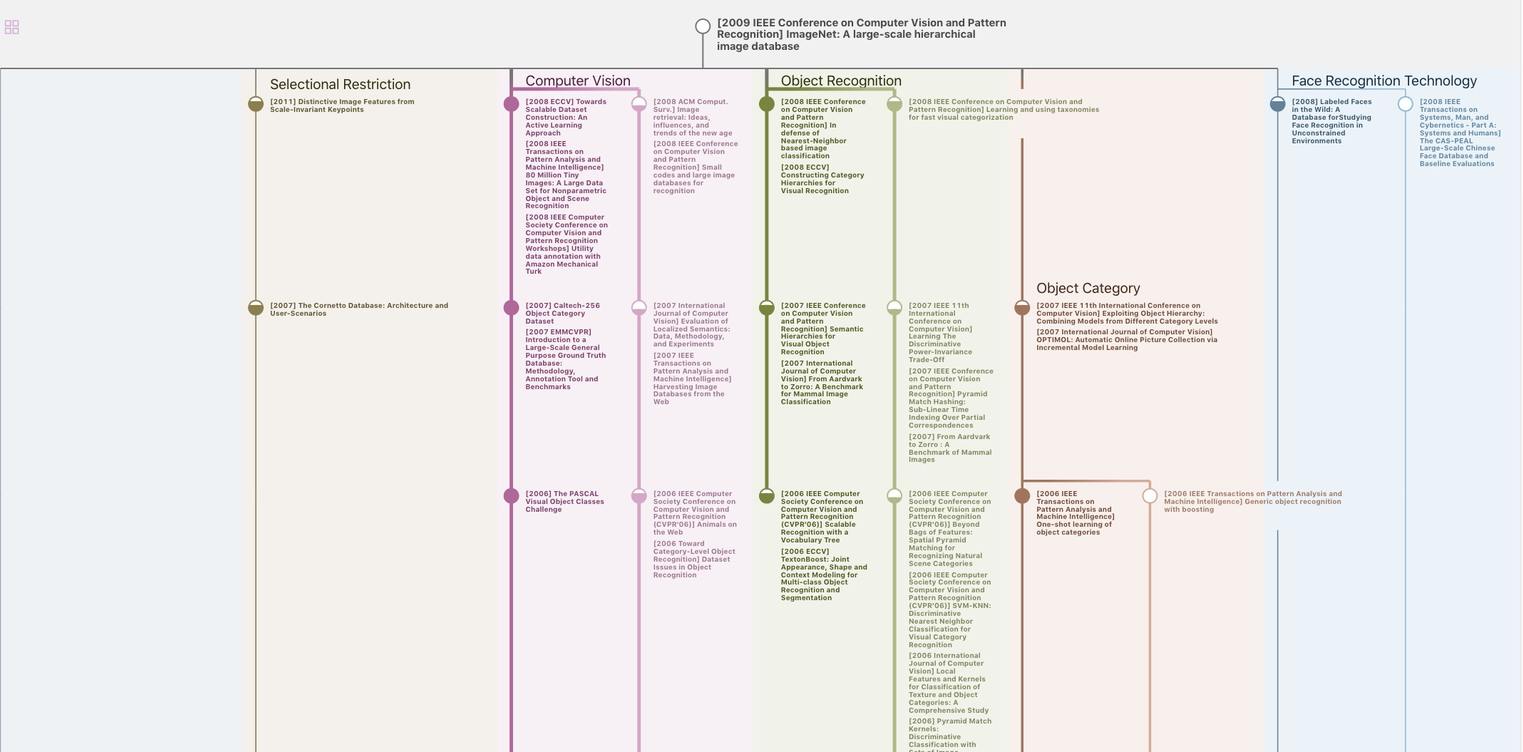
生成溯源树,研究论文发展脉络
Chat Paper
正在生成论文摘要