Unsupervised Spectral Demosaicing With Lightweight Spectral Attention Networks
IEEE TRANSACTIONS ON IMAGE PROCESSING(2024)
摘要
This paper presents a deep learning-based spectral demosaicing technique trained in an unsupervised manner. Many existing deep learning-based techniques relying on supervised learning with synthetic images, often underperform on real-world images, especially as the number of spectral bands increases. This paper presents a comprehensive unsupervised spectral demosaicing (USD) framework based on the characteristics of spectral mosaic images. This framework encompasses a training method, model structure, transformation strategy, and a well-fitted model selection strategy. To enable the network to dynamically model spectral correlation while maintaining a compact parameter space, we reduce the complexity and parameters of the spectral attention module. This is achieved by dividing the spectral attention tensor into spectral attention matrices in the spatial dimension and spectral attention vector in the channel dimension. This paper also presents Mosaic25 , a real 25-band hyperspectral mosaic image dataset featuring various objects, illuminations, and materials for benchmarking purposes. Extensive experiments on both synthetic and real-world datasets demonstrate that the proposed method outperforms conventional unsupervised methods in terms of spatial distortion suppression, spectral fidelity, robustness, and computational cost.
更多查看译文
关键词
Training,Correlation,Cameras,Task analysis,Hyperspectral imaging,Electronics packaging,Distortion,Spectral demosaicing,unsupervised learning,spectral imaging,spectral attention networks
AI 理解论文
溯源树
样例
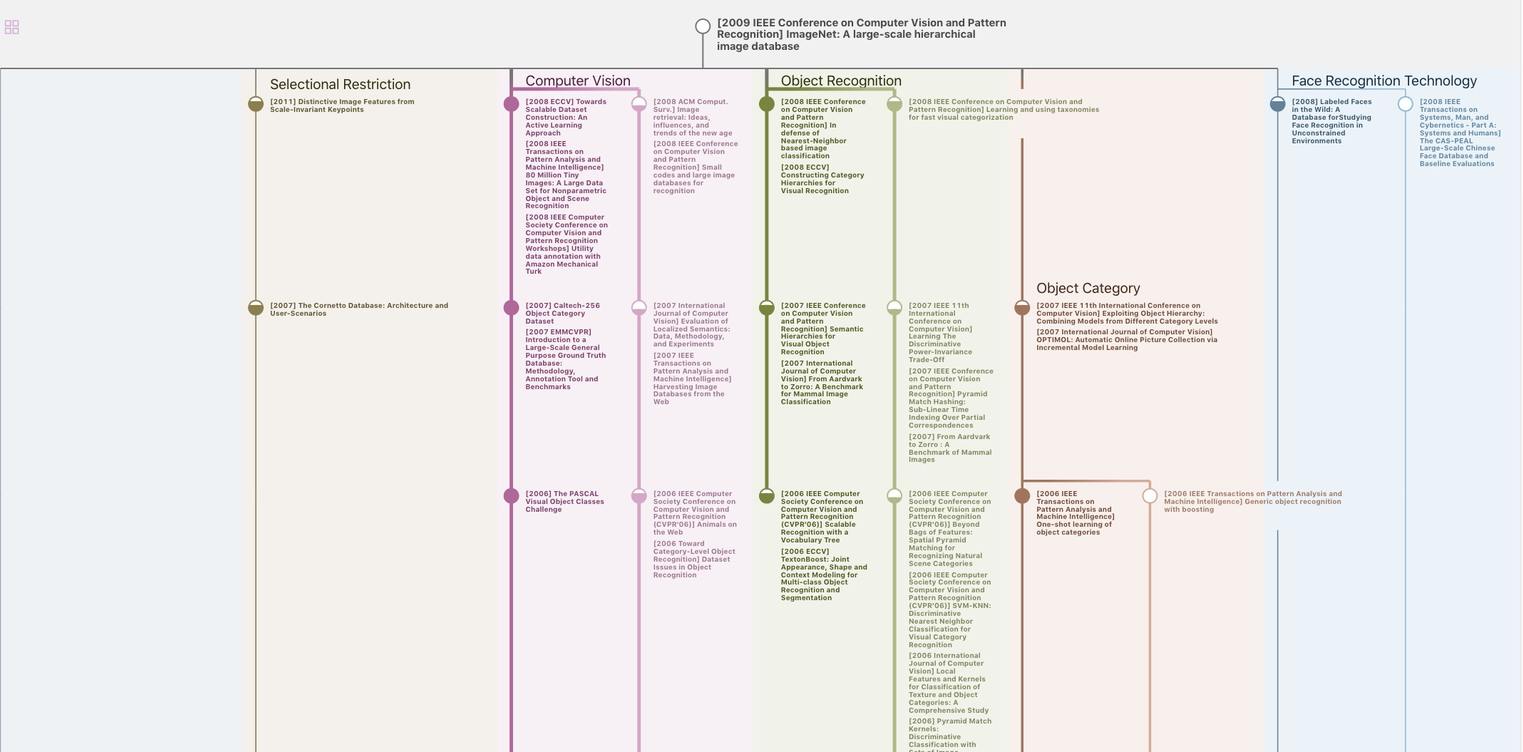
生成溯源树,研究论文发展脉络
Chat Paper
正在生成论文摘要