A Mini-Batch Quasi-Newton Proximal Method for Constrained Total-Variation Nonlinear Image Reconstruction
arXiv (Cornell University)(2023)
摘要
Over the years, computational imaging with accurate nonlinear physical models has drawn considerable interest due to its ability to achieve high-quality reconstructions. However, such nonlinear models are computationally demanding. A popular choice for solving the corresponding inverse problems is accelerated stochastic proximal methods (ASPMs), with the caveat that each iteration is expensive. To overcome this issue, we propose a mini-batch quasi-Newton proximal method (BQNPM) tailored to image-reconstruction problems with total-variation regularization. It involves an efficient approach that computes a weighted proximal mapping at a cost similar to that of the proximal mapping in ASPMs. However, BQNPM requires fewer iterations than ASPMs to converge. We assess the performance of BQNPM on three-dimensional inverse-scattering problems with linear and nonlinear physical models. Our results on simulated and real data show the effectiveness and efficiency of BQNPM,
更多查看译文
关键词
constrained
AI 理解论文
溯源树
样例
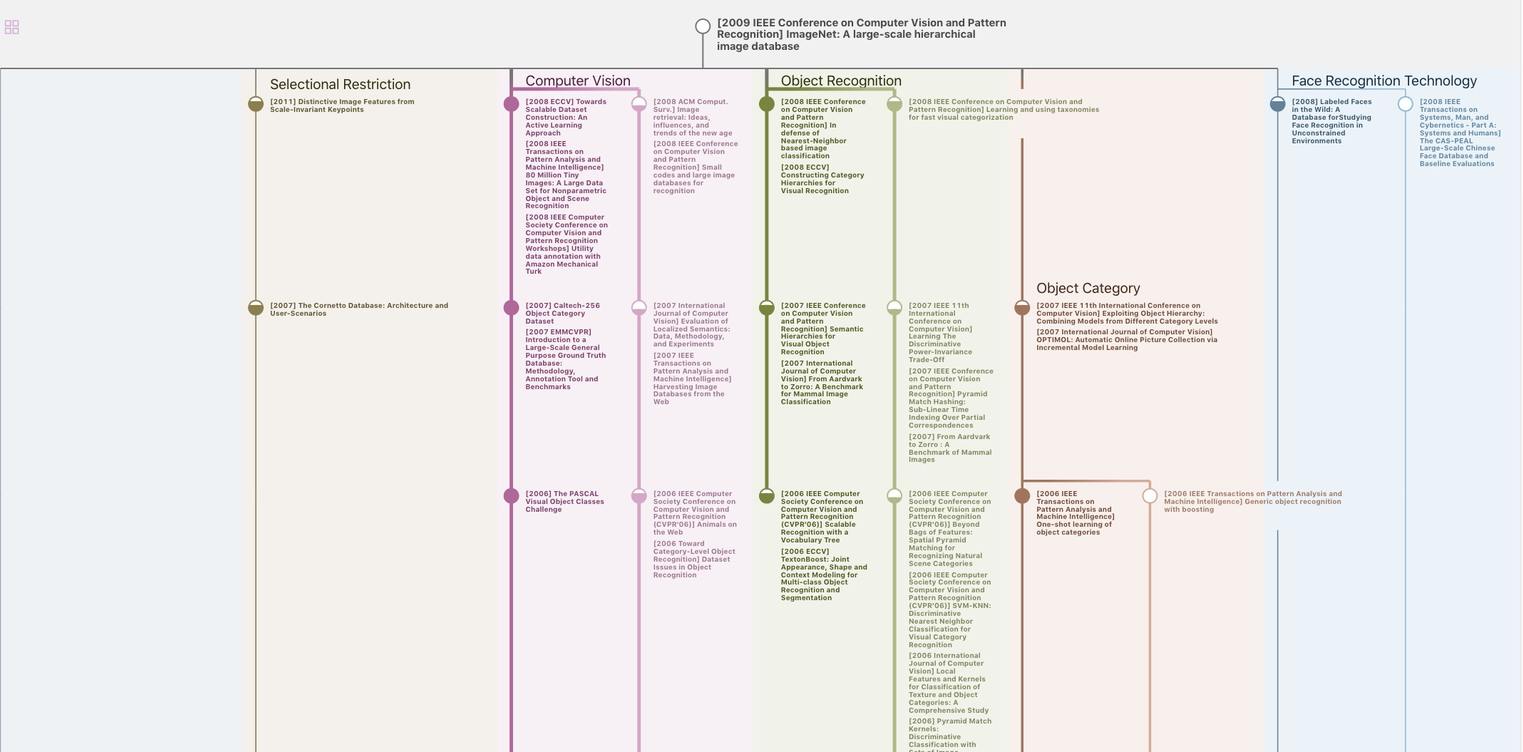
生成溯源树,研究论文发展脉络
Chat Paper
正在生成论文摘要