Convolutional Neural Network Framework for the Automated Analysis of Transition Metal X-ray Photoelectron Spectra
Analytica chimica acta(2023)
摘要
X-ray photoelectron spectroscopy is an indispensable technique for the quantitative determination of sample composition and electronic structure in diverse research fields. Quantitative analysis of the phases present in XP spectra is usually conducted manually by means of empirical peak fitting performed by trained spectroscopists. However, with recent advancements in the usability and reliability of XPS instruments, ever more (inexperienced) users are creating increasingly large data sets that are harder to analyze by hand. In order to aid users with the analysis of large XPS data sets, more automated, easy-to-use analysis techniques are needed. Here, we propose a supervised machine learning framework based on artificial convolutional neural networks. By training such networks on large numbers of artificially created XP spectra with known quantifications (i.e., for each spectrum, the concentration of each chemical species is known), we created universally applicable models for auto-quantification of transition-metal XPS data that are able to predict the sample composition from spectra within seconds. Upon evaluation against more traditional peak fitting methods, we showed that these neural networks achieve competitive quantification accuracy. The proposed framework is shown to be flexible enough to accommodate spectra containing multiple chemical elements and measured with different experimental parameters. The use of dropout variational inference for the determination of quantification uncertainty is illustrated.
更多查看译文
关键词
Electron spectroscopy,Transition metals,Supervised machine learning,Convolutional neural networks,Automated analysis
AI 理解论文
溯源树
样例
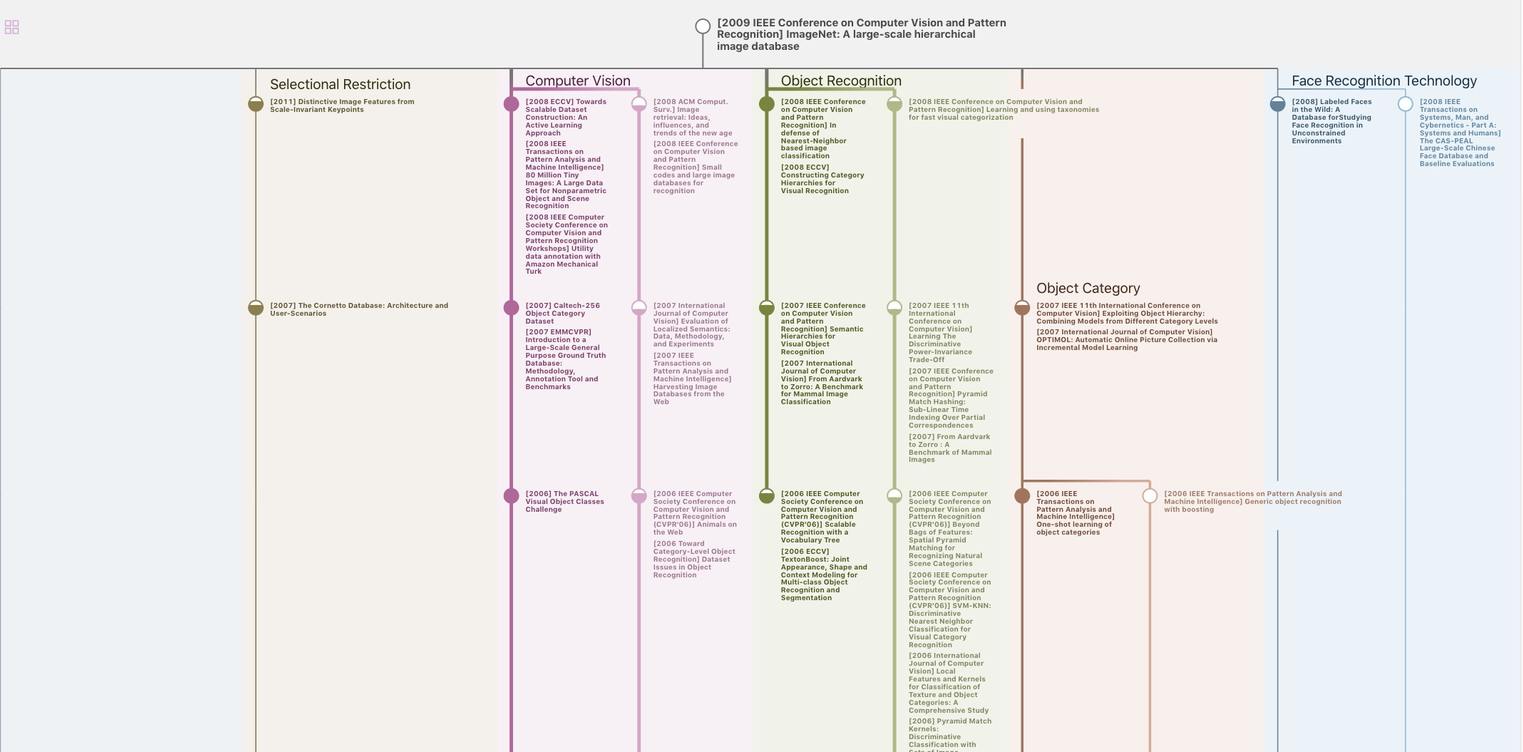
生成溯源树,研究论文发展脉络
Chat Paper
正在生成论文摘要