A Unet-based Research on the Multi-Output Convolution Neural Network's Ability of Decreasing Mis-identification: Automatic Segmentation of Organs at Risk in Thorax
Research Square (Research Square)(2020)
摘要
Abstract Background To study a multi-output convolution neural network (CNN)’s capability of reducing mis-identification. Material and Methods To guarantee that the CNN’s output number was the only experiment variable, we used Unet as research object. By modifying it into a multi-output (MO) one, we got a MO-Unet and the conventional single-output Unet (SO-Uent) as a comparing object. All images involved in this study were computed tomography (CT) scans coming from 105 patients with thoracic tumor. 3 organs at risk (OARs), i.e. lung, heart and spinal cord, were delineated by experienced radiation oncologists and were used as ground truth. The two models were both trained with 1240 CTs (856 images for learning and 384 images for monitor) and under the same learning settings. They were both tested on other 886 images. Dice and mis-identification pixels’ number(n) were 2 metrics for evaluation. Results MO-Unet and SO-Unet achieved Dice of 0.9400 ± 0.0612 (average ± standard deviation) and 0.9451 ± 0.0618 for lung, 0.9143 ± 0.1119 and 0.9160 ± 0.1071 for heart, 0.8988 ± 0.0657 and 0.9020 ± 0.0624 for spinal cord respectively. The two models’ all average Dices were ≤ 0.005. For the normalized number of cases with n = 0, MO-Unet and SO-Unet had 97.29% and 96.84% for spinal cord, 88.49% and 90.86% for heart, 81.26% and 77.09% for lung respectively. Compared to SO-Unet, the mis-identification cases of MO-Unet mainly felled in the range of small n. Conclusions The Dice results showed that the two models had comparable overlap. The n results suggested that the MO-Unet was better in decreasing mis-identification. Besides, a MO network is light-weighted to implement more delineation under the same computing source. Therefore, a MO network is promising in segmenting OARs and has the potential for a widespread application in China.
更多查看译文
关键词
automatic segmentation,neural network,organs,unet-based,multi-output,mis-identification
AI 理解论文
溯源树
样例
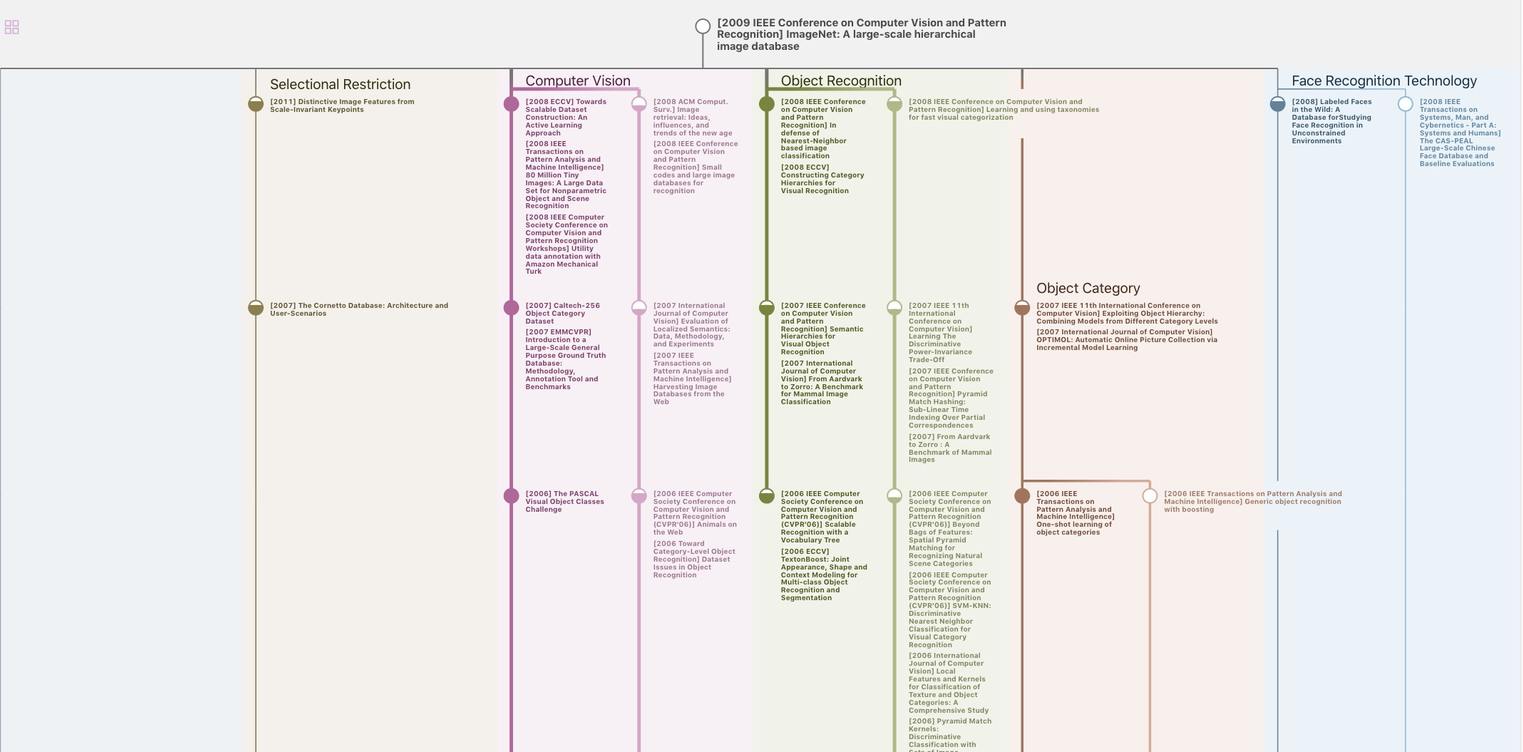
生成溯源树,研究论文发展脉络
Chat Paper
正在生成论文摘要